Processing time series with the Tensorflow Dataset API for feeding an LSTM in batches
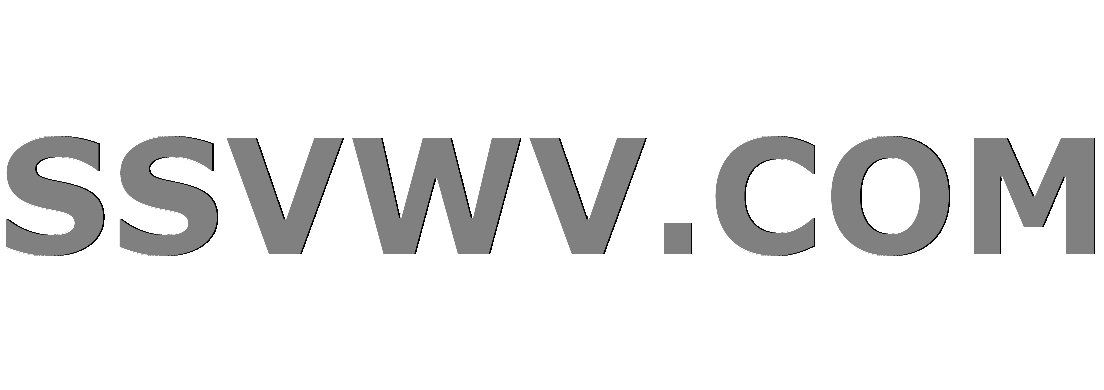
Multi tool use
I have a working solution for my problem, but I find it relatively slow, and I would be surprised if there is no better way of doing what I want. See the description of the problem below.
CURRENT SOLUTION: (comes first, as it might be simpler for experienced users of tensorflow to infer what I want to do from this code snippet)
def stack_batch_dim(*x):
return {key: tf.stack([b[key] for b in x], axis=1) for key in x[0]}
dataset = tf.data.TFRecordDataset(filenames)
dataset = dataset.map(map_func=parse_box2d_protobuf, num_parallel_calls=8)
dataset = dataset.repeat()
# place the "cursors":
datasets = [dataset.skip(t * inter_cursor_space) for t in range(batch_size)]
dataset = tf.data.Dataset.zip(tuple(datasets))
# consume time_depth samples at a time
dataset = dataset.batch(time_depth)
# the actual batch dimension is a tuple and not a tensor
# need to "stack" the tuple into a tensor
dataset = dataset.map(stack_batch_dim)
iterator = dataset.make_initializable_iterator()
So I would like train an LSTM network with a sequence of data points. The data I have is stored in tfrecords, (the recommended way of storing data with tensorflow).
dataset = tf.data.TFRecordDataset(filenames)
dataset = dataset.map(map_func=parse_box2d_protobuf, num_parallel_calls=8)
# parse_box2d_protobuf is a function that transforms raw bytes into
# a python structure of the form:
# {"positions": tensor_of_shape_4,
# "speeds": tensor_of_shape_4,
# "action": tensor_of_shape_4,
# "vision": tensor_of_shape_h_w_3,
# "index": tensor_int64}
iterator = dataset.make_initializable_iterator()
next_sample = iterator.get_next()
with tf.Session() as sess:
sess.run(iterator.initializer)
print(sess.run(next_sample))
# prints a dictionary as expected.
Now what I want to do is to read that sequence in order to feed it in an LSTM in batches.
As you might expect, the "index" key in the dictionary corresponds to an integer that is the index of the data sample in the sequence (starting from 0):
with tf.Session() as sess:
sess.run(iterator.initializer)
for i in range(10, 15):
print(sess.run(next_sample["index"]))
# prints successively 0, 1, 2, 3 and 4
Now let's say I want a batch size of 4, and I want to unfold the LSTM net for 3 iterations. This means I need to read the sequence at 4 positions simultaneously (4 cursors), each cursor reading samples 3 by 3. Let's add a parameter that controls the space between the cursors:
batch_size = 4
time_depth = 3
inter_cursor_space = 5
How can I define an iterator such that calling iterator.get_next()["index"]
in a session returns
with tf.Session() as sess:
sess.run(iterator.initializer)
# first call:
print(sess.run(next_sample["index"]))
# should print
# [[0, 5, 10, 15],
# [1, 6, 11, 16],
# [2, 7, 12, 17]]
# second call:
print(sess.run(next_sample["index"]))
# should print
# [[3, 8, 13, 18],
# [4, 9, 14, 19],
# [5, 10, 15, 20]]
# shape = [time_depth, batch_size]
Such the this iterator is compatible with the tf.nn.static_rnn
function.
python tensorflow lstm tensorflow-datasets
add a comment |
I have a working solution for my problem, but I find it relatively slow, and I would be surprised if there is no better way of doing what I want. See the description of the problem below.
