Neural network to solve nonlinear constrained optimization problem
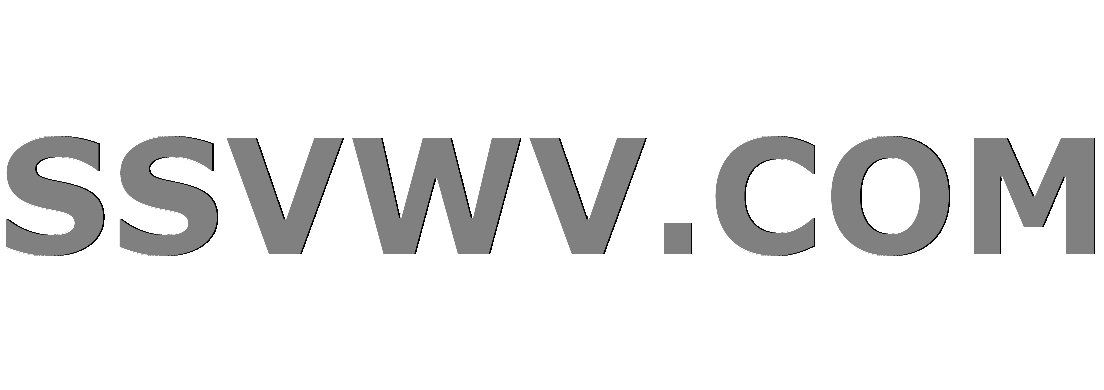
Multi tool use
$begingroup$
I'm trying to build a neural network to solve this optimization problem
minimize $f(x)$
s.t. $h(x)=0$
where $x =(x_1, x_2, dots, x_n)^T in R^n$, $f: R^n rightarrow R$ and $h: R^n rightarrow R^m$ are given functions and $m le n$. $f$ and $h$ are assumed to be twice continuous differentiable.
The idea is presented in this paper (IEEE abstract)
and consists in creating a neural network whose equilibrium point satisfies the necessary conditions of optimality.
Based on the Lagrange multiplier theory, the neural network will be governed by:
$frac{dx}{dt} = - nabla_x L(x, lambda) $
$frac{dlambda}{dt} = nabla_{lambda} L(x, lambda) $
where $L: R^{m+n} rightarrow R$ is the Lagrange function defined by: $L(x, lambda) = f(x) + lambda^T h(x)$, $lambda in R^m$
- I do not see how we could use the last equations to create a neural
network? - For a neural network, the output $(x^*, lambda^*)$ "must" be known,
also, how to implement forward propagation and backpropagation? - Could someone help me understand how to create such a network or, if
possible, provide a Matlab or Python code to implement the network?
Many thanks.
nonlinear-optimization neural-networks
$endgroup$
add a comment |
$begingroup$
I'm trying to build a neural network to solve this optimization problem
minimize $f(x)$
s.t. $h(x)=0$
where $x =(x_1, x_2, dots, x_n)^T in R^n$, $f: R^n rightarrow R$ and $h: R^n rightarrow R^m$ are given functions and $m le n$. $f$ and $h$ are assumed to be twice continuous differentiable.
The idea is presented in this paper (IEEE abstract)
and consists in creating a neural network whose equilibrium point satisfies the necessary conditions of optimality.
Based on the Lagrange multiplier theory, the neural network will be governed by:
$frac{dx}{dt} = - nabla_x L(x, lambda) $
$frac{dlambda}{dt} = nabla_{lambda} L(x, lambda) $
where $L: R^{m+n} rightarrow R$ is the Lagrange function defined by: $L(x, lambda) = f(x) + lambda^T h(x)$, $lambda in R^m$
- I do not see how we could use the last equations to create a neural
network? - For a neural network, the output $(x^*, lambda^*)$ "must" be known,
also, how to implement forward propagation and backpropagation? - Could someone help me understand how to create such a network or, if
possible, provide a Matlab or Python code to implement the network?
Many thanks.
nonlinear-optimization neural-networks
$endgroup$
$begingroup$
You can do these kind of things, but I don't know how efficient it would be..
$endgroup$
– mathreadler
Dec 14 '18 at 18:50
$begingroup$
Any idea to start with or any web ressource ?
