Basic pattern recognition in binary (pixelated) image
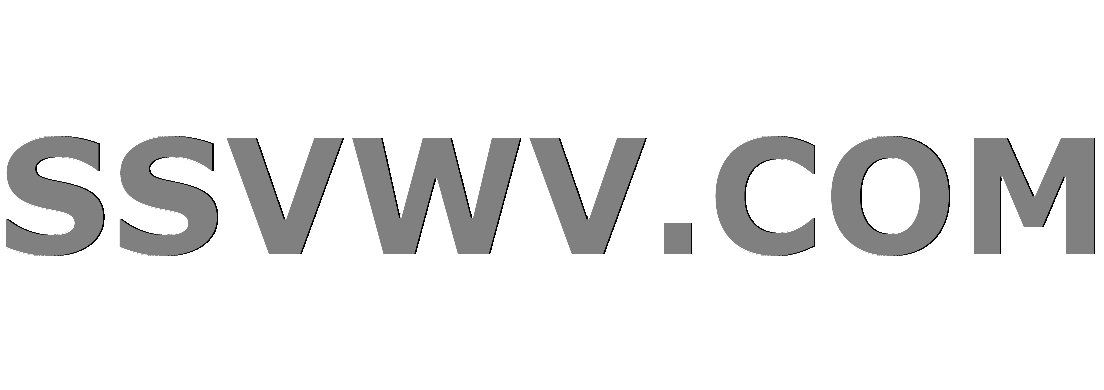
Multi tool use
.everyoneloves__top-leaderboard:empty,.everyoneloves__mid-leaderboard:empty,.everyoneloves__bot-mid-leaderboard:empty{ height:90px;width:728px;box-sizing:border-box;
}
Here is a cropped example (about 11x9
pixels) of the kind of images (which ultimately are actually all of size 28x28
, but stored in memory flattened as a 784
-components array) I will be trying to apply the algorithm on:
Basically, I want to be able to recognize when this shape appears (red lines are used to put emphasis on the separation of the pixels, while the surrounding black border is used to better outline the image against the white background of StackOverflow):
The orientation of it doesn't matter: it must be detected in any of its possible representations (rotations and symmetries) along the horizontal and vertical axis (so, for example, a 45° rotation shouldn't be considered, nor a diagonal symmetry: only consider 90°, 180°, and 270° rotations, for example).
There are two solutions to be found on that image that I first presented, though only one needs to be found (ignore the gray blurr surrounding the white region):
Take this other sample (which also demonstrates that the white figures inside the images aren't always fully surrounded by black pixels):
The function should return True because the shape is present:
Now, there is obviously a simple solution to this:
Use a variable such as pattern = [[1,0,0,0],[1,1,1,1]]
, produce its variations, and then slide all of the variations along the image until an exact match is found at which point the whole thing just stops and returns True
.
This would, however, in the worst case scenario, take up to 8*(28-2)*(28-4)*(2*4)
which is approximately 40000 operations for a single image, which seem a bit overkill (if I did my quick calculations right).
I'm guessing one way of making this naive approach better would be to first of all scan the image until I find the very first white pixel, and then start looking for the pattern 4 rows and 4 columns earlier than that point, but even that doesn't seem good enough.
Any ideas? Maybe this kind of function has already been implemented in some library? I'm looking for an implementation or an algorithm that beats my naive approach.
As a side note, while kind of a hack, I'm guessing this is the kind of problem that can be offloaded to the GPU but I do not have much experience with that. While it wouldn't be what I'm looking for primarily, if you provide an answer, feel free to add a GPU-related note.
python python-3.x image algorithm image-processing
add a comment |
Here is a cropped example (about 11x9
pixels) of the kind of images (which ultimately are actually all of size 28x28
, but stored in memory flattened as a 784
-components array) I will be trying to apply the algorithm on:
Basically, I want to be able to recognize when this shape appears (red lines are used to put emphasis on the separation of the pixels, while the surrounding black border is used to better outline the image against the white background of StackOverflow):
The orientation of it doesn't matter: it must be detected in any of its possible representations (rotations and symmetries) along the horizontal and vertical axis (so, for example, a 45° rotation shouldn't be considered, nor a diagonal symmetry: only consider 90°, 180°, and 270° rotations, for example).
There are two solutions to be found on that image that I first presented, though only one needs to be found (ignore the gray blurr surrounding the white region):
Take this other sample (which also demonstrates that the white figures inside the images aren't always fully surrounded by black pixels):
The function should return True because the shape is present:
Now, there is obviously a simple solution to this:
Use a variable such as pattern = [[1,0,0,0],[1,1,1,1]]
, produce its variations, and then slide all of the variations along the image until an exact match is found at which point the whole thing just stops and returns True
.
This would, however, in the worst case scenario, take up to 8*(28-2)*(28-4)*(2*4)
which is approximately 40000 operations for a single image, which seem a bit overkill (if I did my quick calculations right).
I'm guessing one way of making this naive approach better would be to first of all scan the image until I find the very first white pixel, and then start looking for the pattern 4 rows and 4 columns earlier than that point, but even that doesn't seem good enough.
Any ideas? Maybe this kind of function has already been implemented in some library? I'm looking for an implementation or an algorithm that beats my naive approach.
As a side note, while kind of a hack, I'm guessing this is the kind of problem that can be offloaded to the GPU but I do not have much experience with that. While it wouldn't be what I'm looking for primarily, if you provide an answer, feel free to add a GPU-related note.
python python-3.x image algorithm image-processing
When you manage to figure out a viable solution to your problem I'd really like to have a look at the source if you're willing to share it.
– Jake12342134
Nov 23 '18 at 12:00
I sure will. Would you mind sharing what kind of applications you have in mind for such a solution? Or do you want the code only because you are being curious? :)
– payne
Nov 23 '18 at 15:40
@Jake12342134 there you go: gist.github.com/payne911/…
– payne
Jan 8 at 1:07
Hello, thank you for the code! I'm excited to take a look. I asked solely out of curiosity!
– Jake12342134
Jan 9 at 14:57
@Jake12342134 no worries! I was using this code to detect whether or not a 28x28 picture represented a convex figure without any learning algorithm. I ended up with a >99% accuracy, which isn't bad.
– payne
Jan 9 at 15:16
add a comment |
Here is a cropped example (about 11x9
pixels) of the kind of images (which ultimately are actually all of size 28x28
, but stored in memory flattened as a 784
-components array) I will be trying to apply the algorithm on:
Basically, I want to be able to recognize when this shape appears (red lines are used to put emphasis on the separation of the pixels, while the surrounding black border is used to better outline the image against the white background of StackOverflow):
The orientation of it doesn't matter: it must be detected in any of its possible representations (rotations and symmetries) along the horizontal and vertical axis (so, for example, a 45° rotation shouldn't be considered, nor a diagonal symmetry: only consider 90°, 180°, and 270° rotations, for example).
There are two solutions to be found on that image that I first presented, though only one needs to be found (ignore the gray blurr surrounding the white region):
Take this other sample (which also demonstrates that the white figures inside the images aren't always fully surrounded by black pixels):
The function should return True because the shape is present:
Now, there is obviously a simple solution to this:
Use a variable such as pattern = [[1,0,0,0],[1,1,1,1]]
, produce its variations, and then slide all of the variations along the image until an exact match is found at which point the whole thing just stops and returns True
.
