Large and ill-conditioned quadratic convex problem
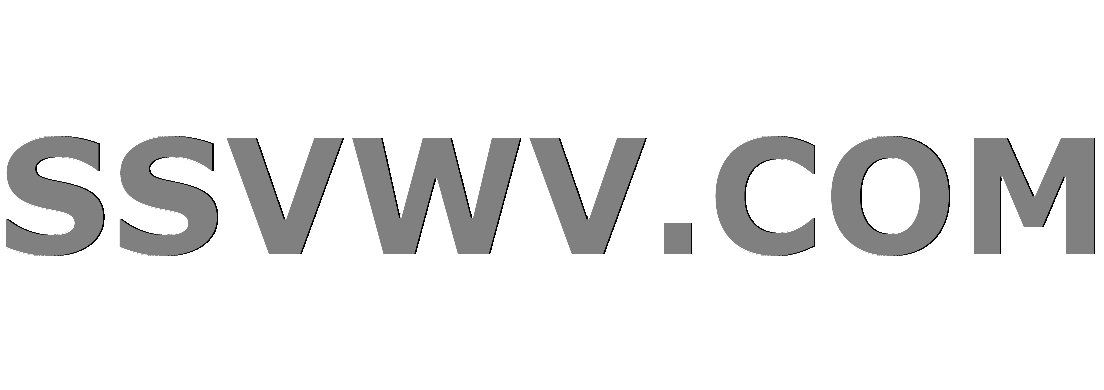
Multi tool use
up vote
1
down vote
favorite
I need to solve a convex quadratic problem numerically:
$min f(x) = frac{1}{2} x^top A x - b^top x$,
where $A$ is a very large and ill-conditioned semi positive definite matrix. Typical conjugate gradient method doesn't work well. SGD is too slow.
I'm looking for a good numerical method with relatively less computational effort.
Any experience or suggestions? Thank you!
numerical-linear-algebra
add a comment |
up vote
1
down vote
favorite
I need to solve a convex quadratic problem numerically:
$min f(x) = frac{1}{2} x^top A x - b^top x$,
where $A$ is a very large and ill-conditioned semi positive definite matrix. Typical conjugate gradient method doesn't work well. SGD is too slow.
I'm looking for a good numerical method with relatively less computational effort.
Any experience or suggestions? Thank you!
numerical-linear-algebra
add a comment |
up vote
1
down vote
favorite
up vote
1
down vote
favorite
I need to solve a convex quadratic problem numerically:
$min f(x) = frac{1}{2} x^top A x - b^top x$,
where $A$ is a very large and ill-conditioned semi positive definite matrix. Typical conjugate gradient method doesn't work well. SGD is too slow.
I'm looking for a good numerical method with relatively less computational effort.
Any experience or suggestions? Thank you!
numerical-linear-algebra
I need to solve a convex quadratic problem numerically:
$min f(x) = frac{1}{2} x^top A x - b^top x$,
where $A$ is a very large and ill-conditioned semi positive definite matrix. Typical conjugate gradient method doesn't work well. SGD is too slow.
I'm looking for a good numerical method with relatively less computational effort.
Any experience or suggestions? Thank you!
numerical-linear-algebra
numerical-linear-algebra
edited Nov 18 at 1:23
asked Nov 17 at 21:11
Joe Lacey
112
112
add a comment |
add a comment |
1 Answer
1
active
oldest
votes
up vote
0
down vote
I assume you are looking to minimize $f(x)$, and that $A$ is symmetric and positive semi-definite.
If $b notin Null(A)^{perp}$ then there is a vector $v in Null(A)$ such that $b^Tv neq 0$. Without loss of generality assume $b^Tv >0$ (else multiply $v$ by $-1$). Then for all real numbers $theta$ we get:
$$ f(theta v) = 0 + (theta)(-b^Tv)$$
so $lim_{thetarightarrowinfty} f(theta v) = -infty$ and no minimizer exists.
Else, $b in Null(A)^{perp} = Col(A^T) = Col(A)$. Just find any vector $r$ such that $Ar=b$. Then:
begin{align}
frac{1}{2}(x-r)^TA(x-r) &= frac{1}{2}x^TAx - x^TAr + frac{1}{2}r^TAr \
&= f(x) + frac{1}{2}r^TAr
end{align}
and this is clearly minimized at $x^*=r$, so
$$ f(x^*) = f(r) = -frac{1}{2}r^TAr$$
In other words, the problem reduces to Gaussian elimination: Find a vector $r$ such that $Ar=b$. If no such $r$ exists then no minimizer exists.
