How do I create a new column in pandas from the difference of two string columns?
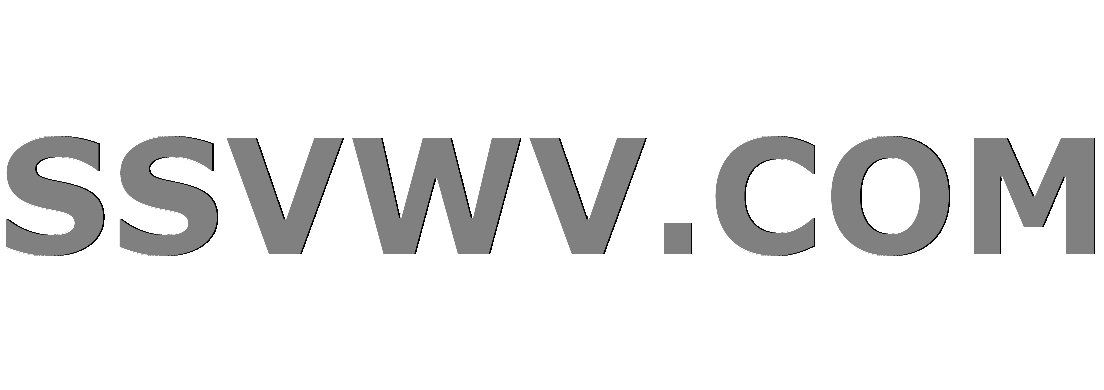
Multi tool use
up vote
2
down vote
favorite
How can I create a new column in pandas that is the result of the difference of two other columns consisting of strings?
I have one column titled "Good_Address" which has entries like "123 Fake Street Apt 101" and another column titled "Bad_Address" which has entries like "123 Fake Street". I want the output in column "Address_Difference" to be " Apt101".
I've tried doing:
import pandas as pd
data = pd.read_csv("AddressFile.csv")
data['Address Difference'] = data['GOOD_ADR1'].replace(data['BAD_ADR1'],'')
data['Address Difference']
but this does not work. It seems that the result is just equal to "123 Fake Street Apt101" (good address in the example above).
I've also tried:
data['Address Difference'] = data['GOOD_ADR1'].str.replace(data['BAD_ADR1'],'')
but this yields an error saying 'Series' objects are mutable, thus they cannot be hashed.
Any help would be appreciated.
Thanks
python regex pandas
add a comment |
up vote
2
down vote
favorite
How can I create a new column in pandas that is the result of the difference of two other columns consisting of strings?
I have one column titled "Good_Address" which has entries like "123 Fake Street Apt 101" and another column titled "Bad_Address" which has entries like "123 Fake Street". I want the output in column "Address_Difference" to be " Apt101".
I've tried doing:
import pandas as pd
data = pd.read_csv("AddressFile.csv")
data['Address Difference'] = data['GOOD_ADR1'].replace(data['BAD_ADR1'],'')
data['Address Difference']
but this does not work. It seems that the result is just equal to "123 Fake Street Apt101" (good address in the example above).
I've also tried:
data['Address Difference'] = data['GOOD_ADR1'].str.replace(data['BAD_ADR1'],'')
but this yields an error saying 'Series' objects are mutable, thus they cannot be hashed.
Any help would be appreciated.
Thanks
python regex pandas
add a comment |
up vote
2
down vote
favorite
up vote
2
down vote
favorite
How can I create a new column in pandas that is the result of the difference of two other columns consisting of strings?
I have one column titled "Good_Address" which has entries like "123 Fake Street Apt 101" and another column titled "Bad_Address" which has entries like "123 Fake Street". I want the output in column "Address_Difference" to be " Apt101".
I've tried doing:
import pandas as pd
data = pd.read_csv("AddressFile.csv")
data['Address Difference'] = data['GOOD_ADR1'].replace(data['BAD_ADR1'],'')
data['Address Difference']
but this does not work. It seems that the result is just equal to "123 Fake Street Apt101" (good address in the example above).