CURRENT SOLUTION: (comes first, as it might be simpler for experienced users of tensorflow to infer what I want to do from this code snippet)
def stack_batch_dim(*x):
return {key: tf.stack([b[key] for b in x], axis=1) for key in x[0]}
dataset = tf.data.TFRecordDataset(filenames)
dataset = dataset.map(map_func=parse_box2d_protobuf, num_parallel_calls=8)
dataset = dataset.repeat()
# place the "cursors":
datasets = [dataset.skip(t * inter_cursor_space) for t in range(batch_size)]
dataset = tf.data.Dataset.zip(tuple(datasets))
# consume time_depth samples at a time
dataset = dataset.batch(time_depth)
# the actual batch dimension is a tuple and not a tensor
# need to "stack" the tuple into a tensor
dataset = dataset.map(stack_batch_dim)
iterator = dataset.make_initializable_iterator()
So I would like train an LSTM network with a sequence of data points. The data I have is stored in tfrecords, (the recommended way of storing data with tensorflow).
dataset = tf.data.TFRecordDataset(filenames)
dataset = dataset.map(map_func=parse_box2d_protobuf, num_parallel_calls=8)
# parse_box2d_protobuf is a function that transforms raw bytes into
# a python structure of the form:
# {"positions": tensor_of_shape_4,
# "speeds": tensor_of_shape_4,
# "action": tensor_of_shape_4,
# "vision": tensor_of_shape_h_w_3,
# "index": tensor_int64}
iterator = dataset.make_initializable_iterator()
next_sample = iterator.get_next()
with tf.Session() as sess:
sess.run(iterator.initializer)
print(sess.run(next_sample))
# prints a dictionary as expected.
Now what I want to do is to read that sequence in order to feed it in an LSTM in batches.
As you might expect, the "index" key in the dictionary corresponds to an integer that is the index of the data sample in the sequence (starting from 0):
with tf.Session() as sess:
sess.run(iterator.initializer)
for i in range(10, 15):
print(sess.run(next_sample["index"]))
# prints successively 0, 1, 2, 3 and 4
Now let's say I want a batch size of 4, and I want to unfold the LSTM net for 3 iterations. This means I need to read the sequence at 4 positions simultaneously (4 cursors), each cursor reading samples 3 by 3. Let's add a parameter that controls the space between the cursors:
batch_size = 4
time_depth = 3
inter_cursor_space = 5
How can I define an iterator such that calling iterator.get_next()["index"]
in a session returns
with tf.Session() as sess:
sess.run(iterator.initializer)
# first call:
print(sess.run(next_sample["index"]))
# should print
# [[0, 5, 10, 15],
# [1, 6, 11, 16],
# [2, 7, 12, 17]]
# second call:
print(sess.run(next_sample["index"]))
# should print
# [[3, 8, 13, 18],
# [4, 9, 14, 19],
# [5, 10, 15, 20]]
# shape = [time_depth, batch_size]
Such the this iterator is compatible with the tf.nn.static_rnn
function.
python tensorflow lstm tensorflow-datasets
add a comment |
I have a working solution for my problem, but I find it relatively slow, and I would be surprised if there is no better way of doing what I want. See the description of the problem below.
CURRENT SOLUTION: (comes first, as it might be simpler for experienced users of tensorflow to infer what I want to do from this code snippet)
def stack_batch_dim(*x):
return {key: tf.stack([b[key] for b in x], axis=1) for key in x[0]}
dataset = tf.data.TFRecordDataset(filenames)
dataset = dataset.map(map_func=parse_box2d_protobuf, num_parallel_calls=8)
dataset = dataset.repeat()
# place the "cursors":
datasets = [dataset.skip(t * inter_cursor_space) for t in range(batch_size)]
dataset = tf.data.Dataset.zip(tuple(datasets))
# consume time_depth samples at a time
dataset = dataset.batch(time_depth)
# the actual batch dimension is a tuple and not a tensor
# need to "stack" the tuple into a tensor
dataset = dataset.map(stack_batch_dim)
iterator = dataset.make_initializable_iterator()
So I would like train an LSTM network with a sequence of data points. The data I have is stored in tfrecords, (the recommended way of storing data with tensorflow).
dataset = tf.data.TFRecordDataset(filenames)
dataset = dataset.map(map_func=parse_box2d_protobuf, num_parallel_calls=8)
# parse_box2d_protobuf is a function that transforms raw bytes into
# a python structure of the form:
# {"positions": tensor_of_shape_4,
# "speeds": tensor_of_shape_4,
# "action": tensor_of_shape_4,
# "vision": tensor_of_shape_h_w_3,
# "index": tensor_int64}
iterator = dataset.make_initializable_iterator()
next_sample = iterator.get_next()
with tf.Session() as sess:
sess.run(iterator.initializer)
print(sess.run(next_sample))
# prints a dictionary as expected.
Now what I want to do is to read that sequence in order to feed it in an LSTM in batches.
