Working with SSIM loss function in tensorflow for RGB images
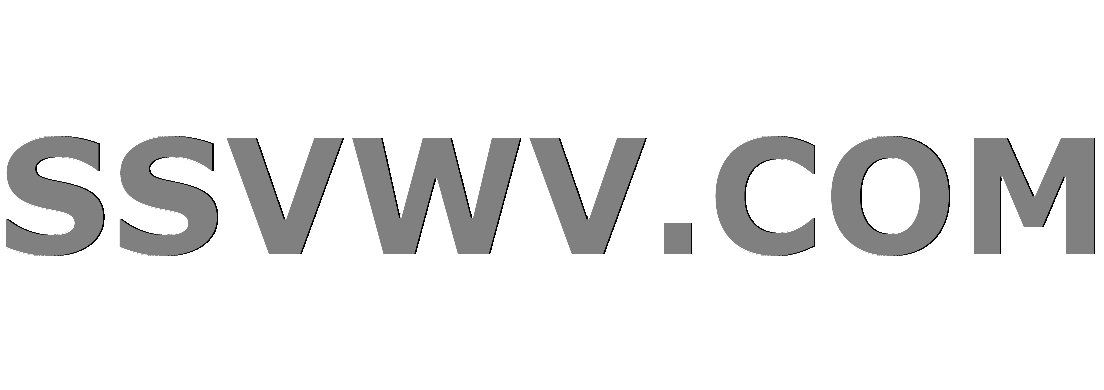
Multi tool use
I want to use SSIM metric as my loss function for the model I'm working on in tensorflow. SSIM should measure the similarity between my reconstructed output image of my denoising autoencoder and the input uncorrupted image (RGB).
As of what I understood, for using the SSIM metric in tensorflow, the images should be normalized to [0,1] or [0,255] and not [-1,1]. After converting my tensors to [0,1] and implementing SSIM as my loss function, the reconstructed image is black and white instead of a colorful RGB image.
tf.reduce_mean(tf.image.ssim(reconstructed, truth, 1.0))
My model is working fine with MSE (mean squared error), the reconstructed images are colorful (RGB).
using tf.losses.mean_squared_error(truth, reconstructed)
the reconstructed image would be RGB image, while using SSIM would give me a one dimensional image.
Why using SSIM as loss function gives me different result than MSE (in terms of reconstructed image channels) in tensorflow?
python image tensorflow mse ssim
add a comment |
I want to use SSIM metric as my loss function for the model I'm working on in tensorflow. SSIM should measure the similarity between my reconstructed output image of my denoising autoencoder and the input uncorrupted image (RGB).
As of what I understood, for using the SSIM metric in tensorflow, the images should be normalized to [0,1] or [0,255] and not [-1,1]. After converting my tensors to [0,1] and implementing SSIM as my loss function, the reconstructed image is black and white instead of a colorful RGB image.
tf.reduce_mean(tf.image.ssim(reconstructed, truth, 1.0))
My model is working fine with MSE (mean squared error), the reconstructed images are colorful (RGB).
using tf.losses.mean_squared_error(truth, reconstructed)
the reconstructed image would be RGB image, while using SSIM would give me a one dimensional image.
Why using SSIM as loss function gives me different result than MSE (in terms of reconstructed image channels) in tensorflow?
python image tensorflow mse ssim
add a comment |
I want to use SSIM metric as my loss function for the model I'm working on in tensorflow. SSIM should measure the similarity between my reconstructed output image of my denoising autoencoder and the input uncorrupted image (RGB).
As of what I understood, for using the SSIM metric in tensorflow, the images should be normalized to [0,1] or [0,255] and not [-1,1]. After converting my tensors to [0,1] and implementing SSIM as my loss function, the reconstructed image is black and white instead of a colorful RGB image.
tf.reduce_mean(tf.image.ssim(reconstructed, truth, 1.0))
My model is working fine with MSE (mean squared error), the reconstructed images are colorful (RGB).
using tf.losses.mean_squared_error(truth, reconstructed)
the reconstructed image would be RGB image, while using SSIM would give me a one dimensional image.
Why using SSIM as loss function gives me different result than MSE (in terms of reconstructed image channels) in tensorflow?
python image tensorflow mse ssim
I want to use SSIM metric as my loss function for the model I'm working on in tensorflow. SSIM should measure the similarity between my reconstructed output image of my denoising autoencoder and the input uncorrupted image (RGB).