This would, however, in the worst case scenario, take up to 8*(28-2)*(28-4)*(2*4)
which is approximately 40000 operations for a single image, which seem a bit overkill (if I did my quick calculations right).
I'm guessing one way of making this naive approach better would be to first of all scan the image until I find the very first white pixel, and then start looking for the pattern 4 rows and 4 columns earlier than that point, but even that doesn't seem good enough.
Any ideas? Maybe this kind of function has already been implemented in some library? I'm looking for an implementation or an algorithm that beats my naive approach.
As a side note, while kind of a hack, I'm guessing this is the kind of problem that can be offloaded to the GPU but I do not have much experience with that. While it wouldn't be what I'm looking for primarily, if you provide an answer, feel free to add a GPU-related note.
python python-3.x image algorithm image-processing
Here is a cropped example (about 11x9
pixels) of the kind of images (which ultimately are actually all of size 28x28
, but stored in memory flattened as a 784
-components array) I will be trying to apply the algorithm on:
Basically, I want to be able to recognize when this shape appears (red lines are used to put emphasis on the separation of the pixels, while the surrounding black border is used to better outline the image against the white background of StackOverflow):
The orientation of it doesn't matter: it must be detected in any of its possible representations (rotations and symmetries) along the horizontal and vertical axis (so, for example, a 45° rotation shouldn't be considered, nor a diagonal symmetry: only consider 90°, 180°, and 270° rotations, for example).
There are two solutions to be found on that image that I first presented, though only one needs to be found (ignore the gray blurr surrounding the white region):
Take this other sample (which also demonstrates that the white figures inside the images aren't always fully surrounded by black pixels):
The function should return True because the shape is present:
Now, there is obviously a simple solution to this:
Use a variable such as pattern = [[1,0,0,0],[1,1,1,1]]
, produce its variations, and then slide all of the variations along the image until an exact match is found at which point the whole thing just stops and returns True
.
This would, however, in the worst case scenario, take up to 8*(28-2)*(28-4)*(2*4)
which is approximately 40000 operations for a single image, which seem a bit overkill (if I did my quick calculations right).
I'm guessing one way of making this naive approach better would be to first of all scan the image until I find the very first white pixel, and then start looking for the pattern 4 rows and 4 columns earlier than that point, but even that doesn't seem good enough.
Any ideas? Maybe this kind of function has already been implemented in some library? I'm looking for an implementation or an algorithm that beats my naive approach.
As a side note, while kind of a hack, I'm guessing this is the kind of problem that can be offloaded to the GPU but I do not have much experience with that. While it wouldn't be what I'm looking for primarily, if you provide an answer, feel free to add a GPU-related note.
python python-3.x image algorithm image-processing
python python-3.x image algorithm image-processing
edited Nov 23 '18 at 21:13
payne
asked Nov 23 '18 at 4:45


paynepayne
630322
630322
When you manage to figure out a viable solution to your problem I'd really like to have a look at the source if you're willing to share it.
– Jake12342134
Nov 23 '18 at 12:00
I sure will. Would you mind sharing what kind of applications you have in mind for such a solution? Or do you want the code only because you are being curious? :)
– payne
Nov 23 '18 at 15:40
@Jake12342134 there you go: gist.github.com/payne911/…
– payne
Jan 8 at 1:07
Hello, thank you for the code! I'm excited to take a look. I asked solely out of curiosity!
– Jake12342134
Jan 9 at 14:57
@Jake12342134 no worries! I was using this code to detect whether or not a 28x28 picture represented a convex figure without any learning algorithm. I ended up with a >99% accuracy, which isn't bad.
– payne
Jan 9 at 15:16
add a comment |
When you manage to figure out a viable solution to your problem I'd really like to have a look at the source if you're willing to share it.
– Jake12342134
Nov 23 '18 at 12:00
I sure will. Would you mind sharing what kind of applications you have in mind for such a solution? Or do you want the code only because you are being curious? :)
– payne
Nov 23 '18 at 15:40
@Jake12342134 there you go: gist.github.com/payne911/…
– payne
Jan 8 at 1:07
Hello, thank you for the code! I'm excited to take a look. I asked solely out of curiosity!
– Jake12342134
Jan 9 at 14:57
@Jake12342134 no worries! I was using this code to detect whether or not a 28x28 picture represented a convex figure without any learning algorithm. I ended up with a >99% accuracy, which isn't bad.
– payne
Jan 9 at 15:16
When you manage to figure out a viable solution to your problem I'd really like to have a look at the source if you're willing to share it.
– Jake12342134
Nov 23 '18 at 12:00
When you manage to figure out a viable solution to your problem I'd really like to have a look at the source if you're willing to share it.
– Jake12342134
Nov 23 '18 at 12:00
I sure will. Would you mind sharing what kind of applications you have in mind for such a solution? Or do you want the code only because you are being curious? :)
– payne
Nov 23 '18 at 15:40
I sure will. Would you mind sharing what kind of applications you have in mind for such a solution? Or do you want the code only because you are being curious? :)
– payne
Nov 23 '18 at 15:40
@Jake12342134 there you go: gist.github.com/payne911/…
– payne
Jan 8 at 1:07
@Jake12342134 there you go: gist.github.com/payne911/…
– payne
Jan 8 at 1:07
Hello, thank you for the code! I'm excited to take a look. I asked solely out of curiosity!
– Jake12342134
Jan 9 at 14:57
Hello, thank you for the code! I'm excited to take a look. I asked solely out of curiosity!
– Jake12342134
Jan 9 at 14:57
@Jake12342134 no worries! I was using this code to detect whether or not a 28x28 picture represented a convex figure without any learning algorithm. I ended up with a >99% accuracy, which isn't bad.
– payne
Jan 9 at 15:16
@Jake12342134 no worries! I was using this code to detect whether or not a 28x28 picture represented a convex figure without any learning algorithm. I ended up with a >99% accuracy, which isn't bad.
– payne
Jan 9 at 15:16
add a comment |
2 Answers
2
active
oldest
votes
If you have too many operations, think how to do less of them.
For this problem I'd use image integrals.
If you convolve a summing kernel over the image (this is a very fast operation in fft domain with just conv2
,imfilter
), you know that only locations where the integral is equal to 5 (in your case) are possible pattern matching places. Checking those (even for your 4 rotations) should be computationally very fast. There can not be more than 50 locations in your example image that fit this pattern.
My python is not too fluent, but this is the proof of concept for your first image in MATLAB, I am sure that translating this code should not be a problem.
% get the same image you have (imgur upscaled it and made it RGB)
I=rgb2gray(imread('https://i.stack.imgur.com/l3u4A.png'));
I=imresize(I,[9 11]);
I=double(I>50);
% Integral filter definition (with your desired size)
h=ones(3,4);
% horizontal and vertical filter (because your filter is not square)
Ifiltv=imfilter(I,h);
Ifilth=imfilter(I,h');
% find the locations where integral is exactly the value you want
[xh,yh]=find(Ifilth==5);
[xv,yv]=find(Ifiltv==5);
% this is just plotting, for completeness
figure()
imshow(I,);
hold on
plot(yh,xh,'r.');
plot(yv,xv,'r.');
This results in 14 locations to check. My standard computer takes 230ns on average on computing both image integrals, which I would call fast.
