What is the definition of dataset (for Bonferroni purposes)?
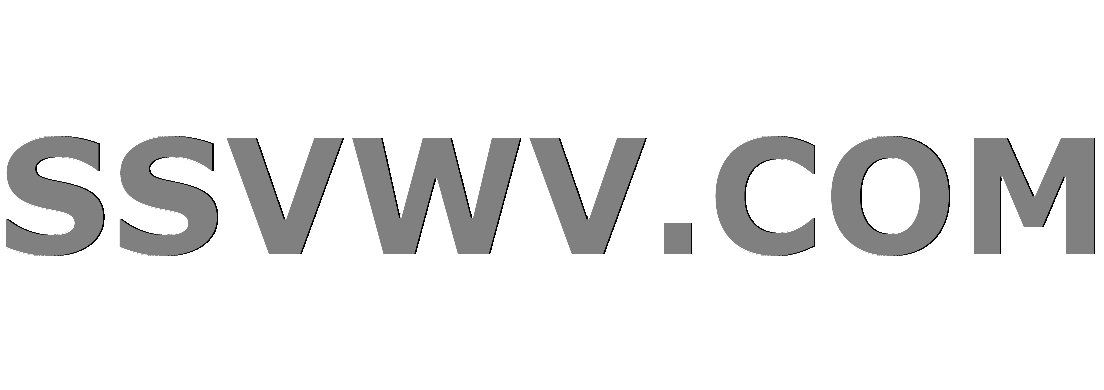
Multi tool use
I'm having difficulties finding a clear rule for when a series of test should be considered a multiple comparison and when we should apply p-value corrections (like Bonferroni).
I understand corrections must be applied every time multiple hypothesis are tested using the same dataset. A classical example is a Post-hoc Tukey test on data from an ANOVA.
However, what is the proper definition of a "dataset"? Whenever two tests share the a sample, are they the same dataset? Do they need to share all samples? The tests must share the same hypothesis?
I found many questions related to mine in this forum and online, but all of them seem to handle examples. If their particular case is or is not a multiple comparison and whether it needs correction, but none seem to come with a objective definition of "dataset".
multiple-comparisons
add a comment |
I'm having difficulties finding a clear rule for when a series of test should be considered a multiple comparison and when we should apply p-value corrections (like Bonferroni).
I understand corrections must be applied every time multiple hypothesis are tested using the same dataset. A classical example is a Post-hoc Tukey test on data from an ANOVA.
However, what is the proper definition of a "dataset"? Whenever two tests share the a sample, are they the same dataset? Do they need to share all samples? The tests must share the same hypothesis?
I found many questions related to mine in this forum and online, but all of them seem to handle examples. If their particular case is or is not a multiple comparison and whether it needs correction, but none seem to come with a objective definition of "dataset".
multiple-comparisons
For perspectives questioning the utility of such adjustments, see Rothman, K. J. (1990). No Adjustments Are Needed for Multiple Comparisons. Epidemiology, 1(1), 43–46. doi.org/10.1097/00001648-199001000-00010 and Saville, D. J. (1990). Multiple Comparison Procedures: The Practical Solution. The American Statistician, 44(2), 174–180. Retrieved from jstor.org/stable/2684163
– Heteroskedastic Jim
Dec 14 '18 at 17:47
add a comment |
I'm having difficulties finding a clear rule for when a series of test should be considered a multiple comparison and when we should apply p-value corrections (like Bonferroni).
I understand corrections must be applied every time multiple hypothesis are tested using the same dataset. A classical example is a Post-hoc Tukey test on data from an ANOVA.
However, what is the proper definition of a "dataset"? Whenever two tests share the a sample, are they the same dataset? Do they need to share all samples? The tests must share the same hypothesis?
I found many questions related to mine in this forum and online, but all of them seem to handle examples. If their particular case is or is not a multiple comparison and whether it needs correction, but none seem to come with a objective definition of "dataset".
multiple-comparisons
I'm having difficulties finding a clear rule for when a series of test should be considered a multiple comparison and when we should apply p-value corrections (like Bonferroni).
I understand corrections must be applied every time multiple hypothesis are tested using the same dataset. A classical example is a Post-hoc Tukey test on data from an ANOVA.
However, what is the proper definition of a "dataset"? Whenever two tests share the a sample, are they the same dataset? Do they need to share all samples? The tests must share the same hypothesis?
