Numba : difference between first time execution and following executions
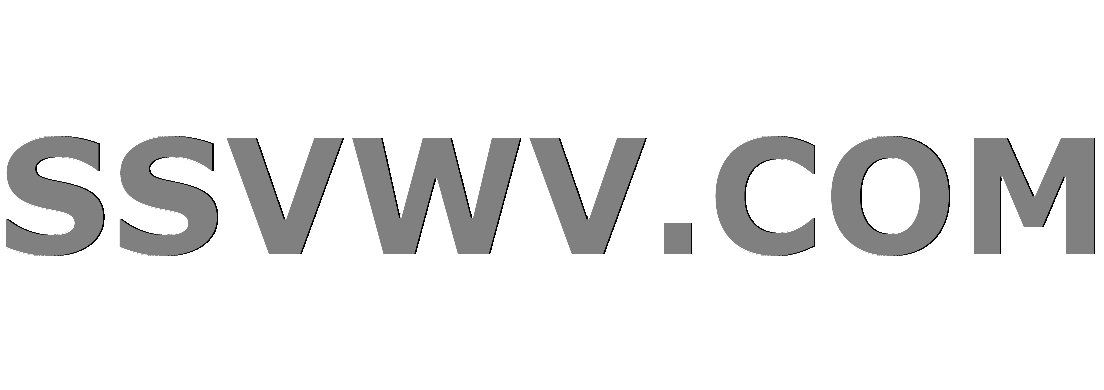
Multi tool use
I just started to use numba to improve performance of my programs. I have reduce the case that I will present
import numba as nb
import numpy as np
from time import time
def dt_max(U,f, eps=1e-5):
return np.min( np.abs( U ) / ( np.abs( f ) + eps ) )
@nb.njit(cache=True)
def fast_dt_max(U,f, eps=1e-5):
m=U[0]
if m<0 : m=-U[0]
for i in range(len(U)) :
v = abs(U[i]) / ( abs(f[i]) + eps )
if v < m : m = v
return m
N=100
Niter = int(1e5)
x=np.linspace(-50,50,N)
t0 = time()
for i in range(Niter):
dt_max(x,x)
print('numpy',time()-t0)
t0 = time()
for i in range(Niter):
fast_dt_max(x,x)
print('numba' ,time()-t0)
I execute this entire file in spyder under the following distribution :
Python 3.5.5 |Anaconda custom (64-bit)| (default, Apr 7 2018, 04:52:34) [MSC v.1900 64 bit (AMD64)]
Type "copyright", "credits" or "license" for more information.
The problem is the following. When I execute for the first time this program, it looks work good :
runfile('E:/02-Codes/TestCode/Python_numba/bug_second_execution.py', wdir='E:/02-Codes/TestCode/Python_numba')
numpy 0.45239996910095215
numba 0.2964000701904297
But when I repeat the execution of all file :
runfile('E:/02-Codes/TestCode/Python_numba/bug_second_execution.py', wdir='E:/02-Codes/TestCode/Python_numba')
numpy 0.45239996910095215
numba 3.5879998207092285
runfile('E:/02-Codes/TestCode/Python_numba/bug_second_execution.py', wdir='E:/02-Codes/TestCode/Python_numba')
numpy 0.4679999351501465
numba 3.5734000205993652
The numba's performance are not the same at all. If I restart Python kernel in my spyder environment, the problemn happens again : first execution is good, all the followings not.
So the first question is : why ? and the second is : how to avoid that ?
Thanks for your help
python numba execution-time
add a comment |
I just started to use numba to improve performance of my programs. I have reduce the case that I will present
import numba as nb
import numpy as np
from time import time
def dt_max(U,f, eps=1e-5):
return np.min( np.abs( U ) / ( np.abs( f ) + eps ) )
@nb.njit(cache=True)
def fast_dt_max(U,f, eps=1e-5):
m=U[0]
if m<0 : m=-U[0]
for i in range(len(U)) :
v = abs(U[i]) / ( abs(f[i]) + eps )
if v < m : m = v
return m
N=100
Niter = int(1e5)
x=np.linspace(-50,50,N)
t0 = time()
for i in range(Niter):
dt_max(x,x)
print('numpy',time()-t0)
t0 = time()
for i in range(Niter):
fast_dt_max(x,x)
print('numba' ,time()-t0)
I execute this entire file in spyder under the following distribution :
Python 3.5.5 |Anaconda custom (64-bit)| (default, Apr 7 2018, 04:52:34) [MSC v.1900 64 bit (AMD64)]
Type "copyright", "credits" or "license" for more information.
