Can we always find a stochastic process whose autocorrelation function is a given positive semidefinite...
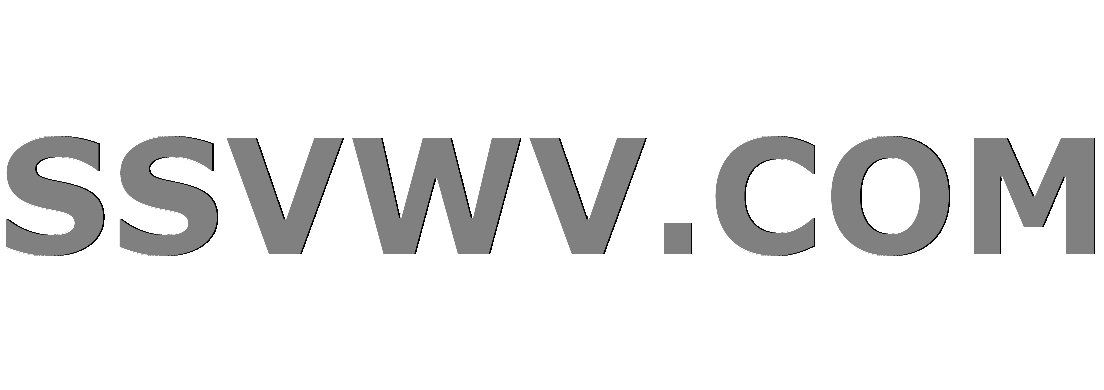
Multi tool use
up vote
1
down vote
favorite
The auto-correlation function of a stochastic process is defined by $$R(t_1, t_2)=mathbb{E}[X(t_1)X(t_2)]$$
We say that a function is positive semidefinite if and only if
$$forall x,y: f(x,y)^2 leq f(x,x)f(y,y)$$
It is easy to see that if $X(t)$ is a stochastic process, then using the Cauchy-Schwartz inequality we have
$$R(t_1, t_2)^2=mathbb{E}[X(t_1)X(t_2)]^2 leq mathbb{E}[X(t_1)^2]mathbb{E}[X(t_2)^2]=R(t_1, t_1)R(t_2, t_2)$$
So, I wonder if one is given a positive semidefinite function $f$, is it always possible to find a stochastic process whose auto-correlation function is $f$?
Note: I'm looking for answers that do not use measure theory.
probability-theory proof-verification stochastic-processes signal-processing
add a comment |
up vote
1
down vote
favorite
The auto-correlation function of a stochastic process is defined by $$R(t_1, t_2)=mathbb{E}[X(t_1)X(t_2)]$$
We say that a function is positive semidefinite if and only if
$$forall x,y: f(x,y)^2 leq f(x,x)f(y,y)$$
It is easy to see that if $X(t)$ is a stochastic process, then using the Cauchy-Schwartz inequality we have
$$R(t_1, t_2)^2=mathbb{E}[X(t_1)X(t_2)]^2 leq mathbb{E}[X(t_1)^2]mathbb{E}[X(t_2)^2]=R(t_1, t_1)R(t_2, t_2)$$
So, I wonder if one is given a positive semidefinite function $f$, is it always possible to find a stochastic process whose auto-correlation function is $f$?
Note: I'm looking for answers that do not use measure theory.
probability-theory proof-verification stochastic-processes signal-processing
By Kolmogorov's consistency theorem, yes.
– Calculon
Nov 8 at 9:58
Yes, they are referring to the same theorem. The Wikipedia page is talking about continuous time processes. You should read it a bit more carefully. I can write more later if no one else responds.
– Calculon
Nov 8 at 10:09
@Calculon Oops, you're right. Is it possible to do this without measure theory? The question has been brought up as a bonus question in an undergrad course. And we're allowed to research it on the internet. But I think we're not allowed to use measure theory as it is out of the scope of the course.
– stressed out
Nov 8 at 10:11
How do you define a stochastic process without using measure theory?
– Mike Hawk
Nov 13 at 20:47
@MikeHawk A family of random variables parametrized by time. It's not 100% rigorous, but it convinces engineers. Doesn't it?
