Problem of statistical inference Poisson
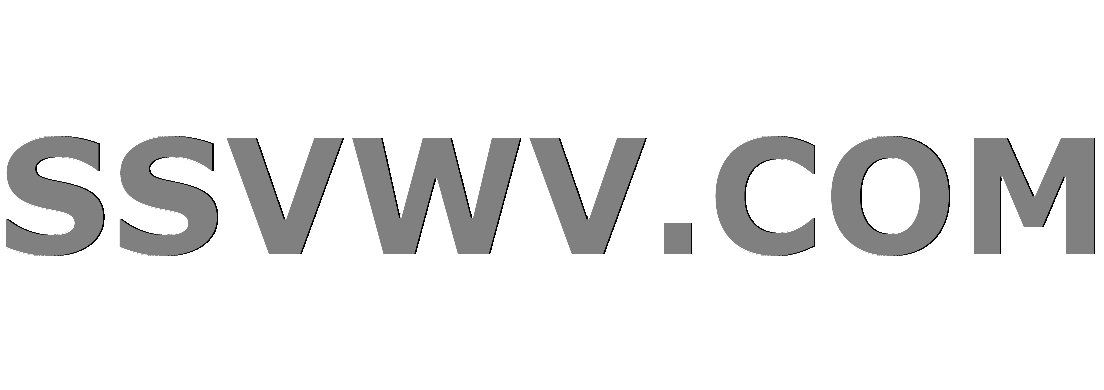
Multi tool use
$begingroup$
I am having problems solving this problem of statistical inference and I do not know if it is well done or not, so I would like someone to review it. I just started with inference, so I have my problems.
-The weekly number of failures due to software problems that have occurred in a computer system are the following:
Number fails 0 1 2 3 4 5 6 8
Frequency 41 62 63 38 12 7 1 1
1) Can it be accepted that the weekly number of failures due to software problems that occur in that computer system is a random variable with Poisson distribution?
What I have to do is calculate the chi-square, which I get from Chi-Square values = 379,682 with 11 g.l. Value-P = 0,0
It does not fit me since it gives me a p-value of 0 and it never happened to me, so I do not know if you could tell me that this result is true
2) Assuming that the weekly number of failures due to software problems is distributed according to a law Poisson: Calculate an unbiased estimate of the weekly rate of system failures due to software problems.
What I have to calculate is a mean that the best estimator (and also unbiased is the sample mean) that has a value of 1.77333 to be unbiased E (Estimation) = μ and because E (Average) = μ
We concluded that he would be unbiased.
3) Calculate a level confidence interval of at least 0.94 for the distribution parameter.
I have this, but I am not very confident that it is correct:
Average (x): 1,77333
Standard deviation (s): 1.36185
(x +/- Ks)
100 (1-1 / K ^ 2) = 94; K = 4.0824
1.7733 +/- 4.0824 * 1.36185 = [-3.7863, 7,3329]
4) Calculate a confidence interval of approximate level 0.94 for the distribution parameter using the central limit theorem.
Of the sample rate I have my own mean which is 1.7733 and of null hypothesis, as they do not tell me anything, I suppose 0.5 for n = 225 and I get the IC [1.61016, 1.94868]
5) Can it be accepted, at a level of 2%, that, as the engineer responsible for the system says, the weekly failure rate is at most 1.6? And at a level of 5%?
It is the same exercise as the previous one but with a null hypothesis of 1.6, for an average of 1.7733 and n = 225. As it tells me that it is unilateral less than (HERE I HAVE DOUBTS IF IT IS UNILATERAL LESS THAN) and with the p-value of 0.981321 I conclude that I can not reject the null hypothesis for alpha 0.02.
For 5% I get the same p-value and same conclusion of rejection
statistics statistical-inference poisson-distribution sampling
$endgroup$
add a comment |
$begingroup$
I am having problems solving this problem of statistical inference and I do not know if it is well done or not, so I would like someone to review it. I just started with inference, so I have my problems.
-The weekly number of failures due to software problems that have occurred in a computer system are the following:
Number fails 0 1 2 3 4 5 6 8
Frequency 41 62 63 38 12 7 1 1
1) Can it be accepted that the weekly number of failures due to software problems that occur in that computer system is a random variable with Poisson distribution?
What I have to do is calculate the chi-square, which I get from Chi-Square values = 379,682 with 11 g.l. Value-P = 0,0
It does not fit me since it gives me a p-value of 0 and it never happened to me, so I do not know if you could tell me that this result is true
2) Assuming that the weekly number of failures due to software problems is distributed according to a law Poisson: Calculate an unbiased estimate of the weekly rate of system failures due to software problems.
What I have to calculate is a mean that the best estimator (and also unbiased is the sample mean) that has a value of 1.77333 to be unbiased E (Estimation) = μ and because E (Average) = μ
We concluded that he would be unbiased.
3) Calculate a level confidence interval of at least 0.94 for the distribution parameter.
I have this, but I am not very confident that it is correct:
Average (x): 1,77333
Standard deviation (s): 1.36185
(x +/- Ks)
100 (1-1 / K ^ 2) = 94; K = 4.0824
1.7733 +/- 4.0824 * 1.36185 = [-3.7863, 7,3329]
4) Calculate a confidence interval of approximate level 0.94 for the distribution parameter using the central limit theorem.
Of the sample rate I have my own mean which is 1.7733 and of null hypothesis, as they do not tell me anything, I suppose 0.5 for n = 225 and I get the IC [1.61016, 1.94868]
5) Can it be accepted, at a level of 2%, that, as the engineer responsible for the system says, the weekly failure rate is at most 1.6? And at a level of 5%?
It is the same exercise as the previous one but with a null hypothesis of 1.6, for an average of 1.7733 and n = 225. As it tells me that it is unilateral less than (HERE I HAVE DOUBTS IF IT IS UNILATERAL LESS THAN) and with the p-value of 0.981321 I conclude that I can not reject the null hypothesis for alpha 0.02.