The problem is the following. When I execute for the first time this program, it looks work good :
runfile('E:/02-Codes/TestCode/Python_numba/bug_second_execution.py', wdir='E:/02-Codes/TestCode/Python_numba')
numpy 0.45239996910095215
numba 0.2964000701904297
But when I repeat the execution of all file :
runfile('E:/02-Codes/TestCode/Python_numba/bug_second_execution.py', wdir='E:/02-Codes/TestCode/Python_numba')
numpy 0.45239996910095215
numba 3.5879998207092285
runfile('E:/02-Codes/TestCode/Python_numba/bug_second_execution.py', wdir='E:/02-Codes/TestCode/Python_numba')
numpy 0.4679999351501465
numba 3.5734000205993652
The numba's performance are not the same at all. If I restart Python kernel in my spyder environment, the problemn happens again : first execution is good, all the followings not.
So the first question is : why ? and the second is : how to avoid that ?
Thanks for your help
python numba execution-time
1
The problem seems to come from setting a default variable for eps. This causes a recompilation after reopening the interpreter. You can check this behaviour with setting the environment variable NUMBA_DEBUG_CACHE to one. eg. (set NUMBA_DEBUG_CACHE=1) and setting no default value for eps. If there are many cached versions for one function in the cache directory, this can also explain the very slow timing for loading the precached version.
– max9111
Nov 20 '18 at 10:15
add a comment |
I just started to use numba to improve performance of my programs. I have reduce the case that I will present
import numba as nb
import numpy as np
from time import time
def dt_max(U,f, eps=1e-5):
return np.min( np.abs( U ) / ( np.abs( f ) + eps ) )
@nb.njit(cache=True)
def fast_dt_max(U,f, eps=1e-5):
m=U[0]
if m<0 : m=-U[0]
for i in range(len(U)) :
v = abs(U[i]) / ( abs(f[i]) + eps )
if v < m : m = v
return m
N=100
Niter = int(1e5)
x=np.linspace(-50,50,N)
t0 = time()
for i in range(Niter):
dt_max(x,x)
print('numpy',time()-t0)
t0 = time()
for i in range(Niter):
fast_dt_max(x,x)
print('numba' ,time()-t0)
I execute this entire file in spyder under the following distribution :
Python 3.5.5 |Anaconda custom (64-bit)| (default, Apr 7 2018, 04:52:34) [MSC v.1900 64 bit (AMD64)]
Type "copyright", "credits" or "license" for more information.
The problem is the following. When I execute for the first time this program, it looks work good :
runfile('E:/02-Codes/TestCode/Python_numba/bug_second_execution.py', wdir='E:/02-Codes/TestCode/Python_numba')
numpy 0.45239996910095215
numba 0.2964000701904297
But when I repeat the execution of all file :
runfile('E:/02-Codes/TestCode/Python_numba/bug_second_execution.py', wdir='E:/02-Codes/TestCode/Python_numba')
numpy 0.45239996910095215
numba 3.5879998207092285
runfile('E:/02-Codes/TestCode/Python_numba/bug_second_execution.py', wdir='E:/02-Codes/TestCode/Python_numba')
numpy 0.4679999351501465
numba 3.5734000205993652
The numba's performance are not the same at all. If I restart Python kernel in my spyder environment, the problemn happens again : first execution is good, all the followings not.
So the first question is : why ? and the second is : how to avoid that ?