– stressed out
Nov 13 at 21:38
add a comment |
up vote
1
down vote
favorite
up vote
1
down vote
favorite
The auto-correlation function of a stochastic process is defined by $$R(t_1, t_2)=mathbb{E}[X(t_1)X(t_2)]$$
We say that a function is positive semidefinite if and only if
$$forall x,y: f(x,y)^2 leq f(x,x)f(y,y)$$
It is easy to see that if $X(t)$ is a stochastic process, then using the Cauchy-Schwartz inequality we have
$$R(t_1, t_2)^2=mathbb{E}[X(t_1)X(t_2)]^2 leq mathbb{E}[X(t_1)^2]mathbb{E}[X(t_2)^2]=R(t_1, t_1)R(t_2, t_2)$$
So, I wonder if one is given a positive semidefinite function $f$, is it always possible to find a stochastic process whose auto-correlation function is $f$?
Note: I'm looking for answers that do not use measure theory.
probability-theory proof-verification stochastic-processes signal-processing
The auto-correlation function of a stochastic process is defined by $$R(t_1, t_2)=mathbb{E}[X(t_1)X(t_2)]$$
We say that a function is positive semidefinite if and only if
$$forall x,y: f(x,y)^2 leq f(x,x)f(y,y)$$
It is easy to see that if $X(t)$ is a stochastic process, then using the Cauchy-Schwartz inequality we have
$$R(t_1, t_2)^2=mathbb{E}[X(t_1)X(t_2)]^2 leq mathbb{E}[X(t_1)^2]mathbb{E}[X(t_2)^2]=R(t_1, t_1)R(t_2, t_2)$$
So, I wonder if one is given a positive semidefinite function $f$, is it always possible to find a stochastic process whose auto-correlation function is $f$?
Note: I'm looking for answers that do not use measure theory.
probability-theory proof-verification stochastic-processes signal-processing
probability-theory proof-verification stochastic-processes signal-processing
edited Nov 8 at 10:16
asked Nov 7 at 11:01
stressed out
3,7381532
3,7381532
By Kolmogorov's consistency theorem, yes.
– Calculon
Nov 8 at 9:58
Yes, they are referring to the same theorem. The Wikipedia page is talking about continuous time processes. You should read it a bit more carefully. I can write more later if no one else responds.
– Calculon
Nov 8 at 10:09
@Calculon Oops, you're right. Is it possible to do this without measure theory? The question has been brought up as a bonus question in an undergrad course. And we're allowed to research it on the internet. But I think we're not allowed to use measure theory as it is out of the scope of the course.
– stressed out
Nov 8 at 10:11
How do you define a stochastic process without using measure theory?
– Mike Hawk
Nov 13 at 20:47
@MikeHawk A family of random variables parametrized by time. It's not 100% rigorous, but it convinces engineers. Doesn't it?
– stressed out
Nov 13 at 21:38
add a comment |
By Kolmogorov's consistency theorem, yes.
– Calculon
Nov 8 at 9:58
Yes, they are referring to the same theorem. The Wikipedia page is talking about continuous time processes. You should read it a bit more carefully. I can write more later if no one else responds.
– Calculon
Nov 8 at 10:09
@Calculon Oops, you're right. Is it possible to do this without measure theory? The question has been brought up as a bonus question in an undergrad course. And we're allowed to research it on the internet. But I think we're not allowed to use measure theory as it is out of the scope of the course.
– stressed out
Nov 8 at 10:11
How do you define a stochastic process without using measure theory?
– Mike Hawk
Nov 13 at 20:47
@MikeHawk A family of random variables parametrized by time. It's not 100% rigorous, but it convinces engineers. Doesn't it?
– stressed out
Nov 13 at 21:38
By Kolmogorov's consistency theorem, yes.
– Calculon
Nov 8 at 9:58
By Kolmogorov's consistency theorem, yes.
– Calculon
Nov 8 at 9:58
Yes, they are referring to the same theorem. The Wikipedia page is talking about continuous time processes. You should read it a bit more carefully. I can write more later if no one else responds.
– Calculon
Nov 8 at 10:09
Yes, they are referring to the same theorem. The Wikipedia page is talking about continuous time processes. You should read it a bit more carefully. I can write more later if no one else responds.