As of what I understood, for using the SSIM metric in tensorflow, the images should be normalized to [0,1] or [0,255] and not [-1,1]. After converting my tensors to [0,1] and implementing SSIM as my loss function, the reconstructed image is black and white instead of a colorful RGB image.
tf.reduce_mean(tf.image.ssim(reconstructed, truth, 1.0))
My model is working fine with MSE (mean squared error), the reconstructed images are colorful (RGB).
using tf.losses.mean_squared_error(truth, reconstructed)
the reconstructed image would be RGB image, while using SSIM would give me a one dimensional image.
Why using SSIM as loss function gives me different result than MSE (in terms of reconstructed image channels) in tensorflow?
python image tensorflow mse ssim
python image tensorflow mse ssim
asked Oct 14 '18 at 0:40


Yousif HYousif H
434
434
add a comment |
add a comment |
2 Answers
2
active
oldest
votes
I was capable of solving the issue by changing the dynamic range of the images to 2.0, since I have images scaled between [-1, 1] by:
loss_rec = tf.reduce_mean(tf.image.ssim(truth, reconstructed, 2.0))
And since a better image quality is shown by a higher SSIM value, I had to minimize the negative of my loss function (SSIM) to optimize my model:
optimizer = tf.train.AdamOptimizer(learning_rate).minimize(-1 * loss_rec)
add a comment |
SSIM is designed to only measure the difference between two luminance signals. The RGB images are converted to greyscale before measuring similarity. If that was fed back into the loss function, it wouldn't know if the image was losing color saturation because it wouldn't show up in the error metric. That's just a theory.
add a comment |
Your Answer
StackExchange.ifUsing("editor", function () {
StackExchange.using("externalEditor", function () {
StackExchange.using("snippets", function () {
StackExchange.snippets.init();
});
});
}, "code-snippets");
StackExchange.ready(function() {
var channelOptions = {
tags: "".split(" "),
id: "1"
};
initTagRenderer("".split(" "), "".split(" "), channelOptions);
StackExchange.using("externalEditor", function() {
// Have to fire editor after snippets, if snippets enabled
if (StackExchange.settings.snippets.snippetsEnabled) {
StackExchange.using("snippets", function() {
createEditor();
});
}
else {
createEditor();
}
});
function createEditor() {
StackExchange.prepareEditor({
heartbeatType: 'answer',
autoActivateHeartbeat: false,
convertImagesToLinks: true,
noModals: true,
showLowRepImageUploadWarning: true,
reputationToPostImages: 10,
bindNavPrevention: true,
postfix: "",
imageUploader: {
brandingHtml: "Powered by u003ca class="icon-imgur-white" href="https://imgur.com/"u003eu003c/au003e",
contentPolicyHtml: "User contributions licensed under u003ca href="https://creativecommons.org/licenses/by-sa/3.0/"u003ecc by-sa 3.0 with attribution requiredu003c/au003e u003ca href="https://stackoverflow.com/legal/content-policy"u003e(content policy)u003c/au003e",
allowUrls: true
},
onDemand: true,
discardSelector: ".discard-answer"
,immediatelyShowMarkdownHelp:true
});
}
});
Sign up or log in
StackExchange.ready(function () {
StackExchange.helpers.onClickDraftSave('#login-link');
});
Sign up using Google
Sign up using Facebook
Sign up using Email and Password
Post as a guest
Required, but never shown
StackExchange.ready(
function () {
StackExchange.openid.initPostLogin('.new-post-login', 'https%3a%2f%2fstackoverflow.com%2fquestions%2f52798540%2fworking-with-ssim-loss-function-in-tensorflow-for-rgb-images%23new-answer', 'question_page');
}
);
Post as a guest
Required, but never shown
2 Answers
2
active
oldest
votes
2 Answers
2
active
oldest
votes
active
oldest
votes
active
oldest
votes
I was capable of solving the issue by changing the dynamic range of the images to 2.0, since I have images scaled between [-1, 1] by:
loss_rec = tf.reduce_mean(tf.image.ssim(truth, reconstructed, 2.0))
And since a better image quality is shown by a higher SSIM value, I had to minimize the negative of my loss function (SSIM) to optimize my model:
optimizer = tf.train.AdamOptimizer(learning_rate).minimize(-1 * loss_rec)
add a comment |
I was capable of solving the issue by changing the dynamic range of the images to 2.0, since I have images scaled between [-1, 1] by:
loss_rec = tf.reduce_mean(tf.image.ssim(truth, reconstructed, 2.0))
And since a better image quality is shown by a higher SSIM value, I had to minimize the negative of my loss function (SSIM) to optimize my model:
optimizer = tf.train.AdamOptimizer(learning_rate).minimize(-1 * loss_rec)
add a comment |