As you might expect, the "index" key in the dictionary corresponds to an integer that is the index of the data sample in the sequence (starting from 0):
with tf.Session() as sess:
sess.run(iterator.initializer)
for i in range(10, 15):
print(sess.run(next_sample["index"]))
# prints successively 0, 1, 2, 3 and 4
Now let's say I want a batch size of 4, and I want to unfold the LSTM net for 3 iterations. This means I need to read the sequence at 4 positions simultaneously (4 cursors), each cursor reading samples 3 by 3. Let's add a parameter that controls the space between the cursors:
batch_size = 4
time_depth = 3
inter_cursor_space = 5
How can I define an iterator such that calling iterator.get_next()["index"]
in a session returns
with tf.Session() as sess:
sess.run(iterator.initializer)
# first call:
print(sess.run(next_sample["index"]))
# should print
# [[0, 5, 10, 15],
# [1, 6, 11, 16],
# [2, 7, 12, 17]]
# second call:
print(sess.run(next_sample["index"]))
# should print
# [[3, 8, 13, 18],
# [4, 9, 14, 19],
# [5, 10, 15, 20]]
# shape = [time_depth, batch_size]
Such the this iterator is compatible with the tf.nn.static_rnn
function.
python tensorflow lstm tensorflow-datasets
I have a working solution for my problem, but I find it relatively slow, and I would be surprised if there is no better way of doing what I want. See the description of the problem below.
CURRENT SOLUTION: (comes first, as it might be simpler for experienced users of tensorflow to infer what I want to do from this code snippet)
def stack_batch_dim(*x):
return {key: tf.stack([b[key] for b in x], axis=1) for key in x[0]}
dataset = tf.data.TFRecordDataset(filenames)
dataset = dataset.map(map_func=parse_box2d_protobuf, num_parallel_calls=8)
dataset = dataset.repeat()
# place the "cursors":
datasets = [dataset.skip(t * inter_cursor_space) for t in range(batch_size)]
dataset = tf.data.Dataset.zip(tuple(datasets))
# consume time_depth samples at a time
dataset = dataset.batch(time_depth)
# the actual batch dimension is a tuple and not a tensor
# need to "stack" the tuple into a tensor
dataset = dataset.map(stack_batch_dim)
iterator = dataset.make_initializable_iterator()
So I would like train an LSTM network with a sequence of data points. The data I have is stored in tfrecords, (the recommended way of storing data with tensorflow).
dataset = tf.data.TFRecordDataset(filenames)
dataset = dataset.map(map_func=parse_box2d_protobuf, num_parallel_calls=8)
# parse_box2d_protobuf is a function that transforms raw bytes into
# a python structure of the form:
# {"positions": tensor_of_shape_4,
# "speeds": tensor_of_shape_4,
# "action": tensor_of_shape_4,
# "vision": tensor_of_shape_h_w_3,
# "index": tensor_int64}
iterator = dataset.make_initializable_iterator()
next_sample = iterator.get_next()
with tf.Session() as sess:
sess.run(iterator.initializer)
print(sess.run(next_sample))
# prints a dictionary as expected.
Now what I want to do is to read that sequence in order to feed it in an LSTM in batches.
As you might expect, the "index" key in the dictionary corresponds to an integer that is the index of the data sample in the sequence (starting from 0):
with tf.Session() as sess:
sess.run(iterator.initializer)
for i in range(10, 15):
print(sess.run(next_sample["index"]))
# prints successively 0, 1, 2, 3 and 4
Now let's say I want a batch size of 4, and I want to unfold the LSTM net for 3 iterations. This means I need to read the sequence at 4 positions simultaneously (4 cursors), each cursor reading samples 3 by 3. Let's add a parameter that controls the space between the cursors:
batch_size = 4
time_depth = 3
inter_cursor_space = 5
How can I define an iterator such that calling iterator.get_next()["index"]
in a session returns
with tf.Session() as sess:
sess.run(iterator.initializer)
# first call:
print(sess.run(next_sample["index"]))
# should print
# [[0, 5, 10, 15],
# [1, 6, 11, 16],
# [2, 7, 12, 17]]
# second call:
print(sess.run(next_sample["index"]))
# should print
# [[3, 8, 13, 18],
# [4, 9, 14, 19],
# [5, 10, 15, 20]]
# shape = [time_depth, batch_size]
Such the this iterator is compatible with the tf.nn.static_rnn
function.