Thanks for your help
python numba execution-time
I just started to use numba to improve performance of my programs. I have reduce the case that I will present
import numba as nb
import numpy as np
from time import time
def dt_max(U,f, eps=1e-5):
return np.min( np.abs( U ) / ( np.abs( f ) + eps ) )
@nb.njit(cache=True)
def fast_dt_max(U,f, eps=1e-5):
m=U[0]
if m<0 : m=-U[0]
for i in range(len(U)) :
v = abs(U[i]) / ( abs(f[i]) + eps )
if v < m : m = v
return m
N=100
Niter = int(1e5)
x=np.linspace(-50,50,N)
t0 = time()
for i in range(Niter):
dt_max(x,x)
print('numpy',time()-t0)
t0 = time()
for i in range(Niter):
fast_dt_max(x,x)
print('numba' ,time()-t0)
I execute this entire file in spyder under the following distribution :
Python 3.5.5 |Anaconda custom (64-bit)| (default, Apr 7 2018, 04:52:34) [MSC v.1900 64 bit (AMD64)]
Type "copyright", "credits" or "license" for more information.
The problem is the following. When I execute for the first time this program, it looks work good :
runfile('E:/02-Codes/TestCode/Python_numba/bug_second_execution.py', wdir='E:/02-Codes/TestCode/Python_numba')
numpy 0.45239996910095215
numba 0.2964000701904297
But when I repeat the execution of all file :
runfile('E:/02-Codes/TestCode/Python_numba/bug_second_execution.py', wdir='E:/02-Codes/TestCode/Python_numba')
numpy 0.45239996910095215
numba 3.5879998207092285
runfile('E:/02-Codes/TestCode/Python_numba/bug_second_execution.py', wdir='E:/02-Codes/TestCode/Python_numba')
numpy 0.4679999351501465
numba 3.5734000205993652
The numba's performance are not the same at all. If I restart Python kernel in my spyder environment, the problemn happens again : first execution is good, all the followings not.
So the first question is : why ? and the second is : how to avoid that ?
Thanks for your help
python numba execution-time
python numba execution-time
asked Nov 20 '18 at 8:40
lg53lg53
437
437
1
The problem seems to come from setting a default variable for eps. This causes a recompilation after reopening the interpreter. You can check this behaviour with setting the environment variable NUMBA_DEBUG_CACHE to one. eg. (set NUMBA_DEBUG_CACHE=1) and setting no default value for eps. If there are many cached versions for one function in the cache directory, this can also explain the very slow timing for loading the precached version.
– max9111
Nov 20 '18 at 10:15
add a comment |
1
The problem seems to come from setting a default variable for eps. This causes a recompilation after reopening the interpreter. You can check this behaviour with setting the environment variable NUMBA_DEBUG_CACHE to one. eg. (set NUMBA_DEBUG_CACHE=1) and setting no default value for eps. If there are many cached versions for one function in the cache directory, this can also explain the very slow timing for loading the precached version.
– max9111
Nov 20 '18 at 10:15
1
1
The problem seems to come from setting a default variable for eps. This causes a recompilation after reopening the interpreter. You can check this behaviour with setting the environment variable NUMBA_DEBUG_CACHE to one. eg. (set NUMBA_DEBUG_CACHE=1) and setting no default value for eps. If there are many cached versions for one function in the cache directory, this can also explain the very slow timing for loading the precached version.
– max9111
Nov 20 '18 at 10:15
The problem seems to come from setting a default variable for eps. This causes a recompilation after reopening the interpreter. You can check this behaviour with setting the environment variable NUMBA_DEBUG_CACHE to one. eg. (set NUMBA_DEBUG_CACHE=1) and setting no default value for eps. If there are many cached versions for one function in the cache directory, this can also explain the very slow timing for loading the precached version.
– max9111
Nov 20 '18 at 10:15
add a comment |
1 Answer
1
active
oldest
votes
Thanks a lot Max for the answer.
