Fréchet mean for a general shape space
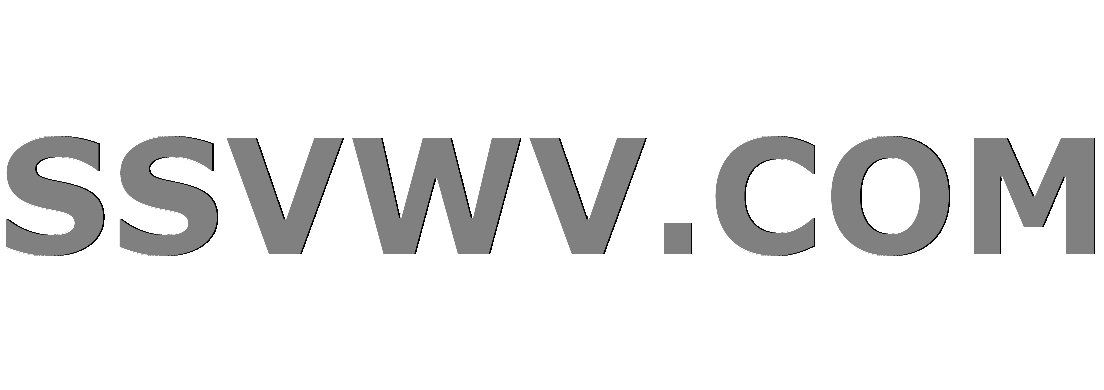
Multi tool use
up vote
1
down vote
favorite
I am posting this question in order to gain a better understand of what the Fréchet mean is for a generalised shape space.
So firstly I gather that the Fréchet mean of a probabilty measure $mu$ on a general metric space $(M,dist)$ is a generalisation of the mean or the expectation of a probability distribution on a Euclidean space and is defined as any global minimum of the function
$$F(x)=int_Mdist(x,y)^2dmu(y).$$
This concept can be further generalised by replacing 'dist' in the integrand by a suitable function of the distance.
My question is, what exactly does this formula do? I think it has something to do with minimising the mean between a data set, however I am not sure of this. Also I am not sure how I would use this formula. What exactly is the 'probability measure' in this case? I think it's some kind of statistical distribution, but again I am really not sure.
Any help with this question will be much appreiciated, thank you.
probability general-topology complex-analysis metric-spaces
add a comment |
up vote
1
down vote
favorite
I am posting this question in order to gain a better understand of what the Fréchet mean is for a generalised shape space.
So firstly I gather that the Fréchet mean of a probabilty measure $mu$ on a general metric space $(M,dist)$ is a generalisation of the mean or the expectation of a probability distribution on a Euclidean space and is defined as any global minimum of the function
$$F(x)=int_Mdist(x,y)^2dmu(y).$$
This concept can be further generalised by replacing 'dist' in the integrand by a suitable function of the distance.
My question is, what exactly does this formula do? I think it has something to do with minimising the mean between a data set, however I am not sure of this. Also I am not sure how I would use this formula. What exactly is the 'probability measure' in this case? I think it's some kind of statistical distribution, but again I am really not sure.
Any help with this question will be much appreiciated, thank you.
probability general-topology complex-analysis metric-spaces
If $x^*$ is an argument of a global minimum of $F$, then we know that the $mu$-average distance from $x^*$ to any point $yin M$ is minimal.
– Ilya
Jan 26 '13 at 21:45
Thank you, so $x^*$ is the argmin of F, would we consider $y$ to be the argmin of another data set? And what exactly is the $mu$-average? I.e. what is a probability measure?
– Luis_G
Jan 26 '13 at 21:55
In order to have any concept of a mean we need a probability measure. When finding the mean of numbers $1,2,3,4$ we (implicitly) use the probability measure $mu$ such that $mu({k})=1/4$ for $k=1,2,3,4$. So, if you need the mean of a finite subset of a metric space, using this soft of measure (normalized counting measure) may be appropriate. If the set is infinite, one has to think harder of what the appropriate measure should be.
– user53153
Jan 27 '13 at 0:33
add a comment |
up vote
1
down vote
favorite
up vote
1
down vote
favorite
I am posting this question in order to gain a better understand of what the Fréchet mean is for a generalised shape space.
So firstly I gather that the Fréchet mean of a probabilty measure $mu$ on a general metric space $(M,dist)$ is a generalisation of the mean or the expectation of a probability distribution on a Euclidean space and is defined as any global minimum of the function
$$F(x)=int_Mdist(x,y)^2dmu(y).$$
This concept can be further generalised by replacing 'dist' in the integrand by a suitable function of the distance.