For 5% I get the same p-value and same conclusion of rejection
statistics statistical-inference poisson-distribution sampling
$endgroup$
add a comment |
$begingroup$
I am having problems solving this problem of statistical inference and I do not know if it is well done or not, so I would like someone to review it. I just started with inference, so I have my problems.
-The weekly number of failures due to software problems that have occurred in a computer system are the following:
Number fails 0 1 2 3 4 5 6 8
Frequency 41 62 63 38 12 7 1 1
1) Can it be accepted that the weekly number of failures due to software problems that occur in that computer system is a random variable with Poisson distribution?
What I have to do is calculate the chi-square, which I get from Chi-Square values = 379,682 with 11 g.l. Value-P = 0,0
It does not fit me since it gives me a p-value of 0 and it never happened to me, so I do not know if you could tell me that this result is true
2) Assuming that the weekly number of failures due to software problems is distributed according to a law Poisson: Calculate an unbiased estimate of the weekly rate of system failures due to software problems.
What I have to calculate is a mean that the best estimator (and also unbiased is the sample mean) that has a value of 1.77333 to be unbiased E (Estimation) = μ and because E (Average) = μ
We concluded that he would be unbiased.
3) Calculate a level confidence interval of at least 0.94 for the distribution parameter.
I have this, but I am not very confident that it is correct:
Average (x): 1,77333
Standard deviation (s): 1.36185
(x +/- Ks)
100 (1-1 / K ^ 2) = 94; K = 4.0824
1.7733 +/- 4.0824 * 1.36185 = [-3.7863, 7,3329]
4) Calculate a confidence interval of approximate level 0.94 for the distribution parameter using the central limit theorem.
Of the sample rate I have my own mean which is 1.7733 and of null hypothesis, as they do not tell me anything, I suppose 0.5 for n = 225 and I get the IC [1.61016, 1.94868]
5) Can it be accepted, at a level of 2%, that, as the engineer responsible for the system says, the weekly failure rate is at most 1.6? And at a level of 5%?
It is the same exercise as the previous one but with a null hypothesis of 1.6, for an average of 1.7733 and n = 225. As it tells me that it is unilateral less than (HERE I HAVE DOUBTS IF IT IS UNILATERAL LESS THAN) and with the p-value of 0.981321 I conclude that I can not reject the null hypothesis for alpha 0.02.
For 5% I get the same p-value and same conclusion of rejection
statistics statistical-inference poisson-distribution sampling
$endgroup$
I am having problems solving this problem of statistical inference and I do not know if it is well done or not, so I would like someone to review it. I just started with inference, so I have my problems.
-The weekly number of failures due to software problems that have occurred in a computer system are the following:
Number fails 0 1 2 3 4 5 6 8
Frequency 41 62 63 38 12 7 1 1
1) Can it be accepted that the weekly number of failures due to software problems that occur in that computer system is a random variable with Poisson distribution?
What I have to do is calculate the chi-square, which I get from Chi-Square values = 379,682 with 11 g.l. Value-P = 0,0
It does not fit me since it gives me a p-value of 0 and it never happened to me, so I do not know if you could tell me that this result is true
2) Assuming that the weekly number of failures due to software problems is distributed according to a law Poisson: Calculate an unbiased estimate of the weekly rate of system failures due to software problems.
What I have to calculate is a mean that the best estimator (and also unbiased is the sample mean) that has a value of 1.77333 to be unbiased E (Estimation) = μ and because E (Average) = μ
We concluded that he would be unbiased.
3) Calculate a level confidence interval of at least 0.94 for the distribution parameter.
I have this, but I am not very confident that it is correct:
Average (x): 1,77333
Standard deviation (s): 1.36185
(x +/- Ks)
100 (1-1 / K ^ 2) = 94; K = 4.0824
1.7733 +/- 4.0824 * 1.36185 = [-3.7863, 7,3329]
4) Calculate a confidence interval of approximate level 0.94 for the distribution parameter using the central limit theorem.
Of the sample rate I have my own mean which is 1.7733 and of null hypothesis, as they do not tell me anything, I suppose 0.5 for n = 225 and I get the IC [1.61016, 1.94868]
5) Can it be accepted, at a level of 2%, that, as the engineer responsible for the system says, the weekly failure rate is at most 1.6? And at a level of 5%?
It is the same exercise as the previous one but with a null hypothesis of 1.6, for an average of 1.7733 and n = 225. As it tells me that it is unilateral less than (HERE I HAVE DOUBTS IF IT IS UNILATERAL LESS THAN) and with the p-value of 0.981321 I conclude that I can not reject the null hypothesis for alpha 0.02.
For 5% I get the same p-value and same conclusion of rejection
statistics statistical-inference poisson-distribution sampling
statistics statistical-inference poisson-distribution sampling
edited Dec 2 '18 at 12:11
Fernando
asked Nov 29 '18 at 18:08
FernandoFernando
495
495
add a comment |
add a comment |
1 Answer
1
active
oldest
votes
$begingroup$
Here is an outline of the procedure you need to follow:
Goodness-of-fit to Poisson. Let the frequencies $f_i = 41, 62, 63, dots$
and the values be $k_i = 0, 1, 2, dots .$
Then estimate the population mean as
$hat lambda = frac 1 n sum_{i=1}^9 k_i f_i = 1.7733,$ where
$n = sum_{i=1}^9 f_i = 225.$
Then use observed category counts $X_j = 0, 1, 2, 3, 4,$ and $ge 5,$ where the last count is $7 = 1 + 0 + 1 = 9.$
Then according to the distribution $mathsf{Pois}(hat lambda = 1.7733),$ find the probabilities of each of
the categories, and multiply them by the sample size
$n$ to obtain expected counts $E_j.$
Contributions $C_j = frac {(X_j - E_j)^2}{E_j}$ to the chi-squared statistic
$Q = sum_j C_j = 2.096$ are shown in the table below along with the
$X_j$ and $E_j.$
X E C
41 38.198659 0.2054395
62 67.737681 0.4860070
63 60.059615 0.1439547
38 35.501239 0.1758758
12 15.738587 0.8880740
9 7.764219 0.1966912
Under the null hypothesis that data are Poisson,
the 'chi-squared' statistic $Q$ is approximately
distributed according to the distribution $mathsf{Chisq}(text{df} = 6-2 = 4).$ The null
hypothesis is not rejected at the 5% level because
the critical value for the test is $c = 9.488 > 2.096;$ you can find $c$
in printed tables of the chi-squared
distribution.