python tensorflow lstm tensorflow-datasets
python tensorflow lstm tensorflow-datasets
asked Nov 21 '18 at 13:42
user3767336user3767336
364
364
add a comment |
add a comment |
0
active
oldest
votes
Your Answer
StackExchange.ifUsing("editor", function () {
StackExchange.using("externalEditor", function () {
StackExchange.using("snippets", function () {
StackExchange.snippets.init();
});
});
}, "code-snippets");
StackExchange.ready(function() {
var channelOptions = {
tags: "".split(" "),
id: "1"
};
initTagRenderer("".split(" "), "".split(" "), channelOptions);
StackExchange.using("externalEditor", function() {
// Have to fire editor after snippets, if snippets enabled
if (StackExchange.settings.snippets.snippetsEnabled) {
StackExchange.using("snippets", function() {
createEditor();
});
}
else {
createEditor();
}
});
function createEditor() {
StackExchange.prepareEditor({
heartbeatType: 'answer',
autoActivateHeartbeat: false,
convertImagesToLinks: true,
noModals: true,
showLowRepImageUploadWarning: true,
reputationToPostImages: 10,
bindNavPrevention: true,
postfix: "",
imageUploader: {
brandingHtml: "Powered by u003ca class="icon-imgur-white" href="https://imgur.com/"u003eu003c/au003e",
contentPolicyHtml: "User contributions licensed under u003ca href="https://creativecommons.org/licenses/by-sa/3.0/"u003ecc by-sa 3.0 with attribution requiredu003c/au003e u003ca href="https://stackoverflow.com/legal/content-policy"u003e(content policy)u003c/au003e",
allowUrls: true
},
onDemand: true,
discardSelector: ".discard-answer"
,immediatelyShowMarkdownHelp:true
});
}
});
Sign up or log in
StackExchange.ready(function () {
StackExchange.helpers.onClickDraftSave('#login-link');
});
Sign up using Google
Sign up using Facebook
Sign up using Email and Password
Post as a guest
Required, but never shown
StackExchange.ready(
function () {
StackExchange.openid.initPostLogin('.new-post-login', 'https%3a%2f%2fstackoverflow.com%2fquestions%2f53413407%2fprocessing-time-series-with-the-tensorflow-dataset-api-for-feeding-an-lstm-in-ba%23new-answer', 'question_page');
}
);
Post as a guest
Required, but never shown
0
active
oldest
votes
0
active
oldest
votes
active
oldest
votes
active
oldest
votes
Thanks for contributing an answer to Stack Overflow!
- Please be sure to answer the question. Provide details and share your research!
But avoid …
- Asking for help, clarification, or responding to other answers.
- Making statements based on opinion; back them up with references or personal experience.
To learn more, see our tips on writing great answers.
Sign up or log in
StackExchange.ready(function () {
StackExchange.helpers.onClickDraftSave('#login-link');
});
Sign up using Google
Sign up using Facebook
Sign up using Email and Password
Post as a guest
Required, but never shown
StackExchange.ready(
function () {
StackExchange.openid.initPostLogin('.new-post-login', 'https%3a%2f%2fstackoverflow.com%2fquestions%2f53413407%2fprocessing-time-series-with-the-tensorflow-dataset-api-for-feeding-an-lstm-in-ba%23new-answer', 'question_page');
}
);
Post as a guest
Required, but never shown
Sign up or log in
StackExchange.ready(function () {
StackExchange.helpers.onClickDraftSave('#login-link');
});
Sign up using Google
Sign up using Facebook
Sign up using Email and Password
Post as a guest
Required, but never shown
Sign up or log in
StackExchange.ready(function () {
StackExchange.helpers.onClickDraftSave('#login-link');
});
Sign up using Google
Sign up using Facebook
Sign up using Email and Password
Post as a guest
Required, but never shown
Sign up or log in
StackExchange.ready(function () {
StackExchange.helpers.onClickDraftSave('#login-link');
});
Sign up using Google
Sign up using Facebook
Sign up using Email and Password
Sign up using Google
Sign up using Facebook
Sign up using Email and Password
Post as a guest
Required, but never shown
Required, but never shown
Required, but never shown
Required, but never shown
Required, but never shown
Required, but never shown
Required, but never shown
Required, but never shown
Required, but never shown
c0mxuVuA9t2F2izX108rp9tH6fyqemaFTea9FX6Jzjlfx YbR0cD,ajY,L6,MwH,Aea3wfmUYWyVnmymO,NNv XMRjOHn5P3I,p2cZA