I've also tried:
data['Address Difference'] = data['GOOD_ADR1'].str.replace(data['BAD_ADR1'],'')
but this yields an error saying 'Series' objects are mutable, thus they cannot be hashed.
Any help would be appreciated.
Thanks
python regex pandas
How can I create a new column in pandas that is the result of the difference of two other columns consisting of strings?
I have one column titled "Good_Address" which has entries like "123 Fake Street Apt 101" and another column titled "Bad_Address" which has entries like "123 Fake Street". I want the output in column "Address_Difference" to be " Apt101".
I've tried doing:
import pandas as pd
data = pd.read_csv("AddressFile.csv")
data['Address Difference'] = data['GOOD_ADR1'].replace(data['BAD_ADR1'],'')
data['Address Difference']
but this does not work. It seems that the result is just equal to "123 Fake Street Apt101" (good address in the example above).
I've also tried:
data['Address Difference'] = data['GOOD_ADR1'].str.replace(data['BAD_ADR1'],'')
but this yields an error saying 'Series' objects are mutable, thus they cannot be hashed.
Any help would be appreciated.
Thanks
python regex pandas
python regex pandas
edited Nov 16 at 19:30


Vaishali
16.8k3927
16.8k3927
asked Nov 13 at 20:19
L. Taylor
112
112
add a comment |
add a comment |
3 Answers
3
active
oldest
votes
up vote
3
down vote
Using replace
with regex
data['Address Difference']=data['GOOD_ADR1'].replace(regex=r'(?i)'+ data['BAD_ADR1'],value="")
Seems to work pretty well in most cases. Let me append a question to this: if I see a good address like 123 N Main St Apt 101 and a bad address like 123 North Main St, why does this code return a value equal to the good address (i.e. 123 N Main St Apt 101)?
– L. Taylor
Nov 13 at 20:39
@L.Taylor since not match , it will return what you have in the good address column, pandas treat it as one string '123 N Main St Apt 101'
– W-B
Nov 13 at 20:41
add a comment |
up vote
2
down vote
I'd use a function that we can map across inputs. This should be fast.
The function will use str.find
to see if the other string is a subset. If the result of str.find
is -1
then the substring could not be found. Otherwise, extricate the substring given the position it was found and the length of the substring.
def rm(x, y):
i = x.find(y)
if i > -1:
j = len(y)
return x[:i] + x[i+j:]
else:
return x
df['Address Difference'] = [*map(rm, df.GOOD_ADR1, df.BAD_ADR1)]
df
BAD_ADR1 GOOD_ADR1 Address Difference
0 123 Fake Street 123 Fake Street Apt 101 Apt 101
Very cool, I guess this would be very expensive computationaly speaking?
– Datanovice
Nov 13 at 20:28
1
No. You should try it. In fact, I clock it at 1000 times faster on a dataframe of length 10,000
– piRSquared
Nov 13 at 20:29
1
Will do! Thanks sir will add this to my code base for reference!
– Datanovice
Nov 13 at 21:28
add a comment |
up vote
1
down vote
You can replace the bad address part from good address
df['Address_Difference'] = df['Good_Address'].replace(df['Bad_Address'], '', regex = True).str.strip()
Bad_Address Good_Address Address_Difference
0 123 Fake Street 123 Fake Street Apt 101 Apt 101
add a comment |
3 Answers
3
active
oldest
votes
3 Answers
3
active
oldest
votes
active
oldest
votes
active
oldest
votes
up vote
3
down vote
Using replace
with regex
data['Address Difference']=data['GOOD_ADR1'].replace(regex=r'(?i)'+ data['BAD_ADR1'],value="")
Seems to work pretty well in most cases. Let me append a question to this: if I see a good address like 123 N Main St Apt 101 and a bad address like 123 North Main St, why does this code return a value equal to the good address (i.e. 123 N Main St Apt 101)?