I completely agree with you for theoretical part. The problem is that we are facing a large ill-conditioned matrix. The goal is to find a good approximate solution. I should make the question clearer. Thanks
– Joe Lacey
Nov 18 at 1:25
add a comment |
1 Answer
1
active
oldest
votes
1 Answer
1
active
oldest
votes
active
oldest
votes
active
oldest
votes
up vote
0
down vote
I assume you are looking to minimize $f(x)$, and that $A$ is symmetric and positive semi-definite.
If $b notin Null(A)^{perp}$ then there is a vector $v in Null(A)$ such that $b^Tv neq 0$. Without loss of generality assume $b^Tv >0$ (else multiply $v$ by $-1$). Then for all real numbers $theta$ we get:
$$ f(theta v) = 0 + (theta)(-b^Tv)$$
so $lim_{thetarightarrowinfty} f(theta v) = -infty$ and no minimizer exists.
Else, $b in Null(A)^{perp} = Col(A^T) = Col(A)$. Just find any vector $r$ such that $Ar=b$. Then:
begin{align}
frac{1}{2}(x-r)^TA(x-r) &= frac{1}{2}x^TAx - x^TAr + frac{1}{2}r^TAr \
&= f(x) + frac{1}{2}r^TAr
end{align}
and this is clearly minimized at $x^*=r$, so
$$ f(x^*) = f(r) = -frac{1}{2}r^TAr$$
In other words, the problem reduces to Gaussian elimination: Find a vector $r$ such that $Ar=b$. If no such $r$ exists then no minimizer exists.
I completely agree with you for theoretical part. The problem is that we are facing a large ill-conditioned matrix. The goal is to find a good approximate solution. I should make the question clearer. Thanks
– Joe Lacey
Nov 18 at 1:25
add a comment |
up vote
0
down vote
I assume you are looking to minimize $f(x)$, and that $A$ is symmetric and positive semi-definite.
If $b notin Null(A)^{perp}$ then there is a vector $v in Null(A)$ such that $b^Tv neq 0$. Without loss of generality assume $b^Tv >0$ (else multiply $v$ by $-1$). Then for all real numbers $theta$ we get:
$$ f(theta v) = 0 + (theta)(-b^Tv)$$
so $lim_{thetarightarrowinfty} f(theta v) = -infty$ and no minimizer exists.
Else, $b in Null(A)^{perp} = Col(A^T) = Col(A)$. Just find any vector $r$ such that $Ar=b$. Then:
begin{align}
frac{1}{2}(x-r)^TA(x-r) &= frac{1}{2}x^TAx - x^TAr + frac{1}{2}r^TAr \
&= f(x) + frac{1}{2}r^TAr
end{align}
and this is clearly minimized at $x^*=r$, so
$$ f(x^*) = f(r) = -frac{1}{2}r^TAr$$
In other words, the problem reduces to Gaussian elimination: Find a vector $r$ such that $Ar=b$. If no such $r$ exists then no minimizer exists.
I completely agree with you for theoretical part. The problem is that we are facing a large ill-conditioned matrix. The goal is to find a good approximate solution. I should make the question clearer. Thanks
– Joe Lacey
Nov 18 at 1:25
add a comment |
up vote
0
down vote
up vote
0
down vote
I assume you are looking to minimize $f(x)$, and that $A$ is symmetric and positive semi-definite.
If $b notin Null(A)^{perp}$ then there is a vector $v in Null(A)$ such that $b^Tv neq 0$. Without loss of generality assume $b^Tv >0$ (else multiply $v$ by $-1$). Then for all real numbers $theta$ we get:
$$ f(theta v) = 0 + (theta)(-b^Tv)$$
so $lim_{thetarightarrowinfty} f(theta v) = -infty$ and no minimizer exists.
Else, $b in Null(A)^{perp} = Col(A^T) = Col(A)$. Just find any vector $r$ such that $Ar=b$. Then:
begin{align}
frac{1}{2}(x-r)^TA(x-r) &= frac{1}{2}x^TAx - x^TAr + frac{1}{2}r^TAr \
&= f(x) + frac{1}{2}r^TAr
end{align}
and this is clearly minimized at $x^*=r$, so
$$ f(x^*) = f(r) = -frac{1}{2}r^TAr$$
In other words, the problem reduces to Gaussian elimination: Find a vector $r$ such that $Ar=b$. If no such $r$ exists then no minimizer exists.