Also GPU computing is not a hack :D. Its the way to go with a big bunch of problems because of the enormous computing power they have. E.g. convolutions in GPUs are incredibly fast.
Would you mind explaining what you mean by "convolve a summing kernel over the image" ? My understanding is that you are basically sliding the pattern over the image as a mask and calculating the sum of the logical AND on each region, only keeping in memory the points where it was equal to 5 (since there are 5 white pixels in the pattern). I'm guessing you're doing so with every possible variation of the pattern so this operation would be repeated 8 times (because of symmetries/rotations). Note: I'm having trouble understanding how to use those red dots: none would allow the pattern to match
– payne
Nov 23 '18 at 15:49
@payne "sliding the pattern over the image as a mask" -> convolution. Then I selected the only locations (red dots) that sum to 5. Only those can match your pattern, no other location can. Im not saying they do, I am saying they are the only ones that have 5 pixels white. as your pattern. I have not given you the location of the pattern, I have given you a very reduced list of possible locations of the pattern. Then just check it using whatever you where doing before.
– Ander Biguri
Nov 23 '18 at 15:52
1
@payne no, summing all the values of an array isO(n)
on CPU andO(log(n))
in GPU. But you need to read a couple of books to understand this better, a comment here won't cut it. GPUs have simultaneous operations, so if you can do a thing at the same time as another one, the complexity is reduced, as its related to time.
– Ander Biguri
Nov 23 '18 at 15:55
1
@payne please take some time to read about some of the concepts I introduced, so you understand the answer better. Read about what "convolution" is in image processing and why it is fast. Also read about what an "image integral" is, as its the core of the answer. I am not using your pattern at no point, just doing a trick to reduce significantly the locations you need to check for the pattern.
– Ander Biguri
Nov 23 '18 at 15:58
1
I ended up implementing your solution: it worked perfectly. I really enjoyed reading on this technique: thank you for sharing! (I have shared a Gist containing my code as a reply to a commenter on the OP.)
– payne
Jan 8 at 1:55
|
show 4 more comments
The operation you are implementing is an operator in Mathematical Morphology called hit and miss.
It can be implemented very efficiently as a composition of two erosions. If the shape you’re detecting can be decomposed into a few simple geometrical shapes (especially rectangles are quick to compute) then the operator can be even more efficient.
You’ll find very efficient erosions in most image processing libraries, for example try OpenCV. OpenCV also has a hit and miss operator, here is a tutorial for how to use it.
As an example for what output to expect, I generated a simple test image (left), applied a hit and miss operator with a template that matches at exactly one place in the image (middle), and again with a template that does not match anywhere (right):
I did this in MATLAB, not Python, because I have it open and it's easiest for me to use. This is the code:
se = [1,1,1,1 % Defines the template
0,0,0,1];
img = [0,0,0,0,0,0 % Defines the test image
0,1,1,1,1,0
0,0,0,0,1,0
0,0,0,0,0,0
0,0,0,0,0,0
0,0,0,0,0,0];
img = dip_image(img,'bin');
res1 = hitmiss(img,se);
res2 = hitmiss(img,rot90(se,2));
% Quick-and-dirty display
h = dipshow([img,res1,res2]);
diptruesize(h,'tight',3000)
hold on
plot([5.5,5.5],[-0.5,5.5],'r-')
plot([11.5,11.5],[-0.5,5.5],'r-')
The code above uses the hit and miss operator as I implemented in DIPimage. This same implementation is available in Python in PyDIP as HitAndMiss
(there is not yet a binary release of PyDIP, you need to compile it yourself):
import PyDIP as dip
# ...
res = dip.HitAndMiss(img, se)
I knew there had to be something quite similar to my naive approach already implemented in OpenCV: thank you for the keyword and link! What is the algorithm's complexity, however ? And is it already implemented so as to offload to the GPU (I'm quite new to OpenCV in general) ?
– payne
Nov 23 '18 at 20:43
@payne: Complexity is O(n), but that is true for most image processing algorithms, you need to visit each pixel. Your example shape is separable into 3 lines (one black, the miss kernel, two white, together the hit kernel). An erosion with a line can be computed in time independent of its length, just 3 comparisons per image pixel. You’d do this 3 times, then compare the outputs, maybe 11 or 12 operations per pixel. — I’m not sure if OpenCV implements it this way. But OpenCV does have GPU implementations of a lot of its algorithms.
– Cris Luengo
Nov 23 '18 at 20:49
1
@Ander: Morpholog has a lot of very interesting operators, it’s still a very active, if small, community. Also most people think that morphology is only applicable to binary images...
– Cris Luengo
Nov 23 '18 at 20:53
1
@payne: Regarding the pattern: I did assume you had a 2x4 matrix there, but it doesn’t really matter, you can use any pattern in either Ander’s or my approach.
– Cris Luengo
Nov 23 '18 at 21:59
1
@payne: Ander’s method is quite efficient especially considering you need to do several orientations. The integral image you compute only once, and the final counts you compute twice (2x4 and 4x2). Then it depends on how many points match the count, how much work you end up doing. But it is a clever approach. I wanted to add this answer not because it is more efficient, but because this is a standard operator and there exist efficient implementations ready to use. It’s always best to do that over rolling your own, until you’ve demonstrated it’s a bottleneck in your processing.
– Cris Luengo
Nov 23 '18 at 22:00
|
show 7 more comments
Your Answer
StackExchange.ifUsing("editor", function () {
StackExchange.using("externalEditor", function () {
StackExchange.using("snippets", function () {
StackExchange.snippets.init();
});
});
}, "code-snippets");
StackExchange.ready(function() {
var channelOptions = {
tags: "".split(" "),
id: "1"
};
initTagRenderer("".split(" "), "".split(" "), channelOptions);
StackExchange.using("externalEditor", function() {
// Have to fire editor after snippets, if snippets enabled
if (StackExchange.settings.snippets.snippetsEnabled) {
StackExchange.using("snippets", function() {
createEditor();
});
}
else {
createEditor();
}
});
function createEditor() {
StackExchange.prepareEditor({
heartbeatType: 'answer',
autoActivateHeartbeat: false,
convertImagesToLinks: true,
noModals: true,
showLowRepImageUploadWarning: true,
reputationToPostImages: 10,
bindNavPrevention: true,
postfix: "",
imageUploader: {
brandingHtml: "Powered by u003ca class="icon-imgur-white" href="https://imgur.com/"u003eu003c/au003e",
contentPolicyHtml: "User contributions licensed under u003ca href="https://creativecommons.org/licenses/by-sa/3.0/"u003ecc by-sa 3.0 with attribution requiredu003c/au003e u003ca href="https://stackoverflow.com/legal/content-policy"u003e(content policy)u003c/au003e",
allowUrls: true
},
onDemand: true,
discardSelector: ".discard-answer"
,immediatelyShowMarkdownHelp:true
});
}
});
Sign up or log in
StackExchange.ready(function () {
StackExchange.helpers.onClickDraftSave('#login-link');
});
Sign up using Google
Sign up using Facebook
Sign up using Email and Password
Post as a guest
Required, but never shown
StackExchange.ready(
function () {
StackExchange.openid.initPostLogin('.new-post-login', 'https%3a%2f%2fstackoverflow.com%2fquestions%2f53440762%2fbasic-pattern-recognition-in-binary-pixelated-image%23new-answer', 'question_page');
}
);
Post as a guest
Required, but never shown
2 Answers
2
active
oldest
votes
2 Answers
2
active
oldest
votes
active
oldest
votes
active
oldest
votes
If you have too many operations, think how to do less of them.