$endgroup$
– Clifford
Dec 15 '18 at 18:00
add a comment |
$begingroup$
I'm trying to build a neural network to solve this optimization problem
minimize $f(x)$
s.t. $h(x)=0$
where $x =(x_1, x_2, dots, x_n)^T in R^n$, $f: R^n rightarrow R$ and $h: R^n rightarrow R^m$ are given functions and $m le n$. $f$ and $h$ are assumed to be twice continuous differentiable.
The idea is presented in this paper (IEEE abstract)
and consists in creating a neural network whose equilibrium point satisfies the necessary conditions of optimality.
Based on the Lagrange multiplier theory, the neural network will be governed by:
$frac{dx}{dt} = - nabla_x L(x, lambda) $
$frac{dlambda}{dt} = nabla_{lambda} L(x, lambda) $
where $L: R^{m+n} rightarrow R$ is the Lagrange function defined by: $L(x, lambda) = f(x) + lambda^T h(x)$, $lambda in R^m$
- I do not see how we could use the last equations to create a neural
network? - For a neural network, the output $(x^*, lambda^*)$ "must" be known,
also, how to implement forward propagation and backpropagation? - Could someone help me understand how to create such a network or, if
possible, provide a Matlab or Python code to implement the network?
Many thanks.
nonlinear-optimization neural-networks
$endgroup$
I'm trying to build a neural network to solve this optimization problem
minimize $f(x)$
s.t. $h(x)=0$
where $x =(x_1, x_2, dots, x_n)^T in R^n$, $f: R^n rightarrow R$ and $h: R^n rightarrow R^m$ are given functions and $m le n$. $f$ and $h$ are assumed to be twice continuous differentiable.
The idea is presented in this paper (IEEE abstract)
and consists in creating a neural network whose equilibrium point satisfies the necessary conditions of optimality.
Based on the Lagrange multiplier theory, the neural network will be governed by:
$frac{dx}{dt} = - nabla_x L(x, lambda) $
$frac{dlambda}{dt} = nabla_{lambda} L(x, lambda) $
where $L: R^{m+n} rightarrow R$ is the Lagrange function defined by: $L(x, lambda) = f(x) + lambda^T h(x)$, $lambda in R^m$
- I do not see how we could use the last equations to create a neural
network? - For a neural network, the output $(x^*, lambda^*)$ "must" be known,
also, how to implement forward propagation and backpropagation? - Could someone help me understand how to create such a network or, if
possible, provide a Matlab or Python code to implement the network?
Many thanks.
nonlinear-optimization neural-networks
nonlinear-optimization neural-networks
edited Dec 14 '18 at 18:35
Clifford
asked Dec 10 '18 at 19:35


CliffordClifford
162
162
$begingroup$
You can do these kind of things, but I don't know how efficient it would be..
$endgroup$
– mathreadler
Dec 14 '18 at 18:50
$begingroup$
Any idea to start with or any web ressource ?
$endgroup$
– Clifford
Dec 15 '18 at 18:00
add a comment |
$begingroup$
You can do these kind of things, but I don't know how efficient it would be..
$endgroup$
– mathreadler
Dec 14 '18 at 18:50
$begingroup$
Any idea to start with or any web ressource ?
$endgroup$
– Clifford
Dec 15 '18 at 18:00
$begingroup$
You can do these kind of things, but I don't know how efficient it would be..
$endgroup$
– mathreadler
Dec 14 '18 at 18:50
$begingroup$
You can do these kind of things, but I don't know how efficient it would be..
$endgroup$
– mathreadler
Dec 14 '18 at 18:50
$begingroup$
Any idea to start with or any web ressource ?
$endgroup$
– Clifford
Dec 15 '18 at 18:00
$begingroup$
Any idea to start with or any web ressource ?