My question is, what exactly does this formula do? I think it has something to do with minimising the mean between a data set, however I am not sure of this. Also I am not sure how I would use this formula. What exactly is the 'probability measure' in this case? I think it's some kind of statistical distribution, but again I am really not sure.
Any help with this question will be much appreiciated, thank you.
probability general-topology complex-analysis metric-spaces
I am posting this question in order to gain a better understand of what the Fréchet mean is for a generalised shape space.
So firstly I gather that the Fréchet mean of a probabilty measure $mu$ on a general metric space $(M,dist)$ is a generalisation of the mean or the expectation of a probability distribution on a Euclidean space and is defined as any global minimum of the function
$$F(x)=int_Mdist(x,y)^2dmu(y).$$
This concept can be further generalised by replacing 'dist' in the integrand by a suitable function of the distance.
My question is, what exactly does this formula do? I think it has something to do with minimising the mean between a data set, however I am not sure of this. Also I am not sure how I would use this formula. What exactly is the 'probability measure' in this case? I think it's some kind of statistical distribution, but again I am really not sure.
Any help with this question will be much appreiciated, thank you.
probability general-topology complex-analysis metric-spaces
probability general-topology complex-analysis metric-spaces
edited Jan 27 '13 at 20:20
asked Jan 26 '13 at 21:39
Luis_G
7418
7418
If $x^*$ is an argument of a global minimum of $F$, then we know that the $mu$-average distance from $x^*$ to any point $yin M$ is minimal.
– Ilya
Jan 26 '13 at 21:45
Thank you, so $x^*$ is the argmin of F, would we consider $y$ to be the argmin of another data set? And what exactly is the $mu$-average? I.e. what is a probability measure?
– Luis_G
Jan 26 '13 at 21:55
In order to have any concept of a mean we need a probability measure. When finding the mean of numbers $1,2,3,4$ we (implicitly) use the probability measure $mu$ such that $mu({k})=1/4$ for $k=1,2,3,4$. So, if you need the mean of a finite subset of a metric space, using this soft of measure (normalized counting measure) may be appropriate. If the set is infinite, one has to think harder of what the appropriate measure should be.
– user53153
Jan 27 '13 at 0:33
add a comment |
If $x^*$ is an argument of a global minimum of $F$, then we know that the $mu$-average distance from $x^*$ to any point $yin M$ is minimal.
– Ilya
Jan 26 '13 at 21:45
Thank you, so $x^*$ is the argmin of F, would we consider $y$ to be the argmin of another data set? And what exactly is the $mu$-average? I.e. what is a probability measure?
– Luis_G
Jan 26 '13 at 21:55
In order to have any concept of a mean we need a probability measure. When finding the mean of numbers $1,2,3,4$ we (implicitly) use the probability measure $mu$ such that $mu({k})=1/4$ for $k=1,2,3,4$. So, if you need the mean of a finite subset of a metric space, using this soft of measure (normalized counting measure) may be appropriate. If the set is infinite, one has to think harder of what the appropriate measure should be.
– user53153
Jan 27 '13 at 0:33
If $x^*$ is an argument of a global minimum of $F$, then we know that the $mu$-average distance from $x^*$ to any point $yin M$ is minimal.
– Ilya
Jan 26 '13 at 21:45
If $x^*$ is an argument of a global minimum of $F$, then we know that the $mu$-average distance from $x^*$ to any point $yin M$ is minimal.
– Ilya
Jan 26 '13 at 21:45
Thank you, so $x^*$ is the argmin of F, would we consider $y$ to be the argmin of another data set? And what exactly is the $mu$-average? I.e. what is a probability measure?
– Luis_G
Jan 26 '13 at 21:55
Thank you, so $x^*$ is the argmin of F, would we consider $y$ to be the argmin of another data set? And what exactly is the $mu$-average? I.e. what is a probability measure?
– Luis_G
Jan 26 '13 at 21:55
In order to have any concept of a mean we need a probability measure. When finding the mean of numbers $1,2,3,4$ we (implicitly) use the probability measure $mu$ such that $mu({k})=1/4$ for $k=1,2,3,4$. So, if you need the mean of a finite subset of a metric space, using this soft of measure (normalized counting measure) may be appropriate. If the set is infinite, one has to think harder of what the appropriate measure should be.