For this problem I'd use image integrals.
If you convolve a summing kernel over the image (this is a very fast operation in fft domain with just conv2
,imfilter
), you know that only locations where the integral is equal to 5 (in your case) are possible pattern matching places. Checking those (even for your 4 rotations) should be computationally very fast. There can not be more than 50 locations in your example image that fit this pattern.
My python is not too fluent, but this is the proof of concept for your first image in MATLAB, I am sure that translating this code should not be a problem.
% get the same image you have (imgur upscaled it and made it RGB)
I=rgb2gray(imread('https://i.stack.imgur.com/l3u4A.png'));
I=imresize(I,[9 11]);
I=double(I>50);
% Integral filter definition (with your desired size)
h=ones(3,4);
% horizontal and vertical filter (because your filter is not square)
Ifiltv=imfilter(I,h);
Ifilth=imfilter(I,h');
% find the locations where integral is exactly the value you want
[xh,yh]=find(Ifilth==5);
[xv,yv]=find(Ifiltv==5);
% this is just plotting, for completeness
figure()
imshow(I,);
hold on
plot(yh,xh,'r.');
plot(yv,xv,'r.');
This results in 14 locations to check. My standard computer takes 230ns on average on computing both image integrals, which I would call fast.
Also GPU computing is not a hack :D. Its the way to go with a big bunch of problems because of the enormous computing power they have. E.g. convolutions in GPUs are incredibly fast.
Would you mind explaining what you mean by "convolve a summing kernel over the image" ? My understanding is that you are basically sliding the pattern over the image as a mask and calculating the sum of the logical AND on each region, only keeping in memory the points where it was equal to 5 (since there are 5 white pixels in the pattern). I'm guessing you're doing so with every possible variation of the pattern so this operation would be repeated 8 times (because of symmetries/rotations). Note: I'm having trouble understanding how to use those red dots: none would allow the pattern to match
– payne
Nov 23 '18 at 15:49
@payne "sliding the pattern over the image as a mask" -> convolution. Then I selected the only locations (red dots) that sum to 5. Only those can match your pattern, no other location can. Im not saying they do, I am saying they are the only ones that have 5 pixels white. as your pattern. I have not given you the location of the pattern, I have given you a very reduced list of possible locations of the pattern. Then just check it using whatever you where doing before.
– Ander Biguri
Nov 23 '18 at 15:52
1
@payne no, summing all the values of an array isO(n)
on CPU andO(log(n))
in GPU. But you need to read a couple of books to understand this better, a comment here won't cut it. GPUs have simultaneous operations, so if you can do a thing at the same time as another one, the complexity is reduced, as its related to time.
– Ander Biguri
Nov 23 '18 at 15:55
1
@payne please take some time to read about some of the concepts I introduced, so you understand the answer better. Read about what "convolution" is in image processing and why it is fast. Also read about what an "image integral" is, as its the core of the answer. I am not using your pattern at no point, just doing a trick to reduce significantly the locations you need to check for the pattern.
– Ander Biguri
Nov 23 '18 at 15:58
1
I ended up implementing your solution: it worked perfectly. I really enjoyed reading on this technique: thank you for sharing! (I have shared a Gist containing my code as a reply to a commenter on the OP.)
– payne
Jan 8 at 1:55
|
show 4 more comments
If you have too many operations, think how to do less of them.
For this problem I'd use image integrals.
If you convolve a summing kernel over the image (this is a very fast operation in fft domain with just conv2
,imfilter
), you know that only locations where the integral is equal to 5 (in your case) are possible pattern matching places. Checking those (even for your 4 rotations) should be computationally very fast. There can not be more than 50 locations in your example image that fit this pattern.
My python is not too fluent, but this is the proof of concept for your first image in MATLAB, I am sure that translating this code should not be a problem.
% get the same image you have (imgur upscaled it and made it RGB)
I=rgb2gray(imread('https://i.stack.imgur.com/l3u4A.png'));
I=imresize(I,[9 11]);
I=double(I>50);
% Integral filter definition (with your desired size)
h=ones(3,4);
% horizontal and vertical filter (because your filter is not square)
Ifiltv=imfilter(I,h);
Ifilth=imfilter(I,h');
% find the locations where integral is exactly the value you want
[xh,yh]=find(Ifilth==5);
[xv,yv]=find(Ifiltv==5);
% this is just plotting, for completeness
figure()
imshow(I,);
hold on
plot(yh,xh,'r.');
plot(yv,xv,'r.');
This results in 14 locations to check. My standard computer takes 230ns on average on computing both image integrals, which I would call fast.
Also GPU computing is not a hack :D. Its the way to go with a big bunch of problems because of the enormous computing power they have. E.g. convolutions in GPUs are incredibly fast.
Would you mind explaining what you mean by "convolve a summing kernel over the image" ? My understanding is that you are basically sliding the pattern over the image as a mask and calculating the sum of the logical AND on each region, only keeping in memory the points where it was equal to 5 (since there are 5 white pixels in the pattern). I'm guessing you're doing so with every possible variation of the pattern so this operation would be repeated 8 times (because of symmetries/rotations). Note: I'm having trouble understanding how to use those red dots: none would allow the pattern to match
– payne
Nov 23 '18 at 15:49
@payne "sliding the pattern over the image as a mask" -> convolution. Then I selected the only locations (red dots) that sum to 5. Only those can match your pattern, no other location can. Im not saying they do, I am saying they are the only ones that have 5 pixels white. as your pattern. I have not given you the location of the pattern, I have given you a very reduced list of possible locations of the pattern. Then just check it using whatever you where doing before.
– Ander Biguri
Nov 23 '18 at 15:52
1
@payne no, summing all the values of an array isO(n)
on CPU andO(log(n))
in GPU. But you need to read a couple of books to understand this better, a comment here won't cut it. GPUs have simultaneous operations, so if you can do a thing at the same time as another one, the complexity is reduced, as its related to time.
– Ander Biguri
Nov 23 '18 at 15:55
1
@payne please take some time to read about some of the concepts I introduced, so you understand the answer better. Read about what "convolution" is in image processing and why it is fast. Also read about what an "image integral" is, as its the core of the answer. I am not using your pattern at no point, just doing a trick to reduce significantly the locations you need to check for the pattern.
– Ander Biguri
Nov 23 '18 at 15:58
1
I ended up implementing your solution: it worked perfectly. I really enjoyed reading on this technique: thank you for sharing! (I have shared a Gist containing my code as a reply to a commenter on the OP.)
– payne
Jan 8 at 1:55
|
show 4 more comments
If you have too many operations, think how to do less of them.
For this problem I'd use image integrals.
If you convolve a summing kernel over the image (this is a very fast operation in fft domain with just conv2
,imfilter
), you know that only locations where the integral is equal to 5 (in your case) are possible pattern matching places. Checking those (even for your 4 rotations) should be computationally very fast. There can not be more than 50 locations in your example image that fit this pattern.
My python is not too fluent, but this is the proof of concept for your first image in MATLAB, I am sure that translating this code should not be a problem.