$endgroup$
– Clifford
Dec 15 '18 at 18:00
add a comment |
0
active
oldest
votes
Your Answer
StackExchange.ifUsing("editor", function () {
return StackExchange.using("mathjaxEditing", function () {
StackExchange.MarkdownEditor.creationCallbacks.add(function (editor, postfix) {
StackExchange.mathjaxEditing.prepareWmdForMathJax(editor, postfix, [["$", "$"], ["\\(","\\)"]]);
});
});
}, "mathjax-editing");
StackExchange.ready(function() {
var channelOptions = {
tags: "".split(" "),
id: "69"
};
initTagRenderer("".split(" "), "".split(" "), channelOptions);
StackExchange.using("externalEditor", function() {
// Have to fire editor after snippets, if snippets enabled
if (StackExchange.settings.snippets.snippetsEnabled) {
StackExchange.using("snippets", function() {
createEditor();
});
}
else {
createEditor();
}
});
function createEditor() {
StackExchange.prepareEditor({
heartbeatType: 'answer',
autoActivateHeartbeat: false,
convertImagesToLinks: true,
noModals: true,
showLowRepImageUploadWarning: true,
reputationToPostImages: 10,
bindNavPrevention: true,
postfix: "",
imageUploader: {
brandingHtml: "Powered by u003ca class="icon-imgur-white" href="https://imgur.com/"u003eu003c/au003e",
contentPolicyHtml: "User contributions licensed under u003ca href="https://creativecommons.org/licenses/by-sa/3.0/"u003ecc by-sa 3.0 with attribution requiredu003c/au003e u003ca href="https://stackoverflow.com/legal/content-policy"u003e(content policy)u003c/au003e",
allowUrls: true
},
noCode: true, onDemand: true,
discardSelector: ".discard-answer"
,immediatelyShowMarkdownHelp:true
});
}
});
Sign up or log in
StackExchange.ready(function () {
StackExchange.helpers.onClickDraftSave('#login-link');
});
Sign up using Google
Sign up using Facebook
Sign up using Email and Password
Post as a guest
Required, but never shown
StackExchange.ready(
function () {
StackExchange.openid.initPostLogin('.new-post-login', 'https%3a%2f%2fmath.stackexchange.com%2fquestions%2f3034372%2fneural-network-to-solve-nonlinear-constrained-optimization-problem%23new-answer', 'question_page');
}
);
Post as a guest
Required, but never shown
0
active
oldest
votes
0
active
oldest
votes
active
oldest
votes
active
oldest
votes
Thanks for contributing an answer to Mathematics Stack Exchange!
- Please be sure to answer the question. Provide details and share your research!
But avoid …
- Asking for help, clarification, or responding to other answers.
- Making statements based on opinion; back them up with references or personal experience.
Use MathJax to format equations. MathJax reference.
To learn more, see our tips on writing great answers.
Sign up or log in
StackExchange.ready(function () {
StackExchange.helpers.onClickDraftSave('#login-link');
});
Sign up using Google
Sign up using Facebook
Sign up using Email and Password
Post as a guest
Required, but never shown
StackExchange.ready(
function () {
StackExchange.openid.initPostLogin('.new-post-login', 'https%3a%2f%2fmath.stackexchange.com%2fquestions%2f3034372%2fneural-network-to-solve-nonlinear-constrained-optimization-problem%23new-answer', 'question_page');
}
);
Post as a guest
Required, but never shown
Sign up or log in
StackExchange.ready(function () {
StackExchange.helpers.onClickDraftSave('#login-link');
});
Sign up using Google
Sign up using Facebook
Sign up using Email and Password
Post as a guest
Required, but never shown
Sign up or log in
StackExchange.ready(function () {
StackExchange.helpers.onClickDraftSave('#login-link');
});
Sign up using Google
Sign up using Facebook
Sign up using Email and Password
Post as a guest
Required, but never shown
Sign up or log in
StackExchange.ready(function () {
StackExchange.helpers.onClickDraftSave('#login-link');
});
Sign up using Google
Sign up using Facebook
Sign up using Email and Password
Sign up using Google
Sign up using Facebook
Sign up using Email and Password
Post as a guest
Required, but never shown
Required, but never shown
Required, but never shown
Required, but never shown
Required, but never shown
Required, but never shown
Required, but never shown
Required, but never shown
Required, but never shown
zgXDrIExw,jlaXkeGGgaKaqbF74,i,Y,SP9Q9EabRqmeZd5YiSrIZzPldX4H5sEOvmfnMREaj etl9QFP,YYkLDFJjYwebXGFl
$begingroup$
You can do these kind of things, but I don't know how efficient it would be..
$endgroup$
– mathreadler
Dec 14 '18 at 18:50
$begingroup$
Any idea to start with or any web ressource ?
$endgroup$
– Clifford
Dec 15 '18 at 18:00