It was necessary to combine original counts 7, 1, 0, 1
into one category in order to have the last of the $E_j$'s exceed $5,$ assuring a reasonable fit of the
null distribution to chi-squared.
I have tried to be careful with my computations in this procedural outline, but
you should check them.
Confidence interval for Poisson mean. I am not sure what style of confidence interval you are
using for the Poisson mean $lambda$ based on the
estimate $hat lambda;$ I don't know what you mean
by 'of at least 0.94', I don't see the rationale for your value of $k,$ and I can't follow all of your computer code.
Also, I'm suspicious of the validity of a CI for $lambda > 0$ with
a lower endpoint that strays so far into negative
territory.
A Wald 95% CI for $nlambda$ is of the form $nhatlambda pm 1.96sqrt{nhatlambda}.$ Divide the endpoints by $n$ to get a 95% CI $(1.610, 1.937)$
for $lambda.$ [This is an asymptotic interval and
so it should be reasonably accurate for a
sample of size as large as $n = 255.]$
It has been suggested that a more accurate CI for $nlambda$ is of the form
$nhatlambda + 2 pm 1.96sqrt{nhatlambda + 1},$ but for $n = 255,$ the adjustments don't
make much difference. The corresponding result for $lambda$ is
$(1.618, 1.945).$
There must be at least
half a dozen styles of Poisson CIs in use, each with enthusiastic adherents, and I don't claim mine is 'best'. Perhaps we will get some
recommendations from others on this site.
A simple simulation (in R) seems to show that this interval has about 95% actual coverage for
$lambda$ and $n$ of the sizes we have here.
set.seed(1201)
lam = 1.7733; n = 255
lam.tot = replicate(10^5, sum(rpois(n, lam)))
lcl = (lam.tot + 2 - 1.96*sqrt(lam.tot + 1))/n
ucl = (lam.tot + 2 + 1.96*sqrt(lam.tot + 1))/n
mean(lcl < lam & ucl > lam)
[1] 0.94997
$endgroup$
$begingroup$
First, thank you for responding. I was calculating things thanks to his explanation and I have obtained something different. In the first part I get a statistic of 2.27488 degrees of freedom (6-1) = 5 and p-value = 0.809949, so I do not reject a null hypothesis for 0.05. In the second question, an unbiased estimator is the sample mean, that is, 1.7733 since their expectations coincide. In question three, they ask me for an IC <level at least>, so I have calculated it with X - + (sigma / sqrt (n alpha)) and I get [1.40265, 2.143959] at 94%
$endgroup$
– Fernando
Dec 2 '18 at 7:49
$begingroup$
I have edited the question with a continuation of the exercise that I do not know if it is well done and I would appreciate if you could tell me if it is well done or not
$endgroup$
– Fernando
Dec 2 '18 at 8:02
$begingroup$
Don't understand how you got 2.275. What is it? What formula? // DF = 6-2 = 4. Ordinarily, DF is one less than number of categories, but you lose one DF for estimating $lambda$ by $hatlambda.$ // If you're testing $H_0: lambda = 1.6$ against $H_a: lambda ne 1.6$ then you'd reject at 6% level if 1.6 is outside 94% CI. // Not sure how you got CI in your comment. Strange you're using 94% CI, usual confidence level is 95%. 94% CI should be shorter than 95% CI. Are you confusing $alpha$ and $sigma?$
$endgroup$
– BruceET
Dec 2 '18 at 9:45
$begingroup$
I am using a program to do the calculations, apart from doing it by hand. DF is always one less than the categories but in this case 2 are lost by the estimation and the categories?
$endgroup$
– Fernando
Dec 2 '18 at 12:12
$begingroup$
I Edit the question and I put a number to each question.// In 3, I think that the calculated is at an approximate level, but in the statement puts <at least level>, so use the formula that I put in the previous comment X - + (σ/ sqrt (n * α )) . (being X the average) // In 4, I think the result is what was obtained in section 3 and here it is <a approximate level>. // In 5, I have doubts if what they ask me is unilateral right or left unilateral
$endgroup$
– Fernando
Dec 2 '18 at 12:18
add a comment |
Your Answer
StackExchange.ifUsing("editor", function () {
return StackExchange.using("mathjaxEditing", function () {
StackExchange.MarkdownEditor.creationCallbacks.add(function (editor, postfix) {
StackExchange.mathjaxEditing.prepareWmdForMathJax(editor, postfix, [["$", "$"], ["\\(","\\)"]]);
});
});
}, "mathjax-editing");
StackExchange.ready(function() {
var channelOptions = {
tags: "".split(" "),
id: "69"
};
initTagRenderer("".split(" "), "".split(" "), channelOptions);
StackExchange.using("externalEditor", function() {
// Have to fire editor after snippets, if snippets enabled
if (StackExchange.settings.snippets.snippetsEnabled) {
StackExchange.using("snippets", function() {
createEditor();
});
}
else {
createEditor();
}
});
function createEditor() {
StackExchange.prepareEditor({
heartbeatType: 'answer',
autoActivateHeartbeat: false,
convertImagesToLinks: true,
noModals: true,
showLowRepImageUploadWarning: true,
reputationToPostImages: 10,
bindNavPrevention: true,
postfix: "",
imageUploader: {
brandingHtml: "Powered by u003ca class="icon-imgur-white" href="https://imgur.com/"u003eu003c/au003e",
contentPolicyHtml: "User contributions licensed under u003ca href="https://creativecommons.org/licenses/by-sa/3.0/"u003ecc by-sa 3.0 with attribution requiredu003c/au003e u003ca href="https://stackoverflow.com/legal/content-policy"u003e(content policy)u003c/au003e",
allowUrls: true
},
noCode: true, onDemand: true,