% get the same image you have (imgur upscaled it and made it RGB)
I=rgb2gray(imread('https://i.stack.imgur.com/l3u4A.png'));
I=imresize(I,[9 11]);
I=double(I>50);
% Integral filter definition (with your desired size)
h=ones(3,4);
% horizontal and vertical filter (because your filter is not square)
Ifiltv=imfilter(I,h);
Ifilth=imfilter(I,h');
% find the locations where integral is exactly the value you want
[xh,yh]=find(Ifilth==5);
[xv,yv]=find(Ifiltv==5);
% this is just plotting, for completeness
figure()
imshow(I,);
hold on
plot(yh,xh,'r.');
plot(yv,xv,'r.');
This results in 14 locations to check. My standard computer takes 230ns on average on computing both image integrals, which I would call fast.
Also GPU computing is not a hack :D. Its the way to go with a big bunch of problems because of the enormous computing power they have. E.g. convolutions in GPUs are incredibly fast.
If you have too many operations, think how to do less of them.
For this problem I'd use image integrals.
If you convolve a summing kernel over the image (this is a very fast operation in fft domain with just conv2
,imfilter
), you know that only locations where the integral is equal to 5 (in your case) are possible pattern matching places. Checking those (even for your 4 rotations) should be computationally very fast. There can not be more than 50 locations in your example image that fit this pattern.
My python is not too fluent, but this is the proof of concept for your first image in MATLAB, I am sure that translating this code should not be a problem.
% get the same image you have (imgur upscaled it and made it RGB)
I=rgb2gray(imread('https://i.stack.imgur.com/l3u4A.png'));
I=imresize(I,[9 11]);
I=double(I>50);
% Integral filter definition (with your desired size)
h=ones(3,4);
% horizontal and vertical filter (because your filter is not square)
Ifiltv=imfilter(I,h);
Ifilth=imfilter(I,h');
% find the locations where integral is exactly the value you want
[xh,yh]=find(Ifilth==5);
[xv,yv]=find(Ifiltv==5);
% this is just plotting, for completeness
figure()
imshow(I,);
hold on
plot(yh,xh,'r.');
plot(yv,xv,'r.');
This results in 14 locations to check. My standard computer takes 230ns on average on computing both image integrals, which I would call fast.
Also GPU computing is not a hack :D. Its the way to go with a big bunch of problems because of the enormous computing power they have. E.g. convolutions in GPUs are incredibly fast.
edited Nov 23 '18 at 20:23


Cris Luengo
23.3k52254
23.3k52254
answered Nov 23 '18 at 10:44


Ander BiguriAnder Biguri
26.8k105693
26.8k105693
Would you mind explaining what you mean by "convolve a summing kernel over the image" ? My understanding is that you are basically sliding the pattern over the image as a mask and calculating the sum of the logical AND on each region, only keeping in memory the points where it was equal to 5 (since there are 5 white pixels in the pattern). I'm guessing you're doing so with every possible variation of the pattern so this operation would be repeated 8 times (because of symmetries/rotations). Note: I'm having trouble understanding how to use those red dots: none would allow the pattern to match
– payne
Nov 23 '18 at 15:49
@payne "sliding the pattern over the image as a mask" -> convolution. Then I selected the only locations (red dots) that sum to 5. Only those can match your pattern, no other location can. Im not saying they do, I am saying they are the only ones that have 5 pixels white. as your pattern. I have not given you the location of the pattern, I have given you a very reduced list of possible locations of the pattern. Then just check it using whatever you where doing before.
– Ander Biguri
Nov 23 '18 at 15:52
1
@payne no, summing all the values of an array isO(n)
on CPU andO(log(n))
in GPU. But you need to read a couple of books to understand this better, a comment here won't cut it. GPUs have simultaneous operations, so if you can do a thing at the same time as another one, the complexity is reduced, as its related to time.
– Ander Biguri
Nov 23 '18 at 15:55
1
@payne please take some time to read about some of the concepts I introduced, so you understand the answer better. Read about what "convolution" is in image processing and why it is fast. Also read about what an "image integral" is, as its the core of the answer. I am not using your pattern at no point, just doing a trick to reduce significantly the locations you need to check for the pattern.
– Ander Biguri
Nov 23 '18 at 15:58
1
I ended up implementing your solution: it worked perfectly. I really enjoyed reading on this technique: thank you for sharing! (I have shared a Gist containing my code as a reply to a commenter on the OP.)
– payne
Jan 8 at 1:55
|
show 4 more comments
Would you mind explaining what you mean by "convolve a summing kernel over the image" ? My understanding is that you are basically sliding the pattern over the image as a mask and calculating the sum of the logical AND on each region, only keeping in memory the points where it was equal to 5 (since there are 5 white pixels in the pattern). I'm guessing you're doing so with every possible variation of the pattern so this operation would be repeated 8 times (because of symmetries/rotations). Note: I'm having trouble understanding how to use those red dots: none would allow the pattern to match
– payne
Nov 23 '18 at 15:49
@payne "sliding the pattern over the image as a mask" -> convolution. Then I selected the only locations (red dots) that sum to 5. Only those can match your pattern, no other location can. Im not saying they do, I am saying they are the only ones that have 5 pixels white. as your pattern. I have not given you the location of the pattern, I have given you a very reduced list of possible locations of the pattern. Then just check it using whatever you where doing before.
– Ander Biguri
Nov 23 '18 at 15:52
1
@payne no, summing all the values of an array isO(n)
on CPU andO(log(n))
in GPU. But you need to read a couple of books to understand this better, a comment here won't cut it. GPUs have simultaneous operations, so if you can do a thing at the same time as another one, the complexity is reduced, as its related to time.
– Ander Biguri
Nov 23 '18 at 15:55
1
@payne please take some time to read about some of the concepts I introduced, so you understand the answer better. Read about what "convolution" is in image processing and why it is fast. Also read about what an "image integral" is, as its the core of the answer. I am not using your pattern at no point, just doing a trick to reduce significantly the locations you need to check for the pattern.
– Ander Biguri
Nov 23 '18 at 15:58
1
I ended up implementing your solution: it worked perfectly. I really enjoyed reading on this technique: thank you for sharing! (I have shared a Gist containing my code as a reply to a commenter on the OP.)
– payne
Jan 8 at 1:55
Would you mind explaining what you mean by "convolve a summing kernel over the image" ? My understanding is that you are basically sliding the pattern over the image as a mask and calculating the sum of the logical AND on each region, only keeping in memory the points where it was equal to 5 (since there are 5 white pixels in the pattern). I'm guessing you're doing so with every possible variation of the pattern so this operation would be repeated 8 times (because of symmetries/rotations). Note: I'm having trouble understanding how to use those red dots: none would allow the pattern to match
– payne
Nov 23 '18 at 15:49
Would you mind explaining what you mean by "convolve a summing kernel over the image" ? My understanding is that you are basically sliding the pattern over the image as a mask and calculating the sum of the logical AND on each region, only keeping in memory the points where it was equal to 5 (since there are 5 white pixels in the pattern). I'm guessing you're doing so with every possible variation of the pattern so this operation would be repeated 8 times (because of symmetries/rotations). Note: I'm having trouble understanding how to use those red dots: none would allow the pattern to match
– payne
Nov 23 '18 at 15:49
@payne "sliding the pattern over the image as a mask" -> convolution. Then I selected the only locations (red dots) that sum to 5. Only those can match your pattern, no other location can. Im not saying they do, I am saying they are the only ones that have 5 pixels white. as your pattern. I have not given you the location of the pattern, I have given you a very reduced list of possible locations of the pattern. Then just check it using whatever you where doing before.
