How to make Spacy's statistical models faster
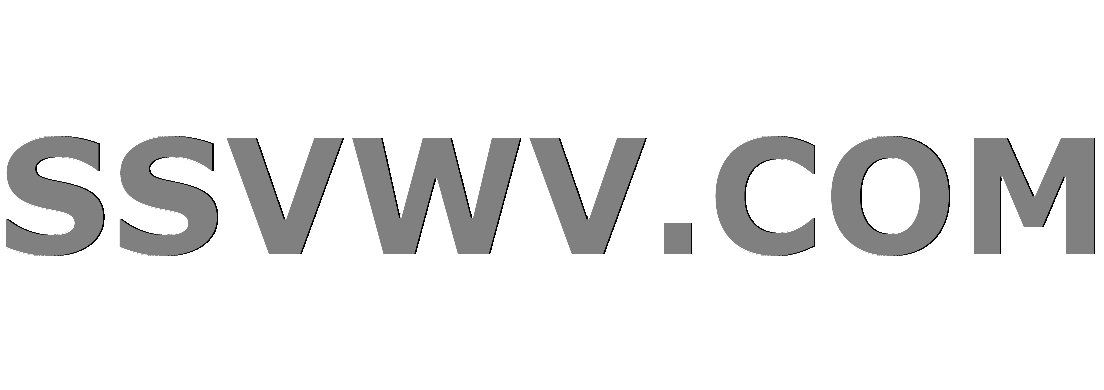
Multi tool use
I am using Spacy's pretrained statistical models such as en_core_web_md. I am trying to find similar words between two lists. While the code is working fine. It takes a lot of time to load the statistical model, each time the code is run.
Here is the code I am using.
How to make the models load faster?
Is there a way to save the model to the disk ?
import spacy
nlp = spacy.load('en_core_web