discardSelector: ".discard-answer"
,immediatelyShowMarkdownHelp:true
});
}
});
Sign up or log in
StackExchange.ready(function () {
StackExchange.helpers.onClickDraftSave('#login-link');
});
Sign up using Google
Sign up using Facebook
Sign up using Email and Password
Post as a guest
Required, but never shown
StackExchange.ready(
function () {
StackExchange.openid.initPostLogin('.new-post-login', 'https%3a%2f%2fmath.stackexchange.com%2fquestions%2f3018972%2fproblem-of-statistical-inference-poisson%23new-answer', 'question_page');
}
);
Post as a guest
Required, but never shown
1 Answer
1
active
oldest
votes
1 Answer
1
active
oldest
votes
active
oldest
votes
active
oldest
votes
$begingroup$
Here is an outline of the procedure you need to follow:
Goodness-of-fit to Poisson. Let the frequencies $f_i = 41, 62, 63, dots$
and the values be $k_i = 0, 1, 2, dots .$
Then estimate the population mean as
$hat lambda = frac 1 n sum_{i=1}^9 k_i f_i = 1.7733,$ where
$n = sum_{i=1}^9 f_i = 225.$
Then use observed category counts $X_j = 0, 1, 2, 3, 4,$ and $ge 5,$ where the last count is $7 = 1 + 0 + 1 = 9.$
Then according to the distribution $mathsf{Pois}(hat lambda = 1.7733),$ find the probabilities of each of
the categories, and multiply them by the sample size
$n$ to obtain expected counts $E_j.$
Contributions $C_j = frac {(X_j - E_j)^2}{E_j}$ to the chi-squared statistic
$Q = sum_j C_j = 2.096$ are shown in the table below along with the
$X_j$ and $E_j.$
X E C
41 38.198659 0.2054395
62 67.737681 0.4860070
63 60.059615 0.1439547
38 35.501239 0.1758758
12 15.738587 0.8880740
9 7.764219 0.1966912
Under the null hypothesis that data are Poisson,
the 'chi-squared' statistic $Q$ is approximately
distributed according to the distribution $mathsf{Chisq}(text{df} = 6-2 = 4).$ The null
hypothesis is not rejected at the 5% level because
the critical value for the test is $c = 9.488 > 2.096;$ you can find $c$
in printed tables of the chi-squared
distribution.
It was necessary to combine original counts 7, 1, 0, 1
into one category in order to have the last of the $E_j$'s exceed $5,$ assuring a reasonable fit of the
null distribution to chi-squared.
I have tried to be careful with my computations in this procedural outline, but
you should check them.
Confidence interval for Poisson mean. I am not sure what style of confidence interval you are
using for the Poisson mean $lambda$ based on the
estimate $hat lambda;$ I don't know what you mean
by 'of at least 0.94', I don't see the rationale for your value of $k,$ and I can't follow all of your computer code.
Also, I'm suspicious of the validity of a CI for $lambda > 0$ with
a lower endpoint that strays so far into negative
territory.
A Wald 95% CI for $nlambda$ is of the form $nhatlambda pm 1.96sqrt{nhatlambda}.$ Divide the endpoints by $n$ to get a 95% CI $(1.610, 1.937)$
for $lambda.$ [This is an asymptotic interval and
so it should be reasonably accurate for a
sample of size as large as $n = 255.]$
It has been suggested that a more accurate CI for $nlambda$ is of the form
$nhatlambda + 2 pm 1.96sqrt{nhatlambda + 1},$ but for $n = 255,$ the adjustments don't
make much difference. The corresponding result for $lambda$ is
$(1.618, 1.945).$
There must be at least
half a dozen styles of Poisson CIs in use, each with enthusiastic adherents, and I don't claim mine is 'best'. Perhaps we will get some
recommendations from others on this site.
A simple simulation (in R) seems to show that this interval has about 95% actual coverage for
$lambda$ and $n$ of the sizes we have here.
set.seed(1201)
lam = 1.7733; n = 255
lam.tot = replicate(10^5, sum(rpois(n, lam)))
lcl = (lam.tot + 2 - 1.96*sqrt(lam.tot + 1))/n
ucl = (lam.tot + 2 + 1.96*sqrt(lam.tot + 1))/n
mean(lcl < lam & ucl > lam)
[1] 0.94997
$endgroup$
$begingroup$
First, thank you for responding. I was calculating things thanks to his explanation and I have obtained something different. In the first part I get a statistic of 2.27488 degrees of freedom (6-1) = 5 and p-value = 0.809949, so I do not reject a null hypothesis for 0.05. In the second question, an unbiased estimator is the sample mean, that is, 1.7733 since their expectations coincide. In question three, they ask me for an IC <level at least>, so I have calculated it with X - + (sigma / sqrt (n alpha)) and I get [1.40265, 2.143959] at 94%
$endgroup$
– Fernando
Dec 2 '18 at 7:49
$begingroup$
I have edited the question with a continuation of the exercise that I do not know if it is well done and I would appreciate if you could tell me if it is well done or not
$endgroup$
– Fernando
Dec 2 '18 at 8:02
$begingroup$
Don't understand how you got 2.275. What is it? What formula? // DF = 6-2 = 4. Ordinarily, DF is one less than number of categories, but you lose one DF for estimating $lambda$ by $hatlambda.$ // If you're testing $H_0: lambda = 1.6$ against $H_a: lambda ne 1.6$ then you'd reject at 6% level if 1.6 is outside 94% CI. // Not sure how you got CI in your comment. Strange you're using 94% CI, usual confidence level is 95%. 94% CI should be shorter than 95% CI. Are you confusing $alpha$ and $sigma?$
$endgroup$
– BruceET
Dec 2 '18 at 9:45
$begingroup$
I am using a program to do the calculations, apart from doing it by hand. DF is always one less than the categories but in this case 2 are lost by the estimation and the categories?