– user53153
Jan 27 '13 at 0:33
In order to have any concept of a mean we need a probability measure. When finding the mean of numbers $1,2,3,4$ we (implicitly) use the probability measure $mu$ such that $mu({k})=1/4$ for $k=1,2,3,4$. So, if you need the mean of a finite subset of a metric space, using this soft of measure (normalized counting measure) may be appropriate. If the set is infinite, one has to think harder of what the appropriate measure should be.
– user53153
Jan 27 '13 at 0:33
add a comment |
1 Answer
1
active
oldest
votes
up vote
0
down vote
It is always useful to consider a simple example. For example, consider the uniform distribution on $M = [0,1]$ and Euclidean metric $d(x,y) = ||x-y||$. We know that the (usual) mean is $1/2$. So let's check. We aim at minimizing
$$
F(x) = int_0^1 ||x-y||^2 dy = int_0^1 (x-y)^2 dy = x^2y-xy^2+y^3/3 big|_{y=0}^1 = x^2 - x.
$$
It is easy to see that this function attains it's minimum at $x=1/2$.
More general, if the distribution has probability density $p$, we want to minimize
$$
F(x) = int_M ||x-y||^2 p(y) dy
$$
We compute the minimum by setting the derivative to zero:
$$
d/dx F(x) = int_M d/dx ||x-y||^2 p(y) dy = int_M 2(x-y) p(y) dy \= 2 x int_M p(y) dy - 2int_M y p(y) dy = 2x - 2int_M y p(y) dy = 0
$$
The last equality is equivalent to
$$
x = int_M y p(y) dy
$$
Hence, we have shown that if $d$ is the Euclidean metric, the Fréchet mean of a distribution with density $p$ coincides with the usual mean of this distribution $x = int_M y p(y) dy$
Even more general, there need not be a density, you may want to use the abstract formulation using a probability measure $mu$
$$
F(x) = int_M ||x-y||^2 d mu(y)
$$
add a comment |
Your Answer
StackExchange.ifUsing("editor", function () {
return StackExchange.using("mathjaxEditing", function () {
StackExchange.MarkdownEditor.creationCallbacks.add(function (editor, postfix) {
StackExchange.mathjaxEditing.prepareWmdForMathJax(editor, postfix, [["$", "$"], ["\\(","\\)"]]);
});
});
}, "mathjax-editing");
StackExchange.ready(function() {
var channelOptions = {
tags: "".split(" "),
id: "69"
};
initTagRenderer("".split(" "), "".split(" "), channelOptions);
StackExchange.using("externalEditor", function() {
// Have to fire editor after snippets, if snippets enabled
if (StackExchange.settings.snippets.snippetsEnabled) {
StackExchange.using("snippets", function() {
createEditor();
});
}
else {
createEditor();
}
});
function createEditor() {
StackExchange.prepareEditor({
heartbeatType: 'answer',
convertImagesToLinks: true,
noModals: true,
showLowRepImageUploadWarning: true,
reputationToPostImages: 10,
bindNavPrevention: true,
postfix: "",
imageUploader: {
brandingHtml: "Powered by u003ca class="icon-imgur-white" href="https://imgur.com/"u003eu003c/au003e",
contentPolicyHtml: "User contributions licensed under u003ca href="https://creativecommons.org/licenses/by-sa/3.0/"u003ecc by-sa 3.0 with attribution requiredu003c/au003e u003ca href="https://stackoverflow.com/legal/content-policy"u003e(content policy)u003c/au003e",
allowUrls: true
},
noCode: true, onDemand: true,
discardSelector: ".discard-answer"
,immediatelyShowMarkdownHelp:true
});
}
});
Sign up or log in
StackExchange.ready(function () {
StackExchange.helpers.onClickDraftSave('#login-link');
});
Sign up using Google
Sign up using Facebook
Sign up using Email and Password
Post as a guest
Required, but never shown
StackExchange.ready(
function () {
StackExchange.openid.initPostLogin('.new-post-login', 'https%3a%2f%2fmath.stackexchange.com%2fquestions%2f287629%2ffr%25c3%25a9chet-mean-for-a-general-shape-space%23new-answer', 'question_page');
}
);
Post as a guest
Required, but never shown
1 Answer
1
active
oldest
votes
1 Answer
1
active
oldest
votes
active
oldest
votes
active
oldest
votes
up vote
0
down vote
It is always useful to consider a simple example. For example, consider the uniform distribution on $M = [0,1]$ and Euclidean metric $d(x,y) = ||x-y||$. We know that the (usual) mean is $1/2$. So let's check. We aim at minimizing
$$
F(x) = int_0^1 ||x-y||^2 dy = int_0^1 (x-y)^2 dy = x^2y-xy^2+y^3/3 big|_{y=0}^1 = x^2 - x.
$$
It is easy to see that this function attains it's minimum at $x=1/2$.