– Ander Biguri
Nov 23 '18 at 15:52
@payne "sliding the pattern over the image as a mask" -> convolution. Then I selected the only locations (red dots) that sum to 5. Only those can match your pattern, no other location can. Im not saying they do, I am saying they are the only ones that have 5 pixels white. as your pattern. I have not given you the location of the pattern, I have given you a very reduced list of possible locations of the pattern. Then just check it using whatever you where doing before.
– Ander Biguri
Nov 23 '18 at 15:52
1
1
@payne no, summing all the values of an array is
O(n)
on CPU and O(log(n))
in GPU. But you need to read a couple of books to understand this better, a comment here won't cut it. GPUs have simultaneous operations, so if you can do a thing at the same time as another one, the complexity is reduced, as its related to time.– Ander Biguri
Nov 23 '18 at 15:55
@payne no, summing all the values of an array is
O(n)
on CPU and O(log(n))
in GPU. But you need to read a couple of books to understand this better, a comment here won't cut it. GPUs have simultaneous operations, so if you can do a thing at the same time as another one, the complexity is reduced, as its related to time.– Ander Biguri
Nov 23 '18 at 15:55
1
1
@payne please take some time to read about some of the concepts I introduced, so you understand the answer better. Read about what "convolution" is in image processing and why it is fast. Also read about what an "image integral" is, as its the core of the answer. I am not using your pattern at no point, just doing a trick to reduce significantly the locations you need to check for the pattern.
– Ander Biguri
Nov 23 '18 at 15:58
@payne please take some time to read about some of the concepts I introduced, so you understand the answer better. Read about what "convolution" is in image processing and why it is fast. Also read about what an "image integral" is, as its the core of the answer. I am not using your pattern at no point, just doing a trick to reduce significantly the locations you need to check for the pattern.
– Ander Biguri
Nov 23 '18 at 15:58
1
1
I ended up implementing your solution: it worked perfectly. I really enjoyed reading on this technique: thank you for sharing! (I have shared a Gist containing my code as a reply to a commenter on the OP.)
– payne
Jan 8 at 1:55
I ended up implementing your solution: it worked perfectly. I really enjoyed reading on this technique: thank you for sharing! (I have shared a Gist containing my code as a reply to a commenter on the OP.)
– payne
Jan 8 at 1:55
|
show 4 more comments
The operation you are implementing is an operator in Mathematical Morphology called hit and miss.
It can be implemented very efficiently as a composition of two erosions. If the shape you’re detecting can be decomposed into a few simple geometrical shapes (especially rectangles are quick to compute) then the operator can be even more efficient.
You’ll find very efficient erosions in most image processing libraries, for example try OpenCV. OpenCV also has a hit and miss operator, here is a tutorial for how to use it.
As an example for what output to expect, I generated a simple test image (left), applied a hit and miss operator with a template that matches at exactly one place in the image (middle), and again with a template that does not match anywhere (right):
I did this in MATLAB, not Python, because I have it open and it's easiest for me to use. This is the code:
se = [1,1,1,1 % Defines the template
0,0,0,1];
img = [0,0,0,0,0,0 % Defines the test image
0,1,1,1,1,0
0,0,0,0,1,0
0,0,0,0,0,0
0,0,0,0,0,0
0,0,0,0,0,0];
img = dip_image(img,'bin');
res1 = hitmiss(img,se);
res2 = hitmiss(img,rot90(se,2));
% Quick-and-dirty display
h = dipshow([img,res1,res2]);
diptruesize(h,'tight',3000)
hold on
plot([5.5,5.5],[-0.5,5.5],'r-')
plot([11.5,11.5],[-0.5,5.5],'r-')
The code above uses the hit and miss operator as I implemented in DIPimage. This same implementation is available in Python in PyDIP as HitAndMiss
(there is not yet a binary release of PyDIP, you need to compile it yourself):
import PyDIP as dip
# ...
res = dip.HitAndMiss(img, se)
I knew there had to be something quite similar to my naive approach already implemented in OpenCV: thank you for the keyword and link! What is the algorithm's complexity, however ? And is it already implemented so as to offload to the GPU (I'm quite new to OpenCV in general) ?
– payne
Nov 23 '18 at 20:43
@payne: Complexity is O(n), but that is true for most image processing algorithms, you need to visit each pixel. Your example shape is separable into 3 lines (one black, the miss kernel, two white, together the hit kernel). An erosion with a line can be computed in time independent of its length, just 3 comparisons per image pixel. You’d do this 3 times, then compare the outputs, maybe 11 or 12 operations per pixel. — I’m not sure if OpenCV implements it this way. But OpenCV does have GPU implementations of a lot of its algorithms.
– Cris Luengo
Nov 23 '18 at 20:49
1
@Ander: Morpholog has a lot of very interesting operators, it’s still a very active, if small, community. Also most people think that morphology is only applicable to binary images...
– Cris Luengo
Nov 23 '18 at 20:53
1
@payne: Regarding the pattern: I did assume you had a 2x4 matrix there, but it doesn’t really matter, you can use any pattern in either Ander’s or my approach.
– Cris Luengo
Nov 23 '18 at 21:59
1
@payne: Ander’s method is quite efficient especially considering you need to do several orientations. The integral image you compute only once, and the final counts you compute twice (2x4 and 4x2). Then it depends on how many points match the count, how much work you end up doing. But it is a clever approach. I wanted to add this answer not because it is more efficient, but because this is a standard operator and there exist efficient implementations ready to use. It’s always best to do that over rolling your own, until you’ve demonstrated it’s a bottleneck in your processing.
– Cris Luengo
Nov 23 '18 at 22:00
|
show 7 more comments
The operation you are implementing is an operator in Mathematical Morphology called hit and miss.
It can be implemented very efficiently as a composition of two erosions. If the shape you’re detecting can be decomposed into a few simple geometrical shapes (especially rectangles are quick to compute) then the operator can be even more efficient.
You’ll find very efficient erosions in most image processing libraries, for example try OpenCV. OpenCV also has a hit and miss operator, here is a tutorial for how to use it.
As an example for what output to expect, I generated a simple test image (left), applied a hit and miss operator with a template that matches at exactly one place in the image (middle), and again with a template that does not match anywhere (right):
I did this in MATLAB, not Python, because I have it open and it's easiest for me to use. This is the code:
se = [1,1,1,1 % Defines the template
0,0,0,1];
img = [0,0,0,0,0,0 % Defines the test image
0,1,1,1,1,0
0,0,0,0,1,0
0,0,0,0,0,0
0,0,0,0,0,0
0,0,0,0,0,0];
img = dip_image(img,'bin');
res1 = hitmiss(img,se);
res2 = hitmiss(img,rot90(se,2));
% Quick-and-dirty display
h = dipshow([img,res1,res2]);
diptruesize(h,'tight',3000)
hold on
plot([5.5,5.5],[-0.5,5.5],'r-')
plot([11.5,11.5],[-0.5,5.5],'r-')
The code above uses the hit and miss operator as I implemented in DIPimage. This same implementation is available in Python in PyDIP as HitAndMiss
(there is not yet a binary release of PyDIP, you need to compile it yourself):
import PyDIP as dip
# ...
res = dip.HitAndMiss(img, se)
I knew there had to be something quite similar to my naive approach already implemented in OpenCV: thank you for the keyword and link! What is the algorithm's complexity, however ? And is it already implemented so as to offload to the GPU (I'm quite new to OpenCV in general) ?