– Calculon
Nov 8 at 10:09
@Calculon Oops, you're right. Is it possible to do this without measure theory? The question has been brought up as a bonus question in an undergrad course. And we're allowed to research it on the internet. But I think we're not allowed to use measure theory as it is out of the scope of the course.
– stressed out
Nov 8 at 10:11
@Calculon Oops, you're right. Is it possible to do this without measure theory? The question has been brought up as a bonus question in an undergrad course. And we're allowed to research it on the internet. But I think we're not allowed to use measure theory as it is out of the scope of the course.
– stressed out
Nov 8 at 10:11
How do you define a stochastic process without using measure theory?
– Mike Hawk
Nov 13 at 20:47
How do you define a stochastic process without using measure theory?
– Mike Hawk
Nov 13 at 20:47
@MikeHawk A family of random variables parametrized by time. It's not 100% rigorous, but it convinces engineers. Doesn't it?
– stressed out
Nov 13 at 21:38
@MikeHawk A family of random variables parametrized by time. It's not 100% rigorous, but it convinces engineers. Doesn't it?
– stressed out
Nov 13 at 21:38
add a comment |
1 Answer
1
active
oldest
votes
up vote
1
down vote
accepted
Obviously not. Consider $R(x,y)=-1$. But I'll assume you also meant to require that $R(x,x)ge 0$. However, even this is not sufficient.
To see this, pick any 3 time points $t_1, t_2, t_3$ and consider the random variables $X_{t_1}, X_{t_2}, X_{t_3}$. Define the matrix $A_{ij}=E(X_{t_i}X_{t_j})=R(t_i, t_j)$. I claim $A$ must be non-negative definite. Indeed, for any vector $v$, $(v,Av)=sum_{i,j}v_iv_jA_{ij}=sum_{i,j}v_iv_jE(X_{t_i}X_{t_j})=Esum_{i,j}(v_iX_{t_i})(v_jX_{t_j})=E(sum_i v_iX_{t_i})^2ge 0$. By the same argument, it must be the case that $sum_{i,j=1}^Nv_iv_jR(t_i,t_j)ge 0$ for any $N$, $v_i$ and time points $t_i$. A function which satisfies this condition is called a Mercer kernel.
To see that your condition of positive-semidefiniteness does not imply being a Mercer kernel, it is sufficient to exhibit a symmetric matrix M such that:
1. the diagonal entries of M are positive
2. all 2x2 minors have non-negative determinant, and
3. M has a negative eigenvalue. I leave it as an exercise to construct such a matrix.
Interestingly, if R is a Mercer kernel, then the answer to your question is yes, and the process can be described somewhat explicitly. I'll assume that t is restricted to lie in the unit interval $[0,1]$. By Mercer's theorem, we can write $R(x,y)=sum_{i=1}^{infty} lambda_i f_i(x)f_i(y)$, where the functions satisfy $int_0^1 R(x,y)f_i(y)=lambda_if_i(x)$.
Now let $X_t=sum_{i=1}^{infty} sqrt{lambda_i}xi_if_i(t)$ where $xi_i$ are iid normal random variables with mean $0$ and standard deviation $1$. The series converges almost surely by Kolmogorov's Two SEries theorem.
We see that $EX_tX_s=sum_{i,j}sqrt{lambda_ilambda_j}f_i(t)f_j(s)E(xi_ixi_j)$. By independence, we have $E(xi_ixi_j)=Exi_iExi_j=delta_{ij}$. So $EX_tX_s=sum_ilambda_if_i(t)f_i(s)=R(t,s)$ as desired.
because $E(X_{t}^2)=R(t,t)$ cannot be negative
– Mike Hawk
Nov 14 at 14:27
add a comment |
1 Answer
1
active
oldest
votes
1 Answer
1
active
oldest
votes
active
oldest
votes
active
oldest
votes
up vote
1
down vote
accepted
Obviously not. Consider $R(x,y)=-1$. But I'll assume you also meant to require that $R(x,x)ge 0$. However, even this is not sufficient.
