Check failed: K_ == new_K (6656 vs. 4096) Input size incompatible with inner product parameters
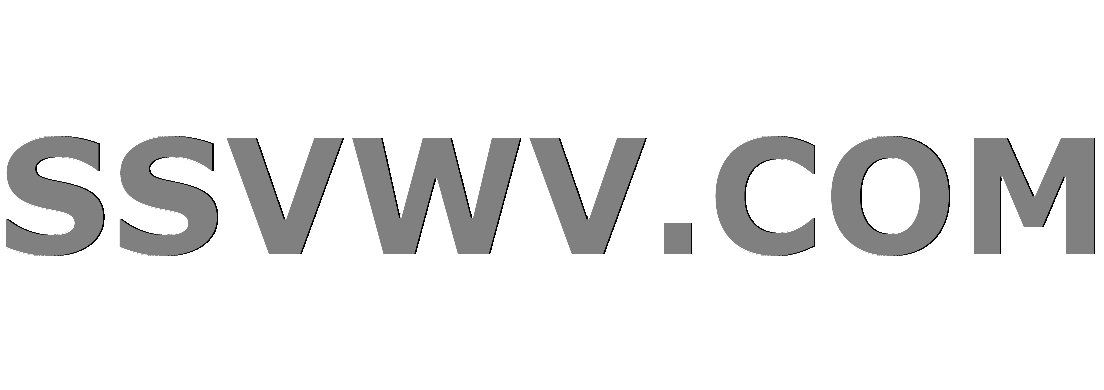
Multi tool use
There is another similar question here. But that is not duplicate to my question as they are more on input dimension mismatch from network's requirement.
For me is I use customized network but input dimension is still the same.
I am not using any per-trained network as it is my own network.
I have problem with training on customized network with py-faster-rcnn method.
The original VGG16 NET has the following RCNN network.
layer {
name: "roi_pool5"
type: "ROIPooling"
bottom: "conv5_3"
bottom: "rois"
top: "pool5"
roi_pooling_param {
pooled_w: 7
pooled_h: 7
spatial_scale: 0.0625 # 1/16
}
}
layer {
name: "fc6"
type: "InnerProduct"
bottom: "pool5"
top: "fc6"
param {
lr_mult: 1
}
param {
lr_mult: 2
}
inner_product_param {
num_output: 4096
}
}
layer {
name: "relu6"
type: "ReLU"
bottom: "fc6"
top: "fc6"
}
layer {
name: "drop6"
type: "Dropout"
bottom: "fc6"
top: "fc6"
dropout_param {
dropout_ratio: 0.5
}
}
layer {
name: "fc7"
type: "InnerProduct"
bottom: "fc6"
top: "fc7"
param {
lr_mult: 1
}
param {
lr_mult: 2
}
inner_product_param {
num_output: 4096
}
}
layer {
name: "relu7"
type: "ReLU"
bottom: "fc7"
top: "fc7"
}
layer {
name: "drop7"
type: "Dropout"
bottom: "fc7"
top: "fc7"
dropout_param {
dropout_ratio: 0.5
}
}
layer {
name: "cls_score"
type: "InnerProduct"
bottom: "fc7"
top: "cls_score"
param {
lr_mult: 1
}
param {
lr_mult: 2
}
inner_product_param {
num_output: 4
weight_filler {
type: "gaussian"
std: 0.01
}
bias_filler {
type: "constant"
value: 0
}
}
}
layer {
name: "bbox_pred"
type: "InnerProduct"
bottom: "fc7"
top: "bbox_pred"
param {
lr_mult: 1
}
param {
lr_mult: 2
}
inner_product_param {
num_output: 16
weight_filler {
type: "gaussian"
std: 0.001
}
bias_filler {
type: "constant"
value: 0
}
}
}
layer {
name: "loss_cls"
type: "SoftmaxWithLoss"
bottom: "cls_score"
bottom: "labels"
propagate_down: 1
propagate_down: 0
top: "loss_cls"
loss_weight: 1
}
layer {
name: "loss_bbox"
type: "SmoothL1Loss"
bottom: "bbox_pred"
bottom: "bbox_targets"
bottom: "bbox_inside_weights"
bottom: "bbox_outside_weights"
top: "loss_bbox"
loss_weight: 1
}
Which has roi_pool5 fed into inner product layer with output channels 4096.
roi_pool5 has input conv5_3 with 512 channels.
For me, my RCNN network has Inception layers with 64 channels. Then fed to innerproduct layer with output 512 channels.
According to discussion here, innerproduct layer is designed to accept fixed size of input. What does it mean to fixed size of input?
Where should I check to meet innerproduct layer's fixed size requirement?