I assume you are looking to minimize $f(x)$, and that $A$ is symmetric and positive semi-definite.
If $b notin Null(A)^{perp}$ then there is a vector $v in Null(A)$ such that $b^Tv neq 0$. Without loss of generality assume $b^Tv >0$ (else multiply $v$ by $-1$). Then for all real numbers $theta$ we get:
$$ f(theta v) = 0 + (theta)(-b^Tv)$$
so $lim_{thetarightarrowinfty} f(theta v) = -infty$ and no minimizer exists.
Else, $b in Null(A)^{perp} = Col(A^T) = Col(A)$. Just find any vector $r$ such that $Ar=b$. Then:
begin{align}
frac{1}{2}(x-r)^TA(x-r) &= frac{1}{2}x^TAx - x^TAr + frac{1}{2}r^TAr \
&= f(x) + frac{1}{2}r^TAr
end{align}
and this is clearly minimized at $x^*=r$, so
$$ f(x^*) = f(r) = -frac{1}{2}r^TAr$$
In other words, the problem reduces to Gaussian elimination: Find a vector $r$ such that $Ar=b$. If no such $r$ exists then no minimizer exists.
edited Nov 17 at 22:54
answered Nov 17 at 22:49
Michael
13.2k11325
13.2k11325
I completely agree with you for theoretical part. The problem is that we are facing a large ill-conditioned matrix. The goal is to find a good approximate solution. I should make the question clearer. Thanks
– Joe Lacey
Nov 18 at 1:25
add a comment |
I completely agree with you for theoretical part. The problem is that we are facing a large ill-conditioned matrix. The goal is to find a good approximate solution. I should make the question clearer. Thanks
– Joe Lacey
Nov 18 at 1:25
I completely agree with you for theoretical part. The problem is that we are facing a large ill-conditioned matrix. The goal is to find a good approximate solution. I should make the question clearer. Thanks
– Joe Lacey
Nov 18 at 1:25
I completely agree with you for theoretical part. The problem is that we are facing a large ill-conditioned matrix. The goal is to find a good approximate solution. I should make the question clearer. Thanks
– Joe Lacey
Nov 18 at 1:25
add a comment |
Thanks for contributing an answer to Mathematics Stack Exchange!
- Please be sure to answer the question. Provide details and share your research!
But avoid …
- Asking for help, clarification, or responding to other answers.
- Making statements based on opinion; back them up with references or personal experience.
Use MathJax to format equations. MathJax reference.
To learn more, see our tips on writing great answers.
Some of your past answers have not been well-received, and you're in danger of being blocked from answering.
Please pay close attention to the following guidance:
- Please be sure to answer the question. Provide details and share your research!
But avoid …
- Asking for help, clarification, or responding to other answers.
- Making statements based on opinion; back them up with references or personal experience.
To learn more, see our tips on writing great answers.
Sign up or log in
StackExchange.ready(function () {
StackExchange.helpers.onClickDraftSave('#login-link');
});
Sign up using Google
Sign up using Facebook
Sign up using Email and Password
Post as a guest
Required, but never shown
StackExchange.ready(
function () {
StackExchange.openid.initPostLogin('.new-post-login', 'https%3a%2f%2fmath.stackexchange.com%2fquestions%2f3002808%2flarge-and-ill-conditioned-quadratic-convex-problem%23new-answer', 'question_page');
}
);
Post as a guest
Required, but never shown
Sign up or log in
StackExchange.ready(function () {
StackExchange.helpers.onClickDraftSave('#login-link');
});
Sign up using Google
Sign up using Facebook
Sign up using Email and Password
Post as a guest
Required, but never shown
Sign up or log in
StackExchange.ready(function () {
StackExchange.helpers.onClickDraftSave('#login-link');
});
Sign up using Google
Sign up using Facebook
Sign up using Email and Password
Post as a guest
Required, but never shown
Sign up or log in
StackExchange.ready(function () {
StackExchange.helpers.onClickDraftSave('#login-link');
});
Sign up using Google
Sign up using Facebook
Sign up using Email and Password
Sign up using Google
Sign up using Facebook
Sign up using Email and Password
Post as a guest
Required, but never shown
Required, but never shown
Required, but never shown
Required, but never shown
Required, but never shown
Required, but never shown
Required, but never shown
Required, but never shown
Required, but never shown
E1J zbJs bC29yo6C,j53DKOCG,SAM3iYnhTHdzymTLGpX4iQrA8798LJhfK6Y9,GSR8,dWnDQ6Le ejrZZHRFYv14h0CIQM7iTx