To see this, pick any 3 time points $t_1, t_2, t_3$ and consider the random variables $X_{t_1}, X_{t_2}, X_{t_3}$. Define the matrix $A_{ij}=E(X_{t_i}X_{t_j})=R(t_i, t_j)$. I claim $A$ must be non-negative definite. Indeed, for any vector $v$, $(v,Av)=sum_{i,j}v_iv_jA_{ij}=sum_{i,j}v_iv_jE(X_{t_i}X_{t_j})=Esum_{i,j}(v_iX_{t_i})(v_jX_{t_j})=E(sum_i v_iX_{t_i})^2ge 0$. By the same argument, it must be the case that $sum_{i,j=1}^Nv_iv_jR(t_i,t_j)ge 0$ for any $N$, $v_i$ and time points $t_i$. A function which satisfies this condition is called a Mercer kernel.
To see that your condition of positive-semidefiniteness does not imply being a Mercer kernel, it is sufficient to exhibit a symmetric matrix M such that:
1. the diagonal entries of M are positive
2. all 2x2 minors have non-negative determinant, and
3. M has a negative eigenvalue. I leave it as an exercise to construct such a matrix.
Interestingly, if R is a Mercer kernel, then the answer to your question is yes, and the process can be described somewhat explicitly. I'll assume that t is restricted to lie in the unit interval $[0,1]$. By Mercer's theorem, we can write $R(x,y)=sum_{i=1}^{infty} lambda_i f_i(x)f_i(y)$, where the functions satisfy $int_0^1 R(x,y)f_i(y)=lambda_if_i(x)$.
Now let $X_t=sum_{i=1}^{infty} sqrt{lambda_i}xi_if_i(t)$ where $xi_i$ are iid normal random variables with mean $0$ and standard deviation $1$. The series converges almost surely by Kolmogorov's Two SEries theorem.
We see that $EX_tX_s=sum_{i,j}sqrt{lambda_ilambda_j}f_i(t)f_j(s)E(xi_ixi_j)$. By independence, we have $E(xi_ixi_j)=Exi_iExi_j=delta_{ij}$. So $EX_tX_s=sum_ilambda_if_i(t)f_i(s)=R(t,s)$ as desired.
because $E(X_{t}^2)=R(t,t)$ cannot be negative
– Mike Hawk
Nov 14 at 14:27
add a comment |
up vote
1
down vote
accepted
Obviously not. Consider $R(x,y)=-1$. But I'll assume you also meant to require that $R(x,x)ge 0$. However, even this is not sufficient.
To see this, pick any 3 time points $t_1, t_2, t_3$ and consider the random variables $X_{t_1}, X_{t_2}, X_{t_3}$. Define the matrix $A_{ij}=E(X_{t_i}X_{t_j})=R(t_i, t_j)$. I claim $A$ must be non-negative definite. Indeed, for any vector $v$, $(v,Av)=sum_{i,j}v_iv_jA_{ij}=sum_{i,j}v_iv_jE(X_{t_i}X_{t_j})=Esum_{i,j}(v_iX_{t_i})(v_jX_{t_j})=E(sum_i v_iX_{t_i})^2ge 0$. By the same argument, it must be the case that $sum_{i,j=1}^Nv_iv_jR(t_i,t_j)ge 0$ for any $N$, $v_i$ and time points $t_i$. A function which satisfies this condition is called a Mercer kernel.
To see that your condition of positive-semidefiniteness does not imply being a Mercer kernel, it is sufficient to exhibit a symmetric matrix M such that:
1. the diagonal entries of M are positive
2. all 2x2 minors have non-negative determinant, and
3. M has a negative eigenvalue. I leave it as an exercise to construct such a matrix.
Interestingly, if R is a Mercer kernel, then the answer to your question is yes, and the process can be described somewhat explicitly. I'll assume that t is restricted to lie in the unit interval $[0,1]$. By Mercer's theorem, we can write $R(x,y)=sum_{i=1}^{infty} lambda_i f_i(x)f_i(y)$, where the functions satisfy $int_0^1 R(x,y)f_i(y)=lambda_if_i(x)$.
Now let $X_t=sum_{i=1}^{infty} sqrt{lambda_i}xi_if_i(t)$ where $xi_i$ are iid normal random variables with mean $0$ and standard deviation $1$. The series converges almost surely by Kolmogorov's Two SEries theorem.