My RCNN network is shown here.
tensorflow deep-learning caffe
add a comment |
There is another similar question here. But that is not duplicate to my question as they are more on input dimension mismatch from network's requirement.
For me is I use customized network but input dimension is still the same.
I am not using any per-trained network as it is my own network.
I have problem with training on customized network with py-faster-rcnn method.
The original VGG16 NET has the following RCNN network.
layer {
name: "roi_pool5"
type: "ROIPooling"
bottom: "conv5_3"
bottom: "rois"
top: "pool5"
roi_pooling_param {
pooled_w: 7
pooled_h: 7
spatial_scale: 0.0625 # 1/16
}
}
layer {
name: "fc6"
type: "InnerProduct"
bottom: "pool5"
top: "fc6"
param {
lr_mult: 1
}
param {
lr_mult: 2
}
inner_product_param {
num_output: 4096
}
}
layer {
name: "relu6"
type: "ReLU"
bottom: "fc6"
top: "fc6"
}
layer {
name: "drop6"
type: "Dropout"
bottom: "fc6"
top: "fc6"
dropout_param {
dropout_ratio: 0.5
}
}
layer {
name: "fc7"
type: "InnerProduct"
bottom: "fc6"
top: "fc7"
param {
lr_mult: 1
}
param {
lr_mult: 2
}
inner_product_param {
num_output: 4096
}
}
layer {
name: "relu7"
type: "ReLU"
bottom: "fc7"
top: "fc7"
}
layer {
name: "drop7"
type: "Dropout"
bottom: "fc7"
top: "fc7"
dropout_param {
dropout_ratio: 0.5
}
}
layer {
name: "cls_score"
type: "InnerProduct"
bottom: "fc7"
top: "cls_score"
param {
lr_mult: 1
}
param {
lr_mult: 2
}
inner_product_param {
num_output: 4
weight_filler {
type: "gaussian"
std: 0.01
}
bias_filler {
type: "constant"
value: 0
}
}
}
layer {
name: "bbox_pred"
type: "InnerProduct"
bottom: "fc7"
top: "bbox_pred"
param {
lr_mult: 1
}
param {
lr_mult: 2
}
inner_product_param {
num_output: 16
weight_filler {
type: "gaussian"
std: 0.001
}
bias_filler {
type: "constant"
value: 0
}
}
}
layer {
name: "loss_cls"
type: "SoftmaxWithLoss"
bottom: "cls_score"
bottom: "labels"
propagate_down: 1
propagate_down: 0
top: "loss_cls"
loss_weight: 1
}
layer {
name: "loss_bbox"
type: "SmoothL1Loss"
bottom: "bbox_pred"
bottom: "bbox_targets"
bottom: "bbox_inside_weights"
bottom: "bbox_outside_weights"
top: "loss_bbox"
loss_weight: 1
}
Which has roi_pool5 fed into inner product layer with output channels 4096.
roi_pool5 has input conv5_3 with 512 channels.
For me, my RCNN network has Inception layers with 64 channels. Then fed to innerproduct layer with output 512 channels.
According to discussion here, innerproduct layer is designed to accept fixed size of input. What does it mean to fixed size of input?
Where should I check to meet innerproduct layer's fixed size requirement?
My RCNN network is shown here.
tensorflow deep-learning caffe
add a comment |
There is another similar question here. But that is not duplicate to my question as they are more on input dimension mismatch from network's requirement.
For me is I use customized network but input dimension is still the same.
I am not using any per-trained network as it is my own network.
I have problem with training on customized network with py-faster-rcnn method.
The original VGG16 NET has the following RCNN network.