– payne
Nov 23 '18 at 20:43
@payne: Complexity is O(n), but that is true for most image processing algorithms, you need to visit each pixel. Your example shape is separable into 3 lines (one black, the miss kernel, two white, together the hit kernel). An erosion with a line can be computed in time independent of its length, just 3 comparisons per image pixel. You’d do this 3 times, then compare the outputs, maybe 11 or 12 operations per pixel. — I’m not sure if OpenCV implements it this way. But OpenCV does have GPU implementations of a lot of its algorithms.
– Cris Luengo
Nov 23 '18 at 20:49
1
@Ander: Morpholog has a lot of very interesting operators, it’s still a very active, if small, community. Also most people think that morphology is only applicable to binary images...
– Cris Luengo
Nov 23 '18 at 20:53
1
@payne: Regarding the pattern: I did assume you had a 2x4 matrix there, but it doesn’t really matter, you can use any pattern in either Ander’s or my approach.
– Cris Luengo
Nov 23 '18 at 21:59
1
@payne: Ander’s method is quite efficient especially considering you need to do several orientations. The integral image you compute only once, and the final counts you compute twice (2x4 and 4x2). Then it depends on how many points match the count, how much work you end up doing. But it is a clever approach. I wanted to add this answer not because it is more efficient, but because this is a standard operator and there exist efficient implementations ready to use. It’s always best to do that over rolling your own, until you’ve demonstrated it’s a bottleneck in your processing.
– Cris Luengo
Nov 23 '18 at 22:00
|
show 7 more comments
The operation you are implementing is an operator in Mathematical Morphology called hit and miss.
It can be implemented very efficiently as a composition of two erosions. If the shape you’re detecting can be decomposed into a few simple geometrical shapes (especially rectangles are quick to compute) then the operator can be even more efficient.
You’ll find very efficient erosions in most image processing libraries, for example try OpenCV. OpenCV also has a hit and miss operator, here is a tutorial for how to use it.
As an example for what output to expect, I generated a simple test image (left), applied a hit and miss operator with a template that matches at exactly one place in the image (middle), and again with a template that does not match anywhere (right):
I did this in MATLAB, not Python, because I have it open and it's easiest for me to use. This is the code:
se = [1,1,1,1 % Defines the template
0,0,0,1];
img = [0,0,0,0,0,0 % Defines the test image
0,1,1,1,1,0
0,0,0,0,1,0
0,0,0,0,0,0
0,0,0,0,0,0
0,0,0,0,0,0];
img = dip_image(img,'bin');
res1 = hitmiss(img,se);
res2 = hitmiss(img,rot90(se,2));
% Quick-and-dirty display
h = dipshow([img,res1,res2]);
diptruesize(h,'tight',3000)
hold on
plot([5.5,5.5],[-0.5,5.5],'r-')
plot([11.5,11.5],[-0.5,5.5],'r-')
The code above uses the hit and miss operator as I implemented in DIPimage. This same implementation is available in Python in PyDIP as HitAndMiss
(there is not yet a binary release of PyDIP, you need to compile it yourself):
import PyDIP as dip
# ...
res = dip.HitAndMiss(img, se)
The operation you are implementing is an operator in Mathematical Morphology called hit and miss.
It can be implemented very efficiently as a composition of two erosions. If the shape you’re detecting can be decomposed into a few simple geometrical shapes (especially rectangles are quick to compute) then the operator can be even more efficient.
You’ll find very efficient erosions in most image processing libraries, for example try OpenCV. OpenCV also has a hit and miss operator, here is a tutorial for how to use it.
As an example for what output to expect, I generated a simple test image (left), applied a hit and miss operator with a template that matches at exactly one place in the image (middle), and again with a template that does not match anywhere (right):
I did this in MATLAB, not Python, because I have it open and it's easiest for me to use. This is the code:
se = [1,1,1,1 % Defines the template
0,0,0,1];
img = [0,0,0,0,0,0 % Defines the test image
0,1,1,1,1,0
0,0,0,0,1,0
0,0,0,0,0,0
0,0,0,0,0,0
0,0,0,0,0,0];
img = dip_image(img,'bin');
res1 = hitmiss(img,se);
res2 = hitmiss(img,rot90(se,2));
% Quick-and-dirty display
h = dipshow([img,res1,res2]);
diptruesize(h,'tight',3000)
hold on
plot([5.5,5.5],[-0.5,5.5],'r-')
plot([11.5,11.5],[-0.5,5.5],'r-')
The code above uses the hit and miss operator as I implemented in DIPimage. This same implementation is available in Python in PyDIP as HitAndMiss
(there is not yet a binary release of PyDIP, you need to compile it yourself):
import PyDIP as dip
# ...
res = dip.HitAndMiss(img, se)
edited Nov 25 '18 at 5:33
answered Nov 23 '18 at 20:21


Cris LuengoCris Luengo
23.3k52254
23.3k52254
I knew there had to be something quite similar to my naive approach already implemented in OpenCV: thank you for the keyword and link! What is the algorithm's complexity, however ? And is it already implemented so as to offload to the GPU (I'm quite new to OpenCV in general) ?
– payne
Nov 23 '18 at 20:43
@payne: Complexity is O(n), but that is true for most image processing algorithms, you need to visit each pixel. Your example shape is separable into 3 lines (one black, the miss kernel, two white, together the hit kernel). An erosion with a line can be computed in time independent of its length, just 3 comparisons per image pixel. You’d do this 3 times, then compare the outputs, maybe 11 or 12 operations per pixel. — I’m not sure if OpenCV implements it this way. But OpenCV does have GPU implementations of a lot of its algorithms.
– Cris Luengo
Nov 23 '18 at 20:49
1
@Ander: Morpholog has a lot of very interesting operators, it’s still a very active, if small, community. Also most people think that morphology is only applicable to binary images...
– Cris Luengo
Nov 23 '18 at 20:53
1
@payne: Regarding the pattern: I did assume you had a 2x4 matrix there, but it doesn’t really matter, you can use any pattern in either Ander’s or my approach.
– Cris Luengo
Nov 23 '18 at 21:59
1
@payne: Ander’s method is quite efficient especially considering you need to do several orientations. The integral image you compute only once, and the final counts you compute twice (2x4 and 4x2). Then it depends on how many points match the count, how much work you end up doing. But it is a clever approach. I wanted to add this answer not because it is more efficient, but because this is a standard operator and there exist efficient implementations ready to use. It’s always best to do that over rolling your own, until you’ve demonstrated it’s a bottleneck in your processing.
– Cris Luengo
Nov 23 '18 at 22:00
|
show 7 more comments
I knew there had to be something quite similar to my naive approach already implemented in OpenCV: thank you for the keyword and link! What is the algorithm's complexity, however ? And is it already implemented so as to offload to the GPU (I'm quite new to OpenCV in general) ?