– L. Taylor
Nov 13 at 20:39
@L.Taylor since not match , it will return what you have in the good address column, pandas treat it as one string '123 N Main St Apt 101'
– W-B
Nov 13 at 20:41
add a comment |
up vote
3
down vote
Using replace
with regex
data['Address Difference']=data['GOOD_ADR1'].replace(regex=r'(?i)'+ data['BAD_ADR1'],value="")
Seems to work pretty well in most cases. Let me append a question to this: if I see a good address like 123 N Main St Apt 101 and a bad address like 123 North Main St, why does this code return a value equal to the good address (i.e. 123 N Main St Apt 101)?
– L. Taylor
Nov 13 at 20:39
@L.Taylor since not match , it will return what you have in the good address column, pandas treat it as one string '123 N Main St Apt 101'
– W-B
Nov 13 at 20:41
add a comment |
up vote
3
down vote
up vote
3
down vote
Using replace
with regex
data['Address Difference']=data['GOOD_ADR1'].replace(regex=r'(?i)'+ data['BAD_ADR1'],value="")
Using replace
with regex
data['Address Difference']=data['GOOD_ADR1'].replace(regex=r'(?i)'+ data['BAD_ADR1'],value="")
answered Nov 13 at 20:25


W-B
95.2k72860
95.2k72860
Seems to work pretty well in most cases. Let me append a question to this: if I see a good address like 123 N Main St Apt 101 and a bad address like 123 North Main St, why does this code return a value equal to the good address (i.e. 123 N Main St Apt 101)?
– L. Taylor
Nov 13 at 20:39
@L.Taylor since not match , it will return what you have in the good address column, pandas treat it as one string '123 N Main St Apt 101'
– W-B
Nov 13 at 20:41
add a comment |
Seems to work pretty well in most cases. Let me append a question to this: if I see a good address like 123 N Main St Apt 101 and a bad address like 123 North Main St, why does this code return a value equal to the good address (i.e. 123 N Main St Apt 101)?
– L. Taylor
Nov 13 at 20:39
@L.Taylor since not match , it will return what you have in the good address column, pandas treat it as one string '123 N Main St Apt 101'
– W-B
Nov 13 at 20:41
Seems to work pretty well in most cases. Let me append a question to this: if I see a good address like 123 N Main St Apt 101 and a bad address like 123 North Main St, why does this code return a value equal to the good address (i.e. 123 N Main St Apt 101)?
– L. Taylor
Nov 13 at 20:39
Seems to work pretty well in most cases. Let me append a question to this: if I see a good address like 123 N Main St Apt 101 and a bad address like 123 North Main St, why does this code return a value equal to the good address (i.e. 123 N Main St Apt 101)?
– L. Taylor
Nov 13 at 20:39
@L.Taylor since not match , it will return what you have in the good address column, pandas treat it as one string '123 N Main St Apt 101'
– W-B
Nov 13 at 20:41
@L.Taylor since not match , it will return what you have in the good address column, pandas treat it as one string '123 N Main St Apt 101'
– W-B
Nov 13 at 20:41
add a comment |
up vote
2
down vote
I'd use a function that we can map across inputs. This should be fast.
The function will use str.find
to see if the other string is a subset. If the result of str.find
is -1
then the substring could not be found. Otherwise, extricate the substring given the position it was found and the length of the substring.
def rm(x, y):
i = x.find(y)
if i > -1:
j = len(y)
return x[:i] + x[i+j:]
else:
return x
df['Address Difference'] = [*map(rm, df.GOOD_ADR1, df.BAD_ADR1)]
df
BAD_ADR1 GOOD_ADR1 Address Difference
0 123 Fake Street 123 Fake Street Apt 101 Apt 101
Very cool, I guess this would be very expensive computationaly speaking?