$endgroup$
– Fernando
Dec 2 '18 at 12:12
$begingroup$
I Edit the question and I put a number to each question.// In 3, I think that the calculated is at an approximate level, but in the statement puts <at least level>, so use the formula that I put in the previous comment X - + (σ/ sqrt (n * α )) . (being X the average) // In 4, I think the result is what was obtained in section 3 and here it is <a approximate level>. // In 5, I have doubts if what they ask me is unilateral right or left unilateral
$endgroup$
– Fernando
Dec 2 '18 at 12:18
add a comment |
$begingroup$
Here is an outline of the procedure you need to follow:
Goodness-of-fit to Poisson. Let the frequencies $f_i = 41, 62, 63, dots$
and the values be $k_i = 0, 1, 2, dots .$
Then estimate the population mean as
$hat lambda = frac 1 n sum_{i=1}^9 k_i f_i = 1.7733,$ where
$n = sum_{i=1}^9 f_i = 225.$
Then use observed category counts $X_j = 0, 1, 2, 3, 4,$ and $ge 5,$ where the last count is $7 = 1 + 0 + 1 = 9.$
Then according to the distribution $mathsf{Pois}(hat lambda = 1.7733),$ find the probabilities of each of
the categories, and multiply them by the sample size
$n$ to obtain expected counts $E_j.$
Contributions $C_j = frac {(X_j - E_j)^2}{E_j}$ to the chi-squared statistic
$Q = sum_j C_j = 2.096$ are shown in the table below along with the
$X_j$ and $E_j.$
X E C
41 38.198659 0.2054395
62 67.737681 0.4860070
63 60.059615 0.1439547
38 35.501239 0.1758758
12 15.738587 0.8880740
9 7.764219 0.1966912
Under the null hypothesis that data are Poisson,
the 'chi-squared' statistic $Q$ is approximately
distributed according to the distribution $mathsf{Chisq}(text{df} = 6-2 = 4).$ The null
hypothesis is not rejected at the 5% level because
the critical value for the test is $c = 9.488 > 2.096;$ you can find $c$
in printed tables of the chi-squared
distribution.
It was necessary to combine original counts 7, 1, 0, 1
into one category in order to have the last of the $E_j$'s exceed $5,$ assuring a reasonable fit of the
null distribution to chi-squared.
I have tried to be careful with my computations in this procedural outline, but
you should check them.
Confidence interval for Poisson mean. I am not sure what style of confidence interval you are
using for the Poisson mean $lambda$ based on the
estimate $hat lambda;$ I don't know what you mean
by 'of at least 0.94', I don't see the rationale for your value of $k,$ and I can't follow all of your computer code.
Also, I'm suspicious of the validity of a CI for $lambda > 0$ with
a lower endpoint that strays so far into negative
territory.
A Wald 95% CI for $nlambda$ is of the form $nhatlambda pm 1.96sqrt{nhatlambda}.$ Divide the endpoints by $n$ to get a 95% CI $(1.610, 1.937)$
for $lambda.$ [This is an asymptotic interval and
so it should be reasonably accurate for a
sample of size as large as $n = 255.]$
It has been suggested that a more accurate CI for $nlambda$ is of the form
$nhatlambda + 2 pm 1.96sqrt{nhatlambda + 1},$ but for $n = 255,$ the adjustments don't
make much difference. The corresponding result for $lambda$ is
$(1.618, 1.945).$
There must be at least
half a dozen styles of Poisson CIs in use, each with enthusiastic adherents, and I don't claim mine is 'best'. Perhaps we will get some
recommendations from others on this site.
A simple simulation (in R) seems to show that this interval has about 95% actual coverage for
$lambda$ and $n$ of the sizes we have here.
set.seed(1201)
lam = 1.7733; n = 255
lam.tot = replicate(10^5, sum(rpois(n, lam)))
lcl = (lam.tot + 2 - 1.96*sqrt(lam.tot + 1))/n
ucl = (lam.tot + 2 + 1.96*sqrt(lam.tot + 1))/n
mean(lcl < lam & ucl > lam)
[1] 0.94997
$endgroup$
$begingroup$
First, thank you for responding. I was calculating things thanks to his explanation and I have obtained something different. In the first part I get a statistic of 2.27488 degrees of freedom (6-1) = 5 and p-value = 0.809949, so I do not reject a null hypothesis for 0.05. In the second question, an unbiased estimator is the sample mean, that is, 1.7733 since their expectations coincide. In question three, they ask me for an IC <level at least>, so I have calculated it with X - + (sigma / sqrt (n alpha)) and I get [1.40265, 2.143959] at 94%
$endgroup$
– Fernando
Dec 2 '18 at 7:49
$begingroup$
I have edited the question with a continuation of the exercise that I do not know if it is well done and I would appreciate if you could tell me if it is well done or not
$endgroup$
– Fernando
Dec 2 '18 at 8:02
$begingroup$
Don't understand how you got 2.275. What is it? What formula? // DF = 6-2 = 4. Ordinarily, DF is one less than number of categories, but you lose one DF for estimating $lambda$ by $hatlambda.$ // If you're testing $H_0: lambda = 1.6$ against $H_a: lambda ne 1.6$ then you'd reject at 6% level if 1.6 is outside 94% CI. // Not sure how you got CI in your comment. Strange you're using 94% CI, usual confidence level is 95%. 94% CI should be shorter than 95% CI. Are you confusing $alpha$ and $sigma?$
$endgroup$
– BruceET
Dec 2 '18 at 9:45
$begingroup$
I am using a program to do the calculations, apart from doing it by hand. DF is always one less than the categories but in this case 2 are lost by the estimation and the categories?