I found many questions related to mine in this forum and online, but all of them seem to handle examples. If their particular case is or is not a multiple comparison and whether it needs correction, but none seem to come with a objective definition of "dataset".
multiple-comparisons
multiple-comparisons
edited Dec 14 '18 at 16:10
asked Dec 14 '18 at 14:28
JMenezes
1747
1747
For perspectives questioning the utility of such adjustments, see Rothman, K. J. (1990). No Adjustments Are Needed for Multiple Comparisons. Epidemiology, 1(1), 43–46. doi.org/10.1097/00001648-199001000-00010 and Saville, D. J. (1990). Multiple Comparison Procedures: The Practical Solution. The American Statistician, 44(2), 174–180. Retrieved from jstor.org/stable/2684163
– Heteroskedastic Jim
Dec 14 '18 at 17:47
add a comment |
For perspectives questioning the utility of such adjustments, see Rothman, K. J. (1990). No Adjustments Are Needed for Multiple Comparisons. Epidemiology, 1(1), 43–46. doi.org/10.1097/00001648-199001000-00010 and Saville, D. J. (1990). Multiple Comparison Procedures: The Practical Solution. The American Statistician, 44(2), 174–180. Retrieved from jstor.org/stable/2684163
– Heteroskedastic Jim
Dec 14 '18 at 17:47
For perspectives questioning the utility of such adjustments, see Rothman, K. J. (1990). No Adjustments Are Needed for Multiple Comparisons. Epidemiology, 1(1), 43–46. doi.org/10.1097/00001648-199001000-00010 and Saville, D. J. (1990). Multiple Comparison Procedures: The Practical Solution. The American Statistician, 44(2), 174–180. Retrieved from jstor.org/stable/2684163
– Heteroskedastic Jim
Dec 14 '18 at 17:47
For perspectives questioning the utility of such adjustments, see Rothman, K. J. (1990). No Adjustments Are Needed for Multiple Comparisons. Epidemiology, 1(1), 43–46. doi.org/10.1097/00001648-199001000-00010 and Saville, D. J. (1990). Multiple Comparison Procedures: The Practical Solution. The American Statistician, 44(2), 174–180. Retrieved from jstor.org/stable/2684163
– Heteroskedastic Jim
Dec 14 '18 at 17:47
add a comment |
2 Answers
2
active
oldest
votes
The justification for control of multiple tests has to do with the family of tests. The family of tests can be mutually independent, which is often the case when they are drawn from different datasets; if so, Bonferroni is a good way to control for FWER. But in general, the concept of a dataset doesn't even enter the picture when discussing multiplicity.
It's assumed (incorrectly) that data in different datasets must, by design, be independent whereas two tests calculated with the same dataset must be dependent (also not necessarily correct). To justify and discuss the type of testing correction to use, one should consider the "family of tests". If the tests are dependent or correlated (that is to say that the $p$-value of one test actually depends on the $p$-value from another test), Bonferroni will be conservative. (NB: some rather dicey statistical practices can make Bonferroni anti-conservative, but that really boils down to non-transparency. For instance: test main hypothesis A. If main hypothesis non-significant, test hypotheses A and B and control with Bonferroni. here you allowed yourself to test B only because A was negative, this makes tests A and B negatively correlated even if the data contributing to these tests are independent.)
When the tests are independent, Bonferroni as you know is non-conservative in controlling the FWER. There is some grey area with respect to what constitutes a family of tests. This can be illustrated by considering subgroup analyses, here a global test may or may not have been significant, then the sample population is divvied up into K distinct groups. These groups are likely independent because they are arbitrary combinations of independent data from the parent dataset. You can view them as K distinct datasets, or 1 divided dataset, it doesn't matter. The point is that you conduct K tests. If you report the global hypothesis: at least one group showed heterogeneity of effect from the other groups, then you don't have to control for multiple comparisons. If, on the other hand, you report specific subgroup findings, you have to control for the K number of tests it took you to sniff that finding out. This is the XKCD Jelly Bean comic in a nutshell.
add a comment |
This is a much harder question than one would think and I doubt there are clear answers. The answer is relatively clear when we talk about clinical trials for regulatory purposes (whatever the regulatory authority says). I have the impression that this is an area of pragmatic traditions that have evolved in a kind of ad-hoc and not necessarily philosophically consistent manner within each field of science. There are simply some standard conventions that are typically (but not always) followed in certain fields. However, even with a field where type I error rate control per study has a lot of tradition such as medicine, there is still a debate on this topic.