Indeed, the default value for eps is the reason of the problem. If I switch in debug mode (thanks Max for this tips!) :
import os
os.environ['NUMBA_DEBUG_CACHE'] = '1'
import numba as nb
import numpy as np
from time import time
@nb.njit(cache=True)
def fast_dt_max(U,f, eps=1e-5):
m=U[0]
if m<0 : m=-U[0]
for i in range(len(U)) :
v = abs(U[i]) / ( abs(f[i]) + eps )
if v < m : m = v
return m
This code shows that at each execution, 4 operations is done (2 loads, and 2 saving). Now if I remove the default value for eps, by keeping debug mode :
@nb.njit(cache=True)
def fast_dt_max(U,f, eps):
m=U[0]
if m<0 : m=-U[0]
for i in range(len(U)) :
v = abs(U[i]) / ( abs(f[i]) + eps )
if v < m : m = v
return m
the successive call to the function, let appear only 2 operations per execution (1 load + 1 saving)
CONCLUSION : Not use default parameter values in numba function.
Thanks !
BTW: Your Numba and Numpy version are not equal. If a division by zero occurs the Numba version will act by default like Python and raise an exception. In Numpy a division by zero results in inf and you will see a Runtime Warning. If you write @nb.njit(cache=True,error_model="numpy") Numba will behave like Numpy, but without a runtime warning.
– max9111
Nov 20 '18 at 16:00
What do you mean by "Numba and Numpy version are not equal" ? My numba version is 0.40.1 and my numpy version is 1.14.5.
– lg53
Nov 21 '18 at 9:11
Good tips for error_model="numpy", it let to treat without error case with eps=0. Thanks !
– lg53
Nov 21 '18 at 9:13
I meant that in case of a division by zero your Numba version raises an exception without setting error_model="numpy", while the numpy function doesn't. That can lead to unwanted behaviour.
– max9111
Nov 21 '18 at 9:23
ok, not equal in the meaning that not works identically. I understand, thanks.
– lg53
Nov 21 '18 at 14:52
add a comment |
Your Answer
StackExchange.ifUsing("editor", function () {
StackExchange.using("externalEditor", function () {
StackExchange.using("snippets", function () {
StackExchange.snippets.init();
});
});
}, "code-snippets");
StackExchange.ready(function() {
var channelOptions = {
tags: "".split(" "),
id: "1"
};
initTagRenderer("".split(" "), "".split(" "), channelOptions);
StackExchange.using("externalEditor", function() {
// Have to fire editor after snippets, if snippets enabled
if (StackExchange.settings.snippets.snippetsEnabled) {
StackExchange.using("snippets", function() {
createEditor();
});
}
else {
createEditor();
}
});
function createEditor() {
StackExchange.prepareEditor({
heartbeatType: 'answer',
autoActivateHeartbeat: false,
convertImagesToLinks: true,
noModals: true,
showLowRepImageUploadWarning: true,
reputationToPostImages: 10,
bindNavPrevention: true,
postfix: "",
imageUploader: {
brandingHtml: "Powered by u003ca class="icon-imgur-white" href="https://imgur.com/"u003eu003c/au003e",
contentPolicyHtml: "User contributions licensed under u003ca href="https://creativecommons.org/licenses/by-sa/3.0/"u003ecc by-sa 3.0 with attribution requiredu003c/au003e u003ca href="https://stackoverflow.com/legal/content-policy"u003e(content policy)u003c/au003e",
allowUrls: true
},
onDemand: true,
discardSelector: ".discard-answer"
,immediatelyShowMarkdownHelp:true
});
}
});
Sign up or log in
StackExchange.ready(function () {
StackExchange.helpers.onClickDraftSave('#login-link');
});
Sign up using Google
Sign up using Facebook
Sign up using Email and Password
Post as a guest
Required, but never shown
StackExchange.ready(
function () {
StackExchange.openid.initPostLogin('.new-post-login', 'https%3a%2f%2fstackoverflow.com%2fquestions%2f53389113%2fnumba-difference-between-first-time-execution-and-following-executions%23new-answer', 'question_page');
}
);
Post as a guest
Required, but never shown
1 Answer
1
active
oldest
votes
1 Answer
1
active
oldest
votes
active
oldest
votes
active
oldest
votes
Thanks a lot Max for the answer.