$endgroup$
– Fernando
Dec 2 '18 at 12:12
$begingroup$
I Edit the question and I put a number to each question.// In 3, I think that the calculated is at an approximate level, but in the statement puts <at least level>, so use the formula that I put in the previous comment X - + (σ/ sqrt (n * α )) . (being X the average) // In 4, I think the result is what was obtained in section 3 and here it is <a approximate level>. // In 5, I have doubts if what they ask me is unilateral right or left unilateral
$endgroup$
– Fernando
Dec 2 '18 at 12:18
add a comment |
$begingroup$
Here is an outline of the procedure you need to follow:
Goodness-of-fit to Poisson. Let the frequencies $f_i = 41, 62, 63, dots$
and the values be $k_i = 0, 1, 2, dots .$
Then estimate the population mean as
$hat lambda = frac 1 n sum_{i=1}^9 k_i f_i = 1.7733,$ where
$n = sum_{i=1}^9 f_i = 225.$
Then use observed category counts $X_j = 0, 1, 2, 3, 4,$ and $ge 5,$ where the last count is $7 = 1 + 0 + 1 = 9.$
Then according to the distribution $mathsf{Pois}(hat lambda = 1.7733),$ find the probabilities of each of
the categories, and multiply them by the sample size
$n$ to obtain expected counts $E_j.$
Contributions $C_j = frac {(X_j - E_j)^2}{E_j}$ to the chi-squared statistic
$Q = sum_j C_j = 2.096$ are shown in the table below along with the
$X_j$ and $E_j.$
X E C
41 38.198659 0.2054395
62 67.737681 0.4860070
63 60.059615 0.1439547
38 35.501239 0.1758758
12 15.738587 0.8880740
9 7.764219 0.1966912
Under the null hypothesis that data are Poisson,
the 'chi-squared' statistic $Q$ is approximately
distributed according to the distribution $mathsf{Chisq}(text{df} = 6-2 = 4).$ The null
hypothesis is not rejected at the 5% level because
the critical value for the test is $c = 9.488 > 2.096;$ you can find $c$
in printed tables of the chi-squared
distribution.
It was necessary to combine original counts 7, 1, 0, 1
into one category in order to have the last of the $E_j$'s exceed $5,$ assuring a reasonable fit of the
null distribution to chi-squared.
I have tried to be careful with my computations in this procedural outline, but
you should check them.
Confidence interval for Poisson mean. I am not sure what style of confidence interval you are
using for the Poisson mean $lambda$ based on the
estimate $hat lambda;$ I don't know what you mean
by 'of at least 0.94', I don't see the rationale for your value of $k,$ and I can't follow all of your computer code.
Also, I'm suspicious of the validity of a CI for $lambda > 0$ with
a lower endpoint that strays so far into negative
territory.
A Wald 95% CI for $nlambda$ is of the form $nhatlambda pm 1.96sqrt{nhatlambda}.$ Divide the endpoints by $n$ to get a 95% CI $(1.610, 1.937)$
for $lambda.$ [This is an asymptotic interval and
so it should be reasonably accurate for a
sample of size as large as $n = 255.]$
It has been suggested that a more accurate CI for $nlambda$ is of the form
$nhatlambda + 2 pm 1.96sqrt{nhatlambda + 1},$ but for $n = 255,$ the adjustments don't
make much difference. The corresponding result for $lambda$ is
$(1.618, 1.945).$
There must be at least
half a dozen styles of Poisson CIs in use, each with enthusiastic adherents, and I don't claim mine is 'best'. Perhaps we will get some
recommendations from others on this site.
A simple simulation (in R) seems to show that this interval has about 95% actual coverage for
$lambda$ and $n$ of the sizes we have here.
set.seed(1201)
lam = 1.7733; n = 255
lam.tot = replicate(10^5, sum(rpois(n, lam)))
lcl = (lam.tot + 2 - 1.96*sqrt(lam.tot + 1))/n
ucl = (lam.tot + 2 + 1.96*sqrt(lam.tot + 1))/n
mean(lcl < lam & ucl > lam)
[1] 0.94997
$endgroup$
Here is an outline of the procedure you need to follow:
Goodness-of-fit to Poisson. Let the frequencies $f_i = 41, 62, 63, dots$
and the values be $k_i = 0, 1, 2, dots .$
Then estimate the population mean as
$hat lambda = frac 1 n sum_{i=1}^9 k_i f_i = 1.7733,$ where
$n = sum_{i=1}^9 f_i = 225.$
Then use observed category counts $X_j = 0, 1, 2, 3, 4,$ and $ge 5,$ where the last count is $7 = 1 + 0 + 1 = 9.$
Then according to the distribution $mathsf{Pois}(hat lambda = 1.7733),$ find the probabilities of each of
the categories, and multiply them by the sample size
$n$ to obtain expected counts $E_j.$
Contributions $C_j = frac {(X_j - E_j)^2}{E_j}$ to the chi-squared statistic
$Q = sum_j C_j = 2.096$ are shown in the table below along with the
$X_j$ and $E_j.$
X E C
41 38.198659 0.2054395
62 67.737681 0.4860070
63 60.059615 0.1439547
38 35.501239 0.1758758
12 15.738587 0.8880740
9 7.764219 0.1966912
Under the null hypothesis that data are Poisson,
the 'chi-squared' statistic $Q$ is approximately
distributed according to the distribution $mathsf{Chisq}(text{df} = 6-2 = 4).$ The null
hypothesis is not rejected at the 5% level because
the critical value for the test is $c = 9.488 > 2.096;$ you can find $c$
in printed tables of the chi-squared
distribution.
It was necessary to combine original counts 7, 1, 0, 1
into one category in order to have the last of the $E_j$'s exceed $5,$ assuring a reasonable fit of the
null distribution to chi-squared.
I have tried to be careful with my computations in this procedural outline, but
you should check them.
Confidence interval for Poisson mean. I am not sure what style of confidence interval you are
using for the Poisson mean $lambda$ based on the
estimate $hat lambda;$ I don't know what you mean
by 'of at least 0.94', I don't see the rationale for your value of $k,$ and I can't follow all of your computer code.
Also, I'm suspicious of the validity of a CI for $lambda > 0$ with
a lower endpoint that strays so far into negative
territory.