add a comment |
Your Answer
StackExchange.ifUsing("editor", function () {
return StackExchange.using("mathjaxEditing", function () {
StackExchange.MarkdownEditor.creationCallbacks.add(function (editor, postfix) {
StackExchange.mathjaxEditing.prepareWmdForMathJax(editor, postfix, [["$", "$"], ["\\(","\\)"]]);
});
});
}, "mathjax-editing");
StackExchange.ready(function() {
var channelOptions = {
tags: "".split(" "),
id: "65"
};
initTagRenderer("".split(" "), "".split(" "), channelOptions);
StackExchange.using("externalEditor", function() {
// Have to fire editor after snippets, if snippets enabled
if (StackExchange.settings.snippets.snippetsEnabled) {
StackExchange.using("snippets", function() {
createEditor();
});
}
else {
createEditor();
}
});
function createEditor() {
StackExchange.prepareEditor({
heartbeatType: 'answer',
autoActivateHeartbeat: false,
convertImagesToLinks: false,
noModals: true,
showLowRepImageUploadWarning: true,
reputationToPostImages: null,
bindNavPrevention: true,
postfix: "",
imageUploader: {
brandingHtml: "Powered by u003ca class="icon-imgur-white" href="https://imgur.com/"u003eu003c/au003e",
contentPolicyHtml: "User contributions licensed under u003ca href="https://creativecommons.org/licenses/by-sa/3.0/"u003ecc by-sa 3.0 with attribution requiredu003c/au003e u003ca href="https://stackoverflow.com/legal/content-policy"u003e(content policy)u003c/au003e",
allowUrls: true
},
onDemand: true,
discardSelector: ".discard-answer"
,immediatelyShowMarkdownHelp:true
});
}
});
Sign up or log in
StackExchange.ready(function () {
StackExchange.helpers.onClickDraftSave('#login-link');
});
Sign up using Google
Sign up using Facebook
Sign up using Email and Password
Post as a guest
Required, but never shown
StackExchange.ready(
function () {
StackExchange.openid.initPostLogin('.new-post-login', 'https%3a%2f%2fstats.stackexchange.com%2fquestions%2f382014%2fwhat-is-the-definition-of-dataset-for-bonferroni-purposes%23new-answer', 'question_page');
}
);
Post as a guest
Required, but never shown
2 Answers
2
active
oldest
votes
2 Answers
2
active
oldest
votes
active
oldest
votes
active
oldest
votes
The justification for control of multiple tests has to do with the family of tests. The family of tests can be mutually independent, which is often the case when they are drawn from different datasets; if so, Bonferroni is a good way to control for FWER. But in general, the concept of a dataset doesn't even enter the picture when discussing multiplicity.
It's assumed (incorrectly) that data in different datasets must, by design, be independent whereas two tests calculated with the same dataset must be dependent (also not necessarily correct). To justify and discuss the type of testing correction to use, one should consider the "family of tests". If the tests are dependent or correlated (that is to say that the $p$-value of one test actually depends on the $p$-value from another test), Bonferroni will be conservative. (NB: some rather dicey statistical practices can make Bonferroni anti-conservative, but that really boils down to non-transparency. For instance: test main hypothesis A. If main hypothesis non-significant, test hypotheses A and B and control with Bonferroni. here you allowed yourself to test B only because A was negative, this makes tests A and B negatively correlated even if the data contributing to these tests are independent.)
When the tests are independent, Bonferroni as you know is non-conservative in controlling the FWER. There is some grey area with respect to what constitutes a family of tests. This can be illustrated by considering subgroup analyses, here a global test may or may not have been significant, then the sample population is divvied up into K distinct groups. These groups are likely independent because they are arbitrary combinations of independent data from the parent dataset. You can view them as K distinct datasets, or 1 divided dataset, it doesn't matter. The point is that you conduct K tests. If you report the global hypothesis: at least one group showed heterogeneity of effect from the other groups, then you don't have to control for multiple comparisons. If, on the other hand, you report specific subgroup findings, you have to control for the K number of tests it took you to sniff that finding out. This is the XKCD Jelly Bean comic in a nutshell.
add a comment |
The justification for control of multiple tests has to do with the family of tests. The family of tests can be mutually independent, which is often the case when they are drawn from different datasets; if so, Bonferroni is a good way to control for FWER. But in general, the concept of a dataset doesn't even enter the picture when discussing multiplicity.
It's assumed (incorrectly) that data in different datasets must, by design, be independent whereas two tests calculated with the same dataset must be dependent (also not necessarily correct). To justify and discuss the type of testing correction to use, one should consider the "family of tests". If the tests are dependent or correlated (that is to say that the $p$-value of one test actually depends on the $p$-value from another test), Bonferroni will be conservative. (NB: some rather dicey statistical practices can make Bonferroni anti-conservative, but that really boils down to non-transparency. For instance: test main hypothesis A. If main hypothesis non-significant, test hypotheses A and B and control with Bonferroni. here you allowed yourself to test B only because A was negative, this makes tests A and B negatively correlated even if the data contributing to these tests are independent.)