Indeed, the default value for eps is the reason of the problem. If I switch in debug mode (thanks Max for this tips!) :
import os
os.environ['NUMBA_DEBUG_CACHE'] = '1'
import numba as nb
import numpy as np
from time import time
@nb.njit(cache=True)
def fast_dt_max(U,f, eps=1e-5):
m=U[0]
if m<0 : m=-U[0]
for i in range(len(U)) :
v = abs(U[i]) / ( abs(f[i]) + eps )
if v < m : m = v
return m
This code shows that at each execution, 4 operations is done (2 loads, and 2 saving). Now if I remove the default value for eps, by keeping debug mode :
@nb.njit(cache=True)
def fast_dt_max(U,f, eps):
m=U[0]
if m<0 : m=-U[0]
for i in range(len(U)) :
v = abs(U[i]) / ( abs(f[i]) + eps )
if v < m : m = v
return m
the successive call to the function, let appear only 2 operations per execution (1 load + 1 saving)
CONCLUSION : Not use default parameter values in numba function.
Thanks !
BTW: Your Numba and Numpy version are not equal. If a division by zero occurs the Numba version will act by default like Python and raise an exception. In Numpy a division by zero results in inf and you will see a Runtime Warning. If you write @nb.njit(cache=True,error_model="numpy") Numba will behave like Numpy, but without a runtime warning.
– max9111
Nov 20 '18 at 16:00
What do you mean by "Numba and Numpy version are not equal" ? My numba version is 0.40.1 and my numpy version is 1.14.5.
– lg53
Nov 21 '18 at 9:11
Good tips for error_model="numpy", it let to treat without error case with eps=0. Thanks !
– lg53
Nov 21 '18 at 9:13
I meant that in case of a division by zero your Numba version raises an exception without setting error_model="numpy", while the numpy function doesn't. That can lead to unwanted behaviour.
– max9111
Nov 21 '18 at 9:23
ok, not equal in the meaning that not works identically. I understand, thanks.
– lg53
Nov 21 '18 at 14:52
add a comment |
Thanks a lot Max for the answer.
Indeed, the default value for eps is the reason of the problem. If I switch in debug mode (thanks Max for this tips!) :
import os
os.environ['NUMBA_DEBUG_CACHE'] = '1'
import numba as nb
import numpy as np
from time import time
@nb.njit(cache=True)
def fast_dt_max(U,f, eps=1e-5):
m=U[0]
if m<0 : m=-U[0]
for i in range(len(U)) :
v = abs(U[i]) / ( abs(f[i]) + eps )
if v < m : m = v
return m
This code shows that at each execution, 4 operations is done (2 loads, and 2 saving). Now if I remove the default value for eps, by keeping debug mode :
@nb.njit(cache=True)
def fast_dt_max(U,f, eps):
m=U[0]
if m<0 : m=-U[0]
for i in range(len(U)) :
v = abs(U[i]) / ( abs(f[i]) + eps )
if v < m : m = v
return m
the successive call to the function, let appear only 2 operations per execution (1 load + 1 saving)
CONCLUSION : Not use default parameter values in numba function.
Thanks !
BTW: Your Numba and Numpy version are not equal. If a division by zero occurs the Numba version will act by default like Python and raise an exception. In Numpy a division by zero results in inf and you will see a Runtime Warning. If you write @nb.njit(cache=True,error_model="numpy") Numba will behave like Numpy, but without a runtime warning.
– max9111
Nov 20 '18 at 16:00
What do you mean by "Numba and Numpy version are not equal" ? My numba version is 0.40.1 and my numpy version is 1.14.5.
– lg53
Nov 21 '18 at 9:11
Good tips for error_model="numpy", it let to treat without error case with eps=0. Thanks !
– lg53
Nov 21 '18 at 9:13
I meant that in case of a division by zero your Numba version raises an exception without setting error_model="numpy", while the numpy function doesn't. That can lead to unwanted behaviour.
– max9111
Nov 21 '18 at 9:23
ok, not equal in the meaning that not works identically. I understand, thanks.
– lg53
Nov 21 '18 at 14:52
add a comment |
Thanks a lot Max for the answer.