– Datanovice
Nov 13 at 20:28
1
No. You should try it. In fact, I clock it at 1000 times faster on a dataframe of length 10,000
– piRSquared
Nov 13 at 20:29
1
Will do! Thanks sir will add this to my code base for reference!
– Datanovice
Nov 13 at 21:28
add a comment |
up vote
2
down vote
I'd use a function that we can map across inputs. This should be fast.
The function will use str.find
to see if the other string is a subset. If the result of str.find
is -1
then the substring could not be found. Otherwise, extricate the substring given the position it was found and the length of the substring.
def rm(x, y):
i = x.find(y)
if i > -1:
j = len(y)
return x[:i] + x[i+j:]
else:
return x
df['Address Difference'] = [*map(rm, df.GOOD_ADR1, df.BAD_ADR1)]
df
BAD_ADR1 GOOD_ADR1 Address Difference
0 123 Fake Street 123 Fake Street Apt 101 Apt 101
Very cool, I guess this would be very expensive computationaly speaking?
– Datanovice
Nov 13 at 20:28
1
No. You should try it. In fact, I clock it at 1000 times faster on a dataframe of length 10,000
– piRSquared
Nov 13 at 20:29
1
Will do! Thanks sir will add this to my code base for reference!
– Datanovice
Nov 13 at 21:28
add a comment |
up vote
2
down vote
up vote
2
down vote
I'd use a function that we can map across inputs. This should be fast.
The function will use str.find
to see if the other string is a subset. If the result of str.find
is -1
then the substring could not be found. Otherwise, extricate the substring given the position it was found and the length of the substring.
def rm(x, y):
i = x.find(y)
if i > -1:
j = len(y)
return x[:i] + x[i+j:]
else:
return x
df['Address Difference'] = [*map(rm, df.GOOD_ADR1, df.BAD_ADR1)]
df
BAD_ADR1 GOOD_ADR1 Address Difference
0 123 Fake Street 123 Fake Street Apt 101 Apt 101
I'd use a function that we can map across inputs. This should be fast.
The function will use str.find
to see if the other string is a subset. If the result of str.find
is -1
then the substring could not be found. Otherwise, extricate the substring given the position it was found and the length of the substring.
def rm(x, y):
i = x.find(y)
if i > -1:
j = len(y)
return x[:i] + x[i+j:]
else:
return x
df['Address Difference'] = [*map(rm, df.GOOD_ADR1, df.BAD_ADR1)]
df
BAD_ADR1 GOOD_ADR1 Address Difference
0 123 Fake Street 123 Fake Street Apt 101 Apt 101
edited Nov 13 at 20:41
answered Nov 13 at 20:26


piRSquared
150k21135277
150k21135277
Very cool, I guess this would be very expensive computationaly speaking?
– Datanovice
Nov 13 at 20:28
1
No. You should try it. In fact, I clock it at 1000 times faster on a dataframe of length 10,000
– piRSquared
Nov 13 at 20:29
1
Will do! Thanks sir will add this to my code base for reference!
– Datanovice
Nov 13 at 21:28
add a comment |
Very cool, I guess this would be very expensive computationaly speaking?
– Datanovice
Nov 13 at 20:28
1
No. You should try it. In fact, I clock it at 1000 times faster on a dataframe of length 10,000
– piRSquared
Nov 13 at 20:29
1
Will do! Thanks sir will add this to my code base for reference!
– Datanovice
Nov 13 at 21:28
Very cool, I guess this would be very expensive computationaly speaking?
– Datanovice
Nov 13 at 20:28
Very cool, I guess this would be very expensive computationaly speaking?
– Datanovice
Nov 13 at 20:28
1
1
No. You should try it. In fact, I clock it at 1000 times faster on a dataframe of length 10,000
– piRSquared
Nov 13 at 20:29
No. You should try it. In fact, I clock it at 1000 times faster on a dataframe of length 10,000
– piRSquared
Nov 13 at 20:29
1
1
Will do! Thanks sir will add this to my code base for reference!