A Wald 95% CI for $nlambda$ is of the form $nhatlambda pm 1.96sqrt{nhatlambda}.$ Divide the endpoints by $n$ to get a 95% CI $(1.610, 1.937)$
for $lambda.$ [This is an asymptotic interval and
so it should be reasonably accurate for a
sample of size as large as $n = 255.]$
It has been suggested that a more accurate CI for $nlambda$ is of the form
$nhatlambda + 2 pm 1.96sqrt{nhatlambda + 1},$ but for $n = 255,$ the adjustments don't
make much difference. The corresponding result for $lambda$ is
$(1.618, 1.945).$
There must be at least
half a dozen styles of Poisson CIs in use, each with enthusiastic adherents, and I don't claim mine is 'best'. Perhaps we will get some
recommendations from others on this site.
A simple simulation (in R) seems to show that this interval has about 95% actual coverage for
$lambda$ and $n$ of the sizes we have here.
set.seed(1201)
lam = 1.7733; n = 255
lam.tot = replicate(10^5, sum(rpois(n, lam)))
lcl = (lam.tot + 2 - 1.96*sqrt(lam.tot + 1))/n
ucl = (lam.tot + 2 + 1.96*sqrt(lam.tot + 1))/n
mean(lcl < lam & ucl > lam)
[1] 0.94997
edited Dec 2 '18 at 4:32
answered Dec 2 '18 at 1:17
BruceETBruceET
35.6k71440
35.6k71440
$begingroup$
First, thank you for responding. I was calculating things thanks to his explanation and I have obtained something different. In the first part I get a statistic of 2.27488 degrees of freedom (6-1) = 5 and p-value = 0.809949, so I do not reject a null hypothesis for 0.05. In the second question, an unbiased estimator is the sample mean, that is, 1.7733 since their expectations coincide. In question three, they ask me for an IC <level at least>, so I have calculated it with X - + (sigma / sqrt (n alpha)) and I get [1.40265, 2.143959] at 94%
$endgroup$
– Fernando
Dec 2 '18 at 7:49
$begingroup$
I have edited the question with a continuation of the exercise that I do not know if it is well done and I would appreciate if you could tell me if it is well done or not
$endgroup$
– Fernando
Dec 2 '18 at 8:02
$begingroup$
Don't understand how you got 2.275. What is it? What formula? // DF = 6-2 = 4. Ordinarily, DF is one less than number of categories, but you lose one DF for estimating $lambda$ by $hatlambda.$ // If you're testing $H_0: lambda = 1.6$ against $H_a: lambda ne 1.6$ then you'd reject at 6% level if 1.6 is outside 94% CI. // Not sure how you got CI in your comment. Strange you're using 94% CI, usual confidence level is 95%. 94% CI should be shorter than 95% CI. Are you confusing $alpha$ and $sigma?$
$endgroup$
– BruceET
Dec 2 '18 at 9:45
$begingroup$
I am using a program to do the calculations, apart from doing it by hand. DF is always one less than the categories but in this case 2 are lost by the estimation and the categories?
$endgroup$
– Fernando
Dec 2 '18 at 12:12
$begingroup$
I Edit the question and I put a number to each question.// In 3, I think that the calculated is at an approximate level, but in the statement puts <at least level>, so use the formula that I put in the previous comment X - + (σ/ sqrt (n * α )) . (being X the average) // In 4, I think the result is what was obtained in section 3 and here it is <a approximate level>. // In 5, I have doubts if what they ask me is unilateral right or left unilateral
$endgroup$
– Fernando
Dec 2 '18 at 12:18
add a comment |
$begingroup$
First, thank you for responding. I was calculating things thanks to his explanation and I have obtained something different. In the first part I get a statistic of 2.27488 degrees of freedom (6-1) = 5 and p-value = 0.809949, so I do not reject a null hypothesis for 0.05. In the second question, an unbiased estimator is the sample mean, that is, 1.7733 since their expectations coincide. In question three, they ask me for an IC <level at least>, so I have calculated it with X - + (sigma / sqrt (n alpha)) and I get [1.40265, 2.143959] at 94%
$endgroup$
– Fernando
Dec 2 '18 at 7:49
$begingroup$
I have edited the question with a continuation of the exercise that I do not know if it is well done and I would appreciate if you could tell me if it is well done or not
$endgroup$
– Fernando
Dec 2 '18 at 8:02
$begingroup$
Don't understand how you got 2.275. What is it? What formula? // DF = 6-2 = 4. Ordinarily, DF is one less than number of categories, but you lose one DF for estimating $lambda$ by $hatlambda.$ // If you're testing $H_0: lambda = 1.6$ against $H_a: lambda ne 1.6$ then you'd reject at 6% level if 1.6 is outside 94% CI. // Not sure how you got CI in your comment. Strange you're using 94% CI, usual confidence level is 95%. 94% CI should be shorter than 95% CI. Are you confusing $alpha$ and $sigma?$
$endgroup$
– BruceET
Dec 2 '18 at 9:45
$begingroup$
I am using a program to do the calculations, apart from doing it by hand. DF is always one less than the categories but in this case 2 are lost by the estimation and the categories?