Indeed, the default value for eps is the reason of the problem. If I switch in debug mode (thanks Max for this tips!) :
import os
os.environ['NUMBA_DEBUG_CACHE'] = '1'
import numba as nb
import numpy as np
from time import time
@nb.njit(cache=True)
def fast_dt_max(U,f, eps=1e-5):
m=U[0]
if m<0 : m=-U[0]
for i in range(len(U)) :
v = abs(U[i]) / ( abs(f[i]) + eps )
if v < m : m = v
return m
This code shows that at each execution, 4 operations is done (2 loads, and 2 saving). Now if I remove the default value for eps, by keeping debug mode :
@nb.njit(cache=True)
def fast_dt_max(U,f, eps):
m=U[0]
if m<0 : m=-U[0]
for i in range(len(U)) :
v = abs(U[i]) / ( abs(f[i]) + eps )
if v < m : m = v
return m
the successive call to the function, let appear only 2 operations per execution (1 load + 1 saving)
CONCLUSION : Not use default parameter values in numba function.
Thanks !
Thanks a lot Max for the answer.
Indeed, the default value for eps is the reason of the problem. If I switch in debug mode (thanks Max for this tips!) :
import os
os.environ['NUMBA_DEBUG_CACHE'] = '1'
import numba as nb
import numpy as np
from time import time
@nb.njit(cache=True)
def fast_dt_max(U,f, eps=1e-5):
m=U[0]
if m<0 : m=-U[0]
for i in range(len(U)) :
v = abs(U[i]) / ( abs(f[i]) + eps )
if v < m : m = v
return m
This code shows that at each execution, 4 operations is done (2 loads, and 2 saving). Now if I remove the default value for eps, by keeping debug mode :
@nb.njit(cache=True)
def fast_dt_max(U,f, eps):
m=U[0]
if m<0 : m=-U[0]
for i in range(len(U)) :
v = abs(U[i]) / ( abs(f[i]) + eps )
if v < m : m = v
return m
the successive call to the function, let appear only 2 operations per execution (1 load + 1 saving)
CONCLUSION : Not use default parameter values in numba function.
Thanks !
answered Nov 20 '18 at 14:38
lg53lg53
437
437
BTW: Your Numba and Numpy version are not equal. If a division by zero occurs the Numba version will act by default like Python and raise an exception. In Numpy a division by zero results in inf and you will see a Runtime Warning. If you write @nb.njit(cache=True,error_model="numpy") Numba will behave like Numpy, but without a runtime warning.
– max9111
Nov 20 '18 at 16:00
What do you mean by "Numba and Numpy version are not equal" ? My numba version is 0.40.1 and my numpy version is 1.14.5.
– lg53
Nov 21 '18 at 9:11
Good tips for error_model="numpy", it let to treat without error case with eps=0. Thanks !
– lg53
Nov 21 '18 at 9:13
I meant that in case of a division by zero your Numba version raises an exception without setting error_model="numpy", while the numpy function doesn't. That can lead to unwanted behaviour.
– max9111
Nov 21 '18 at 9:23
ok, not equal in the meaning that not works identically. I understand, thanks.
– lg53
Nov 21 '18 at 14:52
add a comment |
BTW: Your Numba and Numpy version are not equal. If a division by zero occurs the Numba version will act by default like Python and raise an exception. In Numpy a division by zero results in inf and you will see a Runtime Warning. If you write @nb.njit(cache=True,error_model="numpy") Numba will behave like Numpy, but without a runtime warning.
– max9111
Nov 20 '18 at 16:00
What do you mean by "Numba and Numpy version are not equal" ? My numba version is 0.40.1 and my numpy version is 1.14.5.
– lg53
Nov 21 '18 at 9:11
Good tips for error_model="numpy", it let to treat without error case with eps=0. Thanks !
– lg53
Nov 21 '18 at 9:13
I meant that in case of a division by zero your Numba version raises an exception without setting error_model="numpy", while the numpy function doesn't. That can lead to unwanted behaviour.
– max9111
Nov 21 '18 at 9:23
ok, not equal in the meaning that not works identically. I understand, thanks.