More general, if the distribution has probability density $p$, we want to minimize
$$
F(x) = int_M ||x-y||^2 p(y) dy
$$
We compute the minimum by setting the derivative to zero:
$$
d/dx F(x) = int_M d/dx ||x-y||^2 p(y) dy = int_M 2(x-y) p(y) dy \= 2 x int_M p(y) dy - 2int_M y p(y) dy = 2x - 2int_M y p(y) dy = 0
$$
The last equality is equivalent to
$$
x = int_M y p(y) dy
$$
Hence, we have shown that if $d$ is the Euclidean metric, the Fréchet mean of a distribution with density $p$ coincides with the usual mean of this distribution $x = int_M y p(y) dy$
Even more general, there need not be a density, you may want to use the abstract formulation using a probability measure $mu$
$$
F(x) = int_M ||x-y||^2 d mu(y)
$$
add a comment |
up vote
0
down vote
It is always useful to consider a simple example. For example, consider the uniform distribution on $M = [0,1]$ and Euclidean metric $d(x,y) = ||x-y||$. We know that the (usual) mean is $1/2$. So let's check. We aim at minimizing
$$
F(x) = int_0^1 ||x-y||^2 dy = int_0^1 (x-y)^2 dy = x^2y-xy^2+y^3/3 big|_{y=0}^1 = x^2 - x.
$$
It is easy to see that this function attains it's minimum at $x=1/2$.
More general, if the distribution has probability density $p$, we want to minimize
$$
F(x) = int_M ||x-y||^2 p(y) dy
$$
We compute the minimum by setting the derivative to zero:
$$
d/dx F(x) = int_M d/dx ||x-y||^2 p(y) dy = int_M 2(x-y) p(y) dy \= 2 x int_M p(y) dy - 2int_M y p(y) dy = 2x - 2int_M y p(y) dy = 0
$$
The last equality is equivalent to
$$
x = int_M y p(y) dy
$$
Hence, we have shown that if $d$ is the Euclidean metric, the Fréchet mean of a distribution with density $p$ coincides with the usual mean of this distribution $x = int_M y p(y) dy$
Even more general, there need not be a density, you may want to use the abstract formulation using a probability measure $mu$
$$
F(x) = int_M ||x-y||^2 d mu(y)
$$
add a comment |
up vote
0
down vote
up vote
0
down vote
It is always useful to consider a simple example. For example, consider the uniform distribution on $M = [0,1]$ and Euclidean metric $d(x,y) = ||x-y||$. We know that the (usual) mean is $1/2$. So let's check. We aim at minimizing
$$
F(x) = int_0^1 ||x-y||^2 dy = int_0^1 (x-y)^2 dy = x^2y-xy^2+y^3/3 big|_{y=0}^1 = x^2 - x.
$$
It is easy to see that this function attains it's minimum at $x=1/2$.
More general, if the distribution has probability density $p$, we want to minimize
$$
F(x) = int_M ||x-y||^2 p(y) dy
$$
We compute the minimum by setting the derivative to zero:
$$
d/dx F(x) = int_M d/dx ||x-y||^2 p(y) dy = int_M 2(x-y) p(y) dy \= 2 x int_M p(y) dy - 2int_M y p(y) dy = 2x - 2int_M y p(y) dy = 0
$$
The last equality is equivalent to
$$
x = int_M y p(y) dy
$$
Hence, we have shown that if $d$ is the Euclidean metric, the Fréchet mean of a distribution with density $p$ coincides with the usual mean of this distribution $x = int_M y p(y) dy$
Even more general, there need not be a density, you may want to use the abstract formulation using a probability measure $mu$
$$
F(x) = int_M ||x-y||^2 d mu(y)
$$
It is always useful to consider a simple example. For example, consider the uniform distribution on $M = [0,1]$ and Euclidean metric $d(x,y) = ||x-y||$. We know that the (usual) mean is $1/2$. So let's check. We aim at minimizing
$$
F(x) = int_0^1 ||x-y||^2 dy = int_0^1 (x-y)^2 dy = x^2y-xy^2+y^3/3 big|_{y=0}^1 = x^2 - x.
$$
It is easy to see that this function attains it's minimum at $x=1/2$.