$endgroup$
– Fernando
Dec 2 '18 at 12:12
$begingroup$
I Edit the question and I put a number to each question.// In 3, I think that the calculated is at an approximate level, but in the statement puts <at least level>, so use the formula that I put in the previous comment X - + (σ/ sqrt (n * α )) . (being X the average) // In 4, I think the result is what was obtained in section 3 and here it is <a approximate level>. // In 5, I have doubts if what they ask me is unilateral right or left unilateral
$endgroup$
– Fernando
Dec 2 '18 at 12:18
$begingroup$
First, thank you for responding. I was calculating things thanks to his explanation and I have obtained something different. In the first part I get a statistic of 2.27488 degrees of freedom (6-1) = 5 and p-value = 0.809949, so I do not reject a null hypothesis for 0.05. In the second question, an unbiased estimator is the sample mean, that is, 1.7733 since their expectations coincide. In question three, they ask me for an IC <level at least>, so I have calculated it with X - + (sigma / sqrt (n alpha)) and I get [1.40265, 2.143959] at 94%
$endgroup$
– Fernando
Dec 2 '18 at 7:49
$begingroup$
First, thank you for responding. I was calculating things thanks to his explanation and I have obtained something different. In the first part I get a statistic of 2.27488 degrees of freedom (6-1) = 5 and p-value = 0.809949, so I do not reject a null hypothesis for 0.05. In the second question, an unbiased estimator is the sample mean, that is, 1.7733 since their expectations coincide. In question three, they ask me for an IC <level at least>, so I have calculated it with X - + (sigma / sqrt (n alpha)) and I get [1.40265, 2.143959] at 94%
$endgroup$
– Fernando
Dec 2 '18 at 7:49
$begingroup$
I have edited the question with a continuation of the exercise that I do not know if it is well done and I would appreciate if you could tell me if it is well done or not
$endgroup$
– Fernando
Dec 2 '18 at 8:02
$begingroup$
I have edited the question with a continuation of the exercise that I do not know if it is well done and I would appreciate if you could tell me if it is well done or not
$endgroup$
– Fernando
Dec 2 '18 at 8:02
$begingroup$
Don't understand how you got 2.275. What is it? What formula? // DF = 6-2 = 4. Ordinarily, DF is one less than number of categories, but you lose one DF for estimating $lambda$ by $hatlambda.$ // If you're testing $H_0: lambda = 1.6$ against $H_a: lambda ne 1.6$ then you'd reject at 6% level if 1.6 is outside 94% CI. // Not sure how you got CI in your comment. Strange you're using 94% CI, usual confidence level is 95%. 94% CI should be shorter than 95% CI. Are you confusing $alpha$ and $sigma?$
$endgroup$
– BruceET
Dec 2 '18 at 9:45
$begingroup$
Don't understand how you got 2.275. What is it? What formula? // DF = 6-2 = 4. Ordinarily, DF is one less than number of categories, but you lose one DF for estimating $lambda$ by $hatlambda.$ // If you're testing $H_0: lambda = 1.6$ against $H_a: lambda ne 1.6$ then you'd reject at 6% level if 1.6 is outside 94% CI. // Not sure how you got CI in your comment. Strange you're using 94% CI, usual confidence level is 95%. 94% CI should be shorter than 95% CI. Are you confusing $alpha$ and $sigma?$
$endgroup$
– BruceET
Dec 2 '18 at 9:45
$begingroup$
I am using a program to do the calculations, apart from doing it by hand. DF is always one less than the categories but in this case 2 are lost by the estimation and the categories?
$endgroup$
– Fernando
Dec 2 '18 at 12:12
$begingroup$
I am using a program to do the calculations, apart from doing it by hand. DF is always one less than the categories but in this case 2 are lost by the estimation and the categories?
$endgroup$
– Fernando
Dec 2 '18 at 12:12
$begingroup$
I Edit the question and I put a number to each question.// In 3, I think that the calculated is at an approximate level, but in the statement puts <at least level>, so use the formula that I put in the previous comment X - + (σ/ sqrt (n * α )) . (being X the average) // In 4, I think the result is what was obtained in section 3 and here it is <a approximate level>. // In 5, I have doubts if what they ask me is unilateral right or left unilateral
$endgroup$
– Fernando
Dec 2 '18 at 12:18
$begingroup$
I Edit the question and I put a number to each question.// In 3, I think that the calculated is at an approximate level, but in the statement puts <at least level>, so use the formula that I put in the previous comment X - + (σ/ sqrt (n * α )) . (being X the average) // In 4, I think the result is what was obtained in section 3 and here it is <a approximate level>. // In 5, I have doubts if what they ask me is unilateral right or left unilateral
$endgroup$
– Fernando
Dec 2 '18 at 12:18
add a comment |
Thanks for contributing an answer to Mathematics Stack Exchange!
- Please be sure to answer the question. Provide details and share your research!
But avoid …
- Asking for help, clarification, or responding to other answers.
- Making statements based on opinion; back them up with references or personal experience.
Use MathJax to format equations. MathJax reference.
To learn more, see our tips on writing great answers.
Sign up or log in
StackExchange.ready(function () {
StackExchange.helpers.onClickDraftSave('#login-link');
});
Sign up using Google
Sign up using Facebook
Sign up using Email and Password
Post as a guest
Required, but never shown
StackExchange.ready(
function () {
StackExchange.openid.initPostLogin('.new-post-login', 'https%3a%2f%2fmath.stackexchange.com%2fquestions%2f3018972%2fproblem-of-statistical-inference-poisson%23new-answer', 'question_page');
}
);
Post as a guest
Required, but never shown
Sign up or log in
StackExchange.ready(function () {
StackExchange.helpers.onClickDraftSave('#login-link');
});
Sign up using Google
Sign up using Facebook
Sign up using Email and Password
Post as a guest
Required, but never shown
Sign up or log in
StackExchange.ready(function () {
StackExchange.helpers.onClickDraftSave('#login-link');
});
Sign up using Google
Sign up using Facebook
Sign up using Email and Password
Post as a guest
Required, but never shown
Sign up or log in
StackExchange.ready(function () {
StackExchange.helpers.onClickDraftSave('#login-link');
});
Sign up using Google
Sign up using Facebook
Sign up using Email and Password
Sign up using Google
Sign up using Facebook
Sign up using Email and Password
Post as a guest
Required, but never shown
Required, but never shown
Required, but never shown
Required, but never shown
Required, but never shown
Required, but never shown
Required, but never shown
Required, but never shown
Required, but never shown
l,9sxvKTvXqDeF,ktVJ6 7B Dm0 jGmyFBUzmq2eruM7c C0IrNmiKW3gv5mV5yS6s9cef0,iLkq4,VUKKZrK6uUQpM FZ8,zXC,SvtqH