– lg53
Nov 21 '18 at 14:52
BTW: Your Numba and Numpy version are not equal. If a division by zero occurs the Numba version will act by default like Python and raise an exception. In Numpy a division by zero results in inf and you will see a Runtime Warning. If you write @nb.njit(cache=True,error_model="numpy") Numba will behave like Numpy, but without a runtime warning.
– max9111
Nov 20 '18 at 16:00
BTW: Your Numba and Numpy version are not equal. If a division by zero occurs the Numba version will act by default like Python and raise an exception. In Numpy a division by zero results in inf and you will see a Runtime Warning. If you write @nb.njit(cache=True,error_model="numpy") Numba will behave like Numpy, but without a runtime warning.
– max9111
Nov 20 '18 at 16:00
What do you mean by "Numba and Numpy version are not equal" ? My numba version is 0.40.1 and my numpy version is 1.14.5.
– lg53
Nov 21 '18 at 9:11
What do you mean by "Numba and Numpy version are not equal" ? My numba version is 0.40.1 and my numpy version is 1.14.5.
– lg53
Nov 21 '18 at 9:11
Good tips for error_model="numpy", it let to treat without error case with eps=0. Thanks !
– lg53
Nov 21 '18 at 9:13
Good tips for error_model="numpy", it let to treat without error case with eps=0. Thanks !
– lg53
Nov 21 '18 at 9:13
I meant that in case of a division by zero your Numba version raises an exception without setting error_model="numpy", while the numpy function doesn't. That can lead to unwanted behaviour.
– max9111
Nov 21 '18 at 9:23
I meant that in case of a division by zero your Numba version raises an exception without setting error_model="numpy", while the numpy function doesn't. That can lead to unwanted behaviour.
– max9111
Nov 21 '18 at 9:23
ok, not equal in the meaning that not works identically. I understand, thanks.
– lg53
Nov 21 '18 at 14:52
ok, not equal in the meaning that not works identically. I understand, thanks.
– lg53
Nov 21 '18 at 14:52
add a comment |
Thanks for contributing an answer to Stack Overflow!
- Please be sure to answer the question. Provide details and share your research!
But avoid …
- Asking for help, clarification, or responding to other answers.
- Making statements based on opinion; back them up with references or personal experience.
To learn more, see our tips on writing great answers.
Sign up or log in
StackExchange.ready(function () {
StackExchange.helpers.onClickDraftSave('#login-link');
});
Sign up using Google
Sign up using Facebook
Sign up using Email and Password
Post as a guest
Required, but never shown
StackExchange.ready(
function () {
StackExchange.openid.initPostLogin('.new-post-login', 'https%3a%2f%2fstackoverflow.com%2fquestions%2f53389113%2fnumba-difference-between-first-time-execution-and-following-executions%23new-answer', 'question_page');
}
);
Post as a guest
Required, but never shown
Sign up or log in
StackExchange.ready(function () {
StackExchange.helpers.onClickDraftSave('#login-link');
});
Sign up using Google
Sign up using Facebook
Sign up using Email and Password
Post as a guest
Required, but never shown
Sign up or log in
StackExchange.ready(function () {
StackExchange.helpers.onClickDraftSave('#login-link');
});
Sign up using Google
Sign up using Facebook
Sign up using Email and Password
Post as a guest
Required, but never shown
Sign up or log in
StackExchange.ready(function () {
StackExchange.helpers.onClickDraftSave('#login-link');
});
Sign up using Google
Sign up using Facebook
Sign up using Email and Password
Sign up using Google
Sign up using Facebook
Sign up using Email and Password
Post as a guest
Required, but never shown
Required, but never shown
Required, but never shown
Required, but never shown
Required, but never shown
Required, but never shown
Required, but never shown
Required, but never shown
Required, but never shown
yk,z bZZPgrb r,jnwC3stMlFdaQkOJkV
1
The problem seems to come from setting a default variable for eps. This causes a recompilation after reopening the interpreter. You can check this behaviour with setting the environment variable NUMBA_DEBUG_CACHE to one. eg. (set NUMBA_DEBUG_CACHE=1) and setting no default value for eps. If there are many cached versions for one function in the cache directory, this can also explain the very slow timing for loading the precached version.
– max9111
Nov 20 '18 at 10:15