We see that $EX_tX_s=sum_{i,j}sqrt{lambda_ilambda_j}f_i(t)f_j(s)E(xi_ixi_j)$. By independence, we have $E(xi_ixi_j)=Exi_iExi_j=delta_{ij}$. So $EX_tX_s=sum_ilambda_if_i(t)f_i(s)=R(t,s)$ as desired.
because $E(X_{t}^2)=R(t,t)$ cannot be negative
– Mike Hawk
Nov 14 at 14:27
add a comment |
up vote
1
down vote
accepted
up vote
1
down vote
accepted
Obviously not. Consider $R(x,y)=-1$. But I'll assume you also meant to require that $R(x,x)ge 0$. However, even this is not sufficient.
To see this, pick any 3 time points $t_1, t_2, t_3$ and consider the random variables $X_{t_1}, X_{t_2}, X_{t_3}$. Define the matrix $A_{ij}=E(X_{t_i}X_{t_j})=R(t_i, t_j)$. I claim $A$ must be non-negative definite. Indeed, for any vector $v$, $(v,Av)=sum_{i,j}v_iv_jA_{ij}=sum_{i,j}v_iv_jE(X_{t_i}X_{t_j})=Esum_{i,j}(v_iX_{t_i})(v_jX_{t_j})=E(sum_i v_iX_{t_i})^2ge 0$. By the same argument, it must be the case that $sum_{i,j=1}^Nv_iv_jR(t_i,t_j)ge 0$ for any $N$, $v_i$ and time points $t_i$. A function which satisfies this condition is called a Mercer kernel.
To see that your condition of positive-semidefiniteness does not imply being a Mercer kernel, it is sufficient to exhibit a symmetric matrix M such that:
1. the diagonal entries of M are positive
2. all 2x2 minors have non-negative determinant, and
3. M has a negative eigenvalue. I leave it as an exercise to construct such a matrix.
Interestingly, if R is a Mercer kernel, then the answer to your question is yes, and the process can be described somewhat explicitly. I'll assume that t is restricted to lie in the unit interval $[0,1]$. By Mercer's theorem, we can write $R(x,y)=sum_{i=1}^{infty} lambda_i f_i(x)f_i(y)$, where the functions satisfy $int_0^1 R(x,y)f_i(y)=lambda_if_i(x)$.
Now let $X_t=sum_{i=1}^{infty} sqrt{lambda_i}xi_if_i(t)$ where $xi_i$ are iid normal random variables with mean $0$ and standard deviation $1$. The series converges almost surely by Kolmogorov's Two SEries theorem.
We see that $EX_tX_s=sum_{i,j}sqrt{lambda_ilambda_j}f_i(t)f_j(s)E(xi_ixi_j)$. By independence, we have $E(xi_ixi_j)=Exi_iExi_j=delta_{ij}$. So $EX_tX_s=sum_ilambda_if_i(t)f_i(s)=R(t,s)$ as desired.
Obviously not. Consider $R(x,y)=-1$. But I'll assume you also meant to require that $R(x,x)ge 0$. However, even this is not sufficient.
To see this, pick any 3 time points $t_1, t_2, t_3$ and consider the random variables $X_{t_1}, X_{t_2}, X_{t_3}$. Define the matrix $A_{ij}=E(X_{t_i}X_{t_j})=R(t_i, t_j)$. I claim $A$ must be non-negative definite. Indeed, for any vector $v$, $(v,Av)=sum_{i,j}v_iv_jA_{ij}=sum_{i,j}v_iv_jE(X_{t_i}X_{t_j})=Esum_{i,j}(v_iX_{t_i})(v_jX_{t_j})=E(sum_i v_iX_{t_i})^2ge 0$. By the same argument, it must be the case that $sum_{i,j=1}^Nv_iv_jR(t_i,t_j)ge 0$ for any $N$, $v_i$ and time points $t_i$. A function which satisfies this condition is called a Mercer kernel.