When the tests are independent, Bonferroni as you know is non-conservative in controlling the FWER. There is some grey area with respect to what constitutes a family of tests. This can be illustrated by considering subgroup analyses, here a global test may or may not have been significant, then the sample population is divvied up into K distinct groups. These groups are likely independent because they are arbitrary combinations of independent data from the parent dataset. You can view them as K distinct datasets, or 1 divided dataset, it doesn't matter. The point is that you conduct K tests. If you report the global hypothesis: at least one group showed heterogeneity of effect from the other groups, then you don't have to control for multiple comparisons. If, on the other hand, you report specific subgroup findings, you have to control for the K number of tests it took you to sniff that finding out. This is the XKCD Jelly Bean comic in a nutshell.
add a comment |
The justification for control of multiple tests has to do with the family of tests. The family of tests can be mutually independent, which is often the case when they are drawn from different datasets; if so, Bonferroni is a good way to control for FWER. But in general, the concept of a dataset doesn't even enter the picture when discussing multiplicity.
It's assumed (incorrectly) that data in different datasets must, by design, be independent whereas two tests calculated with the same dataset must be dependent (also not necessarily correct). To justify and discuss the type of testing correction to use, one should consider the "family of tests". If the tests are dependent or correlated (that is to say that the $p$-value of one test actually depends on the $p$-value from another test), Bonferroni will be conservative. (NB: some rather dicey statistical practices can make Bonferroni anti-conservative, but that really boils down to non-transparency. For instance: test main hypothesis A. If main hypothesis non-significant, test hypotheses A and B and control with Bonferroni. here you allowed yourself to test B only because A was negative, this makes tests A and B negatively correlated even if the data contributing to these tests are independent.)
When the tests are independent, Bonferroni as you know is non-conservative in controlling the FWER. There is some grey area with respect to what constitutes a family of tests. This can be illustrated by considering subgroup analyses, here a global test may or may not have been significant, then the sample population is divvied up into K distinct groups. These groups are likely independent because they are arbitrary combinations of independent data from the parent dataset. You can view them as K distinct datasets, or 1 divided dataset, it doesn't matter. The point is that you conduct K tests. If you report the global hypothesis: at least one group showed heterogeneity of effect from the other groups, then you don't have to control for multiple comparisons. If, on the other hand, you report specific subgroup findings, you have to control for the K number of tests it took you to sniff that finding out. This is the XKCD Jelly Bean comic in a nutshell.
The justification for control of multiple tests has to do with the family of tests. The family of tests can be mutually independent, which is often the case when they are drawn from different datasets; if so, Bonferroni is a good way to control for FWER. But in general, the concept of a dataset doesn't even enter the picture when discussing multiplicity.
It's assumed (incorrectly) that data in different datasets must, by design, be independent whereas two tests calculated with the same dataset must be dependent (also not necessarily correct). To justify and discuss the type of testing correction to use, one should consider the "family of tests". If the tests are dependent or correlated (that is to say that the $p$-value of one test actually depends on the $p$-value from another test), Bonferroni will be conservative. (NB: some rather dicey statistical practices can make Bonferroni anti-conservative, but that really boils down to non-transparency. For instance: test main hypothesis A. If main hypothesis non-significant, test hypotheses A and B and control with Bonferroni. here you allowed yourself to test B only because A was negative, this makes tests A and B negatively correlated even if the data contributing to these tests are independent.)
When the tests are independent, Bonferroni as you know is non-conservative in controlling the FWER. There is some grey area with respect to what constitutes a family of tests. This can be illustrated by considering subgroup analyses, here a global test may or may not have been significant, then the sample population is divvied up into K distinct groups. These groups are likely independent because they are arbitrary combinations of independent data from the parent dataset. You can view them as K distinct datasets, or 1 divided dataset, it doesn't matter. The point is that you conduct K tests. If you report the global hypothesis: at least one group showed heterogeneity of effect from the other groups, then you don't have to control for multiple comparisons. If, on the other hand, you report specific subgroup findings, you have to control for the K number of tests it took you to sniff that finding out. This is the XKCD Jelly Bean comic in a nutshell.
edited Dec 14 '18 at 17:15
answered Dec 14 '18 at 16:36
AdamO
32.8k261140
32.8k261140
add a comment |
add a comment |
This is a much harder question than one would think and I doubt there are clear answers. The answer is relatively clear when we talk about clinical trials for regulatory purposes (whatever the regulatory authority says). I have the impression that this is an area of pragmatic traditions that have evolved in a kind of ad-hoc and not necessarily philosophically consistent manner within each field of science. There are simply some standard conventions that are typically (but not always) followed in certain fields. However, even with a field where type I error rate control per study has a lot of tradition such as medicine, there is still a debate on this topic.