layer {
name: "roi_pool5"
type: "ROIPooling"
bottom: "conv5_3"
bottom: "rois"
top: "pool5"
roi_pooling_param {
pooled_w: 7
pooled_h: 7
spatial_scale: 0.0625 # 1/16
}
}
layer {
name: "fc6"
type: "InnerProduct"
bottom: "pool5"
top: "fc6"
param {
lr_mult: 1
}
param {
lr_mult: 2
}
inner_product_param {
num_output: 4096
}
}
layer {
name: "relu6"
type: "ReLU"
bottom: "fc6"
top: "fc6"
}
layer {
name: "drop6"
type: "Dropout"
bottom: "fc6"
top: "fc6"
dropout_param {
dropout_ratio: 0.5
}
}
layer {
name: "fc7"
type: "InnerProduct"
bottom: "fc6"
top: "fc7"
param {
lr_mult: 1
}
param {
lr_mult: 2
}
inner_product_param {
num_output: 4096
}
}
layer {
name: "relu7"
type: "ReLU"
bottom: "fc7"
top: "fc7"
}
layer {
name: "drop7"
type: "Dropout"
bottom: "fc7"
top: "fc7"
dropout_param {
dropout_ratio: 0.5
}
}
layer {
name: "cls_score"
type: "InnerProduct"
bottom: "fc7"
top: "cls_score"
param {
lr_mult: 1
}
param {
lr_mult: 2
}
inner_product_param {
num_output: 4
weight_filler {
type: "gaussian"
std: 0.01
}
bias_filler {
type: "constant"
value: 0
}
}
}
layer {
name: "bbox_pred"
type: "InnerProduct"
bottom: "fc7"
top: "bbox_pred"
param {
lr_mult: 1
}
param {
lr_mult: 2
}
inner_product_param {
num_output: 16
weight_filler {
type: "gaussian"
std: 0.001
}
bias_filler {
type: "constant"
value: 0
}
}
}
layer {
name: "loss_cls"
type: "SoftmaxWithLoss"
bottom: "cls_score"
bottom: "labels"
propagate_down: 1
propagate_down: 0
top: "loss_cls"
loss_weight: 1
}
layer {
name: "loss_bbox"
type: "SmoothL1Loss"
bottom: "bbox_pred"
bottom: "bbox_targets"
bottom: "bbox_inside_weights"
bottom: "bbox_outside_weights"
top: "loss_bbox"
loss_weight: 1
}
Which has roi_pool5 fed into inner product layer with output channels 4096.
roi_pool5 has input conv5_3 with 512 channels.
For me, my RCNN network has Inception layers with 64 channels. Then fed to innerproduct layer with output 512 channels.
According to discussion here, innerproduct layer is designed to accept fixed size of input. What does it mean to fixed size of input?
Where should I check to meet innerproduct layer's fixed size requirement?
My RCNN network is shown here.
tensorflow deep-learning caffe
There is another similar question here. But that is not duplicate to my question as they are more on input dimension mismatch from network's requirement.
For me is I use customized network but input dimension is still the same.
I am not using any per-trained network as it is my own network.
I have problem with training on customized network with py-faster-rcnn method.
The original VGG16 NET has the following RCNN network.
layer {
name: "roi_pool5"
type: "ROIPooling"
bottom: "conv5_3"
bottom: "rois"
top: "pool5"
roi_pooling_param {
pooled_w: 7
pooled_h: 7
spatial_scale: 0.0625 # 1/16
}
}
layer {
name: "fc6"
type: "InnerProduct"
bottom: "pool5"
top: "fc6"
param {
lr_mult: 1
}
param {
lr_mult: 2
}
inner_product_param {
num_output: 4096
}
}
layer {
name: "relu6"
type: "ReLU"
bottom: "fc6"
top: "fc6"
}
layer {
name: "drop6"
type: "Dropout"
bottom: "fc6"
top: "fc6"
dropout_param {
dropout_ratio: 0.5
}
}
layer {
name: "fc7"
type: "InnerProduct"
bottom: "fc6"
top: "fc7"
param {
lr_mult: 1
}
param {
lr_mult: 2
}
inner_product_param {
num_output: 4096
}
}
layer {
name: "relu7"
type: "ReLU"
bottom: "fc7"
top: "fc7"
}
layer {
name: "drop7"
type: "Dropout"
bottom: "fc7"
top: "fc7"
dropout_param {
dropout_ratio: 0.5
}
}
layer {
name: "cls_score"
type: "InnerProduct"
bottom: "fc7"
top: "cls_score"
param {
lr_mult: 1
}
param {
lr_mult: 2
}
inner_product_param {
num_output: 4
weight_filler {
type: "gaussian"
std: 0.01
}
bias_filler {
type: "constant"
value: 0
}
}
}
layer {
name: "bbox_pred"
type: "InnerProduct"
bottom: "fc7"
top: "bbox_pred"
param {
lr_mult: 1
}
param {
lr_mult: 2
}
inner_product_param {
num_output: 16
weight_filler {
type: "gaussian"
std: 0.001
}
bias_filler {
type: "constant"
value: 0
}
}
}
layer {
name: "loss_cls"
type: "SoftmaxWithLoss"
bottom: "cls_score"
bottom: "labels"
propagate_down: 1
propagate_down: 0
top: "loss_cls"
loss_weight: 1
}
layer {
name: "loss_bbox"
type: "SmoothL1Loss"
bottom: "bbox_pred"
bottom: "bbox_targets"
bottom: "bbox_inside_weights"
bottom: "bbox_outside_weights"
top: "loss_bbox"
loss_weight: 1
}
Which has roi_pool5 fed into inner product layer with output channels 4096.
roi_pool5 has input conv5_3 with 512 channels.
For me, my RCNN network has Inception layers with 64 channels. Then fed to innerproduct layer with output 512 channels.