– payne
Nov 23 '18 at 20:43
@payne: Complexity is O(n), but that is true for most image processing algorithms, you need to visit each pixel. Your example shape is separable into 3 lines (one black, the miss kernel, two white, together the hit kernel). An erosion with a line can be computed in time independent of its length, just 3 comparisons per image pixel. You’d do this 3 times, then compare the outputs, maybe 11 or 12 operations per pixel. — I’m not sure if OpenCV implements it this way. But OpenCV does have GPU implementations of a lot of its algorithms.
– Cris Luengo
Nov 23 '18 at 20:49
1
@Ander: Morpholog has a lot of very interesting operators, it’s still a very active, if small, community. Also most people think that morphology is only applicable to binary images...
– Cris Luengo
Nov 23 '18 at 20:53
1
@payne: Regarding the pattern: I did assume you had a 2x4 matrix there, but it doesn’t really matter, you can use any pattern in either Ander’s or my approach.
– Cris Luengo
Nov 23 '18 at 21:59
1
@payne: Ander’s method is quite efficient especially considering you need to do several orientations. The integral image you compute only once, and the final counts you compute twice (2x4 and 4x2). Then it depends on how many points match the count, how much work you end up doing. But it is a clever approach. I wanted to add this answer not because it is more efficient, but because this is a standard operator and there exist efficient implementations ready to use. It’s always best to do that over rolling your own, until you’ve demonstrated it’s a bottleneck in your processing.
– Cris Luengo
Nov 23 '18 at 22:00
I knew there had to be something quite similar to my naive approach already implemented in OpenCV: thank you for the keyword and link! What is the algorithm's complexity, however ? And is it already implemented so as to offload to the GPU (I'm quite new to OpenCV in general) ?
– payne
Nov 23 '18 at 20:43
I knew there had to be something quite similar to my naive approach already implemented in OpenCV: thank you for the keyword and link! What is the algorithm's complexity, however ? And is it already implemented so as to offload to the GPU (I'm quite new to OpenCV in general) ?
– payne
Nov 23 '18 at 20:43
@payne: Complexity is O(n), but that is true for most image processing algorithms, you need to visit each pixel. Your example shape is separable into 3 lines (one black, the miss kernel, two white, together the hit kernel). An erosion with a line can be computed in time independent of its length, just 3 comparisons per image pixel. You’d do this 3 times, then compare the outputs, maybe 11 or 12 operations per pixel. — I’m not sure if OpenCV implements it this way. But OpenCV does have GPU implementations of a lot of its algorithms.
– Cris Luengo
Nov 23 '18 at 20:49
@payne: Complexity is O(n), but that is true for most image processing algorithms, you need to visit each pixel. Your example shape is separable into 3 lines (one black, the miss kernel, two white, together the hit kernel). An erosion with a line can be computed in time independent of its length, just 3 comparisons per image pixel. You’d do this 3 times, then compare the outputs, maybe 11 or 12 operations per pixel. — I’m not sure if OpenCV implements it this way. But OpenCV does have GPU implementations of a lot of its algorithms.
– Cris Luengo
Nov 23 '18 at 20:49
1
1
@Ander: Morpholog has a lot of very interesting operators, it’s still a very active, if small, community. Also most people think that morphology is only applicable to binary images...
– Cris Luengo
Nov 23 '18 at 20:53
@Ander: Morpholog has a lot of very interesting operators, it’s still a very active, if small, community. Also most people think that morphology is only applicable to binary images...
– Cris Luengo
Nov 23 '18 at 20:53
1
1
@payne: Regarding the pattern: I did assume you had a 2x4 matrix there, but it doesn’t really matter, you can use any pattern in either Ander’s or my approach.
– Cris Luengo
Nov 23 '18 at 21:59
@payne: Regarding the pattern: I did assume you had a 2x4 matrix there, but it doesn’t really matter, you can use any pattern in either Ander’s or my approach.
– Cris Luengo
Nov 23 '18 at 21:59
1
1
@payne: Ander’s method is quite efficient especially considering you need to do several orientations. The integral image you compute only once, and the final counts you compute twice (2x4 and 4x2). Then it depends on how many points match the count, how much work you end up doing. But it is a clever approach. I wanted to add this answer not because it is more efficient, but because this is a standard operator and there exist efficient implementations ready to use. It’s always best to do that over rolling your own, until you’ve demonstrated it’s a bottleneck in your processing.
– Cris Luengo
Nov 23 '18 at 22:00
@payne: Ander’s method is quite efficient especially considering you need to do several orientations. The integral image you compute only once, and the final counts you compute twice (2x4 and 4x2). Then it depends on how many points match the count, how much work you end up doing. But it is a clever approach. I wanted to add this answer not because it is more efficient, but because this is a standard operator and there exist efficient implementations ready to use. It’s always best to do that over rolling your own, until you’ve demonstrated it’s a bottleneck in your processing.
– Cris Luengo
Nov 23 '18 at 22:00
|
show 7 more comments
Thanks for contributing an answer to Stack Overflow!
- Please be sure to answer the question. Provide details and share your research!
But avoid …
- Asking for help, clarification, or responding to other answers.
- Making statements based on opinion; back them up with references or personal experience.
To learn more, see our tips on writing great answers.
Sign up or log in
StackExchange.ready(function () {
StackExchange.helpers.onClickDraftSave('#login-link');
});
Sign up using Google
Sign up using Facebook
Sign up using Email and Password
Post as a guest
Required, but never shown
StackExchange.ready(
function () {
StackExchange.openid.initPostLogin('.new-post-login', 'https%3a%2f%2fstackoverflow.com%2fquestions%2f53440762%2fbasic-pattern-recognition-in-binary-pixelated-image%23new-answer', 'question_page');
}
);
Post as a guest
Required, but never shown
Sign up or log in
StackExchange.ready(function () {
StackExchange.helpers.onClickDraftSave('#login-link');
});
Sign up using Google
Sign up using Facebook
Sign up using Email and Password
Post as a guest
Required, but never shown
Sign up or log in
StackExchange.ready(function () {
StackExchange.helpers.onClickDraftSave('#login-link');
});
Sign up using Google
Sign up using Facebook
Sign up using Email and Password
Post as a guest
Required, but never shown
Sign up or log in
StackExchange.ready(function () {
StackExchange.helpers.onClickDraftSave('#login-link');
});
Sign up using Google
Sign up using Facebook
Sign up using Email and Password
Sign up using Google
Sign up using Facebook
Sign up using Email and Password
Post as a guest
Required, but never shown
Required, but never shown
Required, but never shown
Required, but never shown
Required, but never shown
Required, but never shown
Required, but never shown
Required, but never shown
Required, but never shown
KwVgLcWevOpk0IJ5W5Yb7L,Fe0,LSOZNZYOX,rp1Lc1lZ9
When you manage to figure out a viable solution to your problem I'd really like to have a look at the source if you're willing to share it.
– Jake12342134
Nov 23 '18 at 12:00
I sure will. Would you mind sharing what kind of applications you have in mind for such a solution? Or do you want the code only because you are being curious? :)
– payne
Nov 23 '18 at 15:40
@Jake12342134 there you go: gist.github.com/payne911/…
– payne
Jan 8 at 1:07
Hello, thank you for the code! I'm excited to take a look. I asked solely out of curiosity!
– Jake12342134
Jan 9 at 14:57
@Jake12342134 no worries! I was using this code to detect whether or not a 28x28 picture represented a convex figure without any learning algorithm. I ended up with a >99% accuracy, which isn't bad.
– payne
Jan 9 at 15:16