add a comment |
This is a much harder question than one would think and I doubt there are clear answers. The answer is relatively clear when we talk about clinical trials for regulatory purposes (whatever the regulatory authority says). I have the impression that this is an area of pragmatic traditions that have evolved in a kind of ad-hoc and not necessarily philosophically consistent manner within each field of science. There are simply some standard conventions that are typically (but not always) followed in certain fields. However, even with a field where type I error rate control per study has a lot of tradition such as medicine, there is still a debate on this topic.
add a comment |
This is a much harder question than one would think and I doubt there are clear answers. The answer is relatively clear when we talk about clinical trials for regulatory purposes (whatever the regulatory authority says). I have the impression that this is an area of pragmatic traditions that have evolved in a kind of ad-hoc and not necessarily philosophically consistent manner within each field of science. There are simply some standard conventions that are typically (but not always) followed in certain fields. However, even with a field where type I error rate control per study has a lot of tradition such as medicine, there is still a debate on this topic.
This is a much harder question than one would think and I doubt there are clear answers. The answer is relatively clear when we talk about clinical trials for regulatory purposes (whatever the regulatory authority says). I have the impression that this is an area of pragmatic traditions that have evolved in a kind of ad-hoc and not necessarily philosophically consistent manner within each field of science. There are simply some standard conventions that are typically (but not always) followed in certain fields. However, even with a field where type I error rate control per study has a lot of tradition such as medicine, there is still a debate on this topic.
answered Dec 14 '18 at 17:20


Björn
9,9051938
9,9051938
add a comment |
add a comment |
Thanks for contributing an answer to Cross Validated!
- Please be sure to answer the question. Provide details and share your research!
But avoid …
- Asking for help, clarification, or responding to other answers.
- Making statements based on opinion; back them up with references or personal experience.
Use MathJax to format equations. MathJax reference.
To learn more, see our tips on writing great answers.
Some of your past answers have not been well-received, and you're in danger of being blocked from answering.
Please pay close attention to the following guidance:
- Please be sure to answer the question. Provide details and share your research!
But avoid …
- Asking for help, clarification, or responding to other answers.
- Making statements based on opinion; back them up with references or personal experience.
To learn more, see our tips on writing great answers.
Sign up or log in
StackExchange.ready(function () {
StackExchange.helpers.onClickDraftSave('#login-link');
});
Sign up using Google
Sign up using Facebook
Sign up using Email and Password
Post as a guest
Required, but never shown
StackExchange.ready(
function () {
StackExchange.openid.initPostLogin('.new-post-login', 'https%3a%2f%2fstats.stackexchange.com%2fquestions%2f382014%2fwhat-is-the-definition-of-dataset-for-bonferroni-purposes%23new-answer', 'question_page');
}
);
Post as a guest
Required, but never shown
Sign up or log in
StackExchange.ready(function () {
StackExchange.helpers.onClickDraftSave('#login-link');
});
Sign up using Google
Sign up using Facebook
Sign up using Email and Password
Post as a guest
Required, but never shown
Sign up or log in
StackExchange.ready(function () {
StackExchange.helpers.onClickDraftSave('#login-link');
});
Sign up using Google
Sign up using Facebook
Sign up using Email and Password
Post as a guest
Required, but never shown
Sign up or log in
StackExchange.ready(function () {
StackExchange.helpers.onClickDraftSave('#login-link');
});
Sign up using Google
Sign up using Facebook
Sign up using Email and Password
Sign up using Google
Sign up using Facebook
Sign up using Email and Password
Post as a guest
Required, but never shown
Required, but never shown
Required, but never shown
Required, but never shown
Required, but never shown
Required, but never shown
Required, but never shown
Required, but never shown
Required, but never shown
nPX2,iRDUp2,Mz4szSeHVA6Je1RWia SyG5U WKKUWi
For perspectives questioning the utility of such adjustments, see Rothman, K. J. (1990). No Adjustments Are Needed for Multiple Comparisons. Epidemiology, 1(1), 43–46. doi.org/10.1097/00001648-199001000-00010 and Saville, D. J. (1990). Multiple Comparison Procedures: The Practical Solution. The American Statistician, 44(2), 174–180. Retrieved from jstor.org/stable/2684163
– Heteroskedastic Jim
Dec 14 '18 at 17:47