More general, if the distribution has probability density $p$, we want to minimize
$$
F(x) = int_M ||x-y||^2 p(y) dy
$$
We compute the minimum by setting the derivative to zero:
$$
d/dx F(x) = int_M d/dx ||x-y||^2 p(y) dy = int_M 2(x-y) p(y) dy \= 2 x int_M p(y) dy - 2int_M y p(y) dy = 2x - 2int_M y p(y) dy = 0
$$
The last equality is equivalent to
$$
x = int_M y p(y) dy
$$
Hence, we have shown that if $d$ is the Euclidean metric, the Fréchet mean of a distribution with density $p$ coincides with the usual mean of this distribution $x = int_M y p(y) dy$
Even more general, there need not be a density, you may want to use the abstract formulation using a probability measure $mu$
$$
F(x) = int_M ||x-y||^2 d mu(y)
$$
answered Nov 19 at 22:54
powermod
1
1
add a comment |
add a comment |
Thanks for contributing an answer to Mathematics Stack Exchange!
- Please be sure to answer the question. Provide details and share your research!
But avoid …
- Asking for help, clarification, or responding to other answers.
- Making statements based on opinion; back them up with references or personal experience.
Use MathJax to format equations. MathJax reference.
To learn more, see our tips on writing great answers.
Some of your past answers have not been well-received, and you're in danger of being blocked from answering.
Please pay close attention to the following guidance:
- Please be sure to answer the question. Provide details and share your research!
But avoid …
- Asking for help, clarification, or responding to other answers.
- Making statements based on opinion; back them up with references or personal experience.
To learn more, see our tips on writing great answers.
Sign up or log in
StackExchange.ready(function () {
StackExchange.helpers.onClickDraftSave('#login-link');
});
Sign up using Google
Sign up using Facebook
Sign up using Email and Password
Post as a guest
Required, but never shown
StackExchange.ready(
function () {
StackExchange.openid.initPostLogin('.new-post-login', 'https%3a%2f%2fmath.stackexchange.com%2fquestions%2f287629%2ffr%25c3%25a9chet-mean-for-a-general-shape-space%23new-answer', 'question_page');
}
);
Post as a guest
Required, but never shown
Sign up or log in
StackExchange.ready(function () {
StackExchange.helpers.onClickDraftSave('#login-link');
});
Sign up using Google
Sign up using Facebook
Sign up using Email and Password
Post as a guest
Required, but never shown
Sign up or log in
StackExchange.ready(function () {
StackExchange.helpers.onClickDraftSave('#login-link');
});
Sign up using Google
Sign up using Facebook
Sign up using Email and Password
Post as a guest
Required, but never shown
Sign up or log in
StackExchange.ready(function () {
StackExchange.helpers.onClickDraftSave('#login-link');
});
Sign up using Google
Sign up using Facebook
Sign up using Email and Password
Sign up using Google
Sign up using Facebook
Sign up using Email and Password
Post as a guest
Required, but never shown
Required, but never shown
Required, but never shown
Required, but never shown
Required, but never shown
Required, but never shown
Required, but never shown
Required, but never shown
Required, but never shown
Q2bj3Jsz,7IM1E,P3,GmRYxGCxnJ1Mhnx R41,K57NVV3Dn5c,UGPyG,EeGiJV1Y cv89rsJWCf,MTJmBT9,pYocggQt iM gIUVT MDQn1cHy
If $x^*$ is an argument of a global minimum of $F$, then we know that the $mu$-average distance from $x^*$ to any point $yin M$ is minimal.
– Ilya
Jan 26 '13 at 21:45
Thank you, so $x^*$ is the argmin of F, would we consider $y$ to be the argmin of another data set? And what exactly is the $mu$-average? I.e. what is a probability measure?
– Luis_G
Jan 26 '13 at 21:55
In order to have any concept of a mean we need a probability measure. When finding the mean of numbers $1,2,3,4$ we (implicitly) use the probability measure $mu$ such that $mu({k})=1/4$ for $k=1,2,3,4$. So, if you need the mean of a finite subset of a metric space, using this soft of measure (normalized counting measure) may be appropriate. If the set is infinite, one has to think harder of what the appropriate measure should be.
– user53153
Jan 27 '13 at 0:33