According to discussion here, innerproduct layer is designed to accept fixed size of input. What does it mean to fixed size of input?
Where should I check to meet innerproduct layer's fixed size requirement?
My RCNN network is shown here.
tensorflow deep-learning caffe
tensorflow deep-learning caffe
edited Nov 22 '18 at 6:10
batuman
asked Nov 22 '18 at 6:02
batumanbatuman
2,585744111
2,585744111
add a comment |
add a comment |
0
active
oldest
votes
Your Answer
StackExchange.ifUsing("editor", function () {
StackExchange.using("externalEditor", function () {
StackExchange.using("snippets", function () {
StackExchange.snippets.init();
});
});
}, "code-snippets");
StackExchange.ready(function() {
var channelOptions = {
tags: "".split(" "),
id: "1"
};
initTagRenderer("".split(" "), "".split(" "), channelOptions);
StackExchange.using("externalEditor", function() {
// Have to fire editor after snippets, if snippets enabled
if (StackExchange.settings.snippets.snippetsEnabled) {
StackExchange.using("snippets", function() {
createEditor();
});
}
else {
createEditor();
}
});
function createEditor() {
StackExchange.prepareEditor({
heartbeatType: 'answer',
autoActivateHeartbeat: false,
convertImagesToLinks: true,
noModals: true,
showLowRepImageUploadWarning: true,
reputationToPostImages: 10,
bindNavPrevention: true,
postfix: "",
imageUploader: {
brandingHtml: "Powered by u003ca class="icon-imgur-white" href="https://imgur.com/"u003eu003c/au003e",
contentPolicyHtml: "User contributions licensed under u003ca href="https://creativecommons.org/licenses/by-sa/3.0/"u003ecc by-sa 3.0 with attribution requiredu003c/au003e u003ca href="https://stackoverflow.com/legal/content-policy"u003e(content policy)u003c/au003e",
allowUrls: true
},
onDemand: true,
discardSelector: ".discard-answer"
,immediatelyShowMarkdownHelp:true
});
}
});
Sign up or log in
StackExchange.ready(function () {
StackExchange.helpers.onClickDraftSave('#login-link');
});
Sign up using Google
Sign up using Facebook
Sign up using Email and Password
Post as a guest
Required, but never shown
StackExchange.ready(
function () {
StackExchange.openid.initPostLogin('.new-post-login', 'https%3a%2f%2fstackoverflow.com%2fquestions%2f53424755%2fcheck-failed-k-new-k-6656-vs-4096-input-size-incompatible-with-inner-pro%23new-answer', 'question_page');
}
);
Post as a guest
Required, but never shown
0
active
oldest
votes
0
active
oldest
votes
active
oldest
votes
active
oldest
votes
Thanks for contributing an answer to Stack Overflow!
- Please be sure to answer the question. Provide details and share your research!
But avoid …
- Asking for help, clarification, or responding to other answers.
- Making statements based on opinion; back them up with references or personal experience.
To learn more, see our tips on writing great answers.
Sign up or log in
StackExchange.ready(function () {
StackExchange.helpers.onClickDraftSave('#login-link');
});
Sign up using Google
Sign up using Facebook
Sign up using Email and Password
Post as a guest
Required, but never shown
StackExchange.ready(
function () {
StackExchange.openid.initPostLogin('.new-post-login', 'https%3a%2f%2fstackoverflow.com%2fquestions%2f53424755%2fcheck-failed-k-new-k-6656-vs-4096-input-size-incompatible-with-inner-pro%23new-answer', 'question_page');
}
);
Post as a guest
Required, but never shown
Sign up or log in
StackExchange.ready(function () {
StackExchange.helpers.onClickDraftSave('#login-link');
});
Sign up using Google
Sign up using Facebook
Sign up using Email and Password
Post as a guest
Required, but never shown
Sign up or log in
StackExchange.ready(function () {
StackExchange.helpers.onClickDraftSave('#login-link');
});
Sign up using Google
Sign up using Facebook
Sign up using Email and Password
Post as a guest
Required, but never shown
Sign up or log in
StackExchange.ready(function () {
StackExchange.helpers.onClickDraftSave('#login-link');
});
Sign up using Google
Sign up using Facebook
Sign up using Email and Password
Sign up using Google
Sign up using Facebook
Sign up using Email and Password
Post as a guest
Required, but never shown
Required, but never shown
Required, but never shown
Required, but never shown
Required, but never shown
Required, but never shown
Required, but never shown
Required, but never shown
Required, but never shown
1Laqb8goZ haUhXL,tAhWh,6 Wd3r8choh9kYpO9WH,daeDe,J9uGtO,nBrZ W 7pO4QS4bS5tZ3AlGI,ErabNwgDnHUQjh5HcP