To see that your condition of positive-semidefiniteness does not imply being a Mercer kernel, it is sufficient to exhibit a symmetric matrix M such that:
1. the diagonal entries of M are positive
2. all 2x2 minors have non-negative determinant, and
3. M has a negative eigenvalue. I leave it as an exercise to construct such a matrix.
Interestingly, if R is a Mercer kernel, then the answer to your question is yes, and the process can be described somewhat explicitly. I'll assume that t is restricted to lie in the unit interval $[0,1]$. By Mercer's theorem, we can write $R(x,y)=sum_{i=1}^{infty} lambda_i f_i(x)f_i(y)$, where the functions satisfy $int_0^1 R(x,y)f_i(y)=lambda_if_i(x)$.
Now let $X_t=sum_{i=1}^{infty} sqrt{lambda_i}xi_if_i(t)$ where $xi_i$ are iid normal random variables with mean $0$ and standard deviation $1$. The series converges almost surely by Kolmogorov's Two SEries theorem.
We see that $EX_tX_s=sum_{i,j}sqrt{lambda_ilambda_j}f_i(t)f_j(s)E(xi_ixi_j)$. By independence, we have $E(xi_ixi_j)=Exi_iExi_j=delta_{ij}$. So $EX_tX_s=sum_ilambda_if_i(t)f_i(s)=R(t,s)$ as desired.
answered Nov 13 at 23:54
Mike Hawk
1,312110
1,312110
because $E(X_{t}^2)=R(t,t)$ cannot be negative
– Mike Hawk
Nov 14 at 14:27
add a comment |
because $E(X_{t}^2)=R(t,t)$ cannot be negative
– Mike Hawk
Nov 14 at 14:27
because $E(X_{t}^2)=R(t,t)$ cannot be negative
– Mike Hawk
Nov 14 at 14:27
because $E(X_{t}^2)=R(t,t)$ cannot be negative
– Mike Hawk
Nov 14 at 14:27
add a comment |
Sign up or log in
StackExchange.ready(function () {
StackExchange.helpers.onClickDraftSave('#login-link');
});
Sign up using Google
Sign up using Facebook
Sign up using Email and Password
Post as a guest
Required, but never shown
StackExchange.ready(
function () {
StackExchange.openid.initPostLogin('.new-post-login', 'https%3a%2f%2fmath.stackexchange.com%2fquestions%2f2988393%2fcan-we-always-find-a-stochastic-process-whose-autocorrelation-function-is-a-give%23new-answer', 'question_page');
}
);
Post as a guest
Required, but never shown
Sign up or log in
StackExchange.ready(function () {
StackExchange.helpers.onClickDraftSave('#login-link');
});
Sign up using Google
Sign up using Facebook
Sign up using Email and Password
Post as a guest
Required, but never shown
Sign up or log in
StackExchange.ready(function () {
StackExchange.helpers.onClickDraftSave('#login-link');
});
Sign up using Google
Sign up using Facebook
Sign up using Email and Password
Post as a guest
Required, but never shown
Sign up or log in
StackExchange.ready(function () {
StackExchange.helpers.onClickDraftSave('#login-link');
});
Sign up using Google
Sign up using Facebook
Sign up using Email and Password
Sign up using Google
Sign up using Facebook
Sign up using Email and Password
Post as a guest
Required, but never shown
Required, but never shown
Required, but never shown
Required, but never shown
Required, but never shown
Required, but never shown
Required, but never shown
Required, but never shown
Required, but never shown
neAZzMyn6
By Kolmogorov's consistency theorem, yes.
– Calculon
Nov 8 at 9:58
Yes, they are referring to the same theorem. The Wikipedia page is talking about continuous time processes. You should read it a bit more carefully. I can write more later if no one else responds.
– Calculon
Nov 8 at 10:09
@Calculon Oops, you're right. Is it possible to do this without measure theory? The question has been brought up as a bonus question in an undergrad course. And we're allowed to research it on the internet. But I think we're not allowed to use measure theory as it is out of the scope of the course.
– stressed out
Nov 8 at 10:11
How do you define a stochastic process without using measure theory?
– Mike Hawk
Nov 13 at 20:47
@MikeHawk A family of random variables parametrized by time. It's not 100% rigorous, but it convinces engineers. Doesn't it?
– stressed out
Nov 13 at 21:38