I was capable of solving the issue by changing the dynamic range of the images to 2.0, since I have images scaled between [-1, 1] by:
loss_rec = tf.reduce_mean(tf.image.ssim(truth, reconstructed, 2.0))
And since a better image quality is shown by a higher SSIM value, I had to minimize the negative of my loss function (SSIM) to optimize my model:
optimizer = tf.train.AdamOptimizer(learning_rate).minimize(-1 * loss_rec)
I was capable of solving the issue by changing the dynamic range of the images to 2.0, since I have images scaled between [-1, 1] by:
loss_rec = tf.reduce_mean(tf.image.ssim(truth, reconstructed, 2.0))
And since a better image quality is shown by a higher SSIM value, I had to minimize the negative of my loss function (SSIM) to optimize my model:
optimizer = tf.train.AdamOptimizer(learning_rate).minimize(-1 * loss_rec)
answered Nov 18 '18 at 14:17


Yousif HYousif H
434
434
add a comment |
add a comment |
SSIM is designed to only measure the difference between two luminance signals. The RGB images are converted to greyscale before measuring similarity. If that was fed back into the loss function, it wouldn't know if the image was losing color saturation because it wouldn't show up in the error metric. That's just a theory.
add a comment |
SSIM is designed to only measure the difference between two luminance signals. The RGB images are converted to greyscale before measuring similarity. If that was fed back into the loss function, it wouldn't know if the image was losing color saturation because it wouldn't show up in the error metric. That's just a theory.
add a comment |
SSIM is designed to only measure the difference between two luminance signals. The RGB images are converted to greyscale before measuring similarity. If that was fed back into the loss function, it wouldn't know if the image was losing color saturation because it wouldn't show up in the error metric. That's just a theory.
SSIM is designed to only measure the difference between two luminance signals. The RGB images are converted to greyscale before measuring similarity. If that was fed back into the loss function, it wouldn't know if the image was losing color saturation because it wouldn't show up in the error metric. That's just a theory.
answered Nov 16 '18 at 18:06
James StanardJames Stanard
1
1
add a comment |
add a comment |
Thanks for contributing an answer to Stack Overflow!
- Please be sure to answer the question. Provide details and share your research!
But avoid …
- Asking for help, clarification, or responding to other answers.
- Making statements based on opinion; back them up with references or personal experience.
To learn more, see our tips on writing great answers.
Some of your past answers have not been well-received, and you're in danger of being blocked from answering.
Please pay close attention to the following guidance:
- Please be sure to answer the question. Provide details and share your research!
But avoid …
- Asking for help, clarification, or responding to other answers.
- Making statements based on opinion; back them up with references or personal experience.
To learn more, see our tips on writing great answers.
Sign up or log in
StackExchange.ready(function () {
StackExchange.helpers.onClickDraftSave('#login-link');
});
Sign up using Google
Sign up using Facebook
Sign up using Email and Password
Post as a guest
Required, but never shown
StackExchange.ready(
function () {
StackExchange.openid.initPostLogin('.new-post-login', 'https%3a%2f%2fstackoverflow.com%2fquestions%2f52798540%2fworking-with-ssim-loss-function-in-tensorflow-for-rgb-images%23new-answer', 'question_page');
}
);
Post as a guest
Required, but never shown
Sign up or log in
StackExchange.ready(function () {
StackExchange.helpers.onClickDraftSave('#login-link');
});
Sign up using Google
Sign up using Facebook
Sign up using Email and Password
Post as a guest
Required, but never shown
Sign up or log in
StackExchange.ready(function () {
StackExchange.helpers.onClickDraftSave('#login-link');
});
Sign up using Google
Sign up using Facebook
Sign up using Email and Password
Post as a guest
Required, but never shown
Sign up or log in
StackExchange.ready(function () {
StackExchange.helpers.onClickDraftSave('#login-link');
});
Sign up using Google
Sign up using Facebook
Sign up using Email and Password
Sign up using Google
Sign up using Facebook
Sign up using Email and Password
Post as a guest
Required, but never shown
Required, but never shown
Required, but never shown
Required, but never shown
Required, but never shown
Required, but never shown
Required, but never shown
Required, but never shown
Required, but never shown
Rwmom4KS66,QF4,hu fhIUF