– Datanovice
Nov 13 at 21:28
Will do! Thanks sir will add this to my code base for reference!
– Datanovice
Nov 13 at 21:28
add a comment |
up vote
1
down vote
You can replace the bad address part from good address
df['Address_Difference'] = df['Good_Address'].replace(df['Bad_Address'], '', regex = True).str.strip()
Bad_Address Good_Address Address_Difference
0 123 Fake Street 123 Fake Street Apt 101 Apt 101
add a comment |
up vote
1
down vote
You can replace the bad address part from good address
df['Address_Difference'] = df['Good_Address'].replace(df['Bad_Address'], '', regex = True).str.strip()
Bad_Address Good_Address Address_Difference
0 123 Fake Street 123 Fake Street Apt 101 Apt 101
add a comment |
up vote
1
down vote
up vote
1
down vote
You can replace the bad address part from good address
df['Address_Difference'] = df['Good_Address'].replace(df['Bad_Address'], '', regex = True).str.strip()
Bad_Address Good_Address Address_Difference
0 123 Fake Street 123 Fake Street Apt 101 Apt 101
You can replace the bad address part from good address
df['Address_Difference'] = df['Good_Address'].replace(df['Bad_Address'], '', regex = True).str.strip()
Bad_Address Good_Address Address_Difference
0 123 Fake Street 123 Fake Street Apt 101 Apt 101
answered Nov 13 at 20:25


Vaishali
16.8k3927
16.8k3927
add a comment |
add a comment |
Thanks for contributing an answer to Stack Overflow!
- Please be sure to answer the question. Provide details and share your research!
But avoid …
- Asking for help, clarification, or responding to other answers.
- Making statements based on opinion; back them up with references or personal experience.
To learn more, see our tips on writing great answers.
Some of your past answers have not been well-received, and you're in danger of being blocked from answering.
Please pay close attention to the following guidance:
- Please be sure to answer the question. Provide details and share your research!
But avoid …
- Asking for help, clarification, or responding to other answers.
- Making statements based on opinion; back them up with references or personal experience.
To learn more, see our tips on writing great answers.
Sign up or log in
StackExchange.ready(function () {
StackExchange.helpers.onClickDraftSave('#login-link');
});
Sign up using Google
Sign up using Facebook
Sign up using Email and Password
Post as a guest
Required, but never shown
StackExchange.ready(
function () {
StackExchange.openid.initPostLogin('.new-post-login', 'https%3a%2f%2fstackoverflow.com%2fquestions%2f53288887%2fhow-do-i-create-a-new-column-in-pandas-from-the-difference-of-two-string-columns%23new-answer', 'question_page');
}
);
Post as a guest
Required, but never shown
Sign up or log in
StackExchange.ready(function () {
StackExchange.helpers.onClickDraftSave('#login-link');
});
Sign up using Google
Sign up using Facebook
Sign up using Email and Password
Post as a guest
Required, but never shown
Sign up or log in
StackExchange.ready(function () {
StackExchange.helpers.onClickDraftSave('#login-link');
});
Sign up using Google
Sign up using Facebook
Sign up using Email and Password
Post as a guest
Required, but never shown
Sign up or log in
StackExchange.ready(function () {
StackExchange.helpers.onClickDraftSave('#login-link');
});
Sign up using Google
Sign up using Facebook
Sign up using Email and Password
Sign up using Google
Sign up using Facebook
Sign up using Email and Password
Post as a guest
Required, but never shown
Required, but never shown
Required, but never shown
Required, but never shown
Required, but never shown
Required, but never shown
Required, but never shown
Required, but never shown
Required, but never shown
oFlhT2DolKa jl71MXF,qASOI kayuHx8AUQJiadmdnGv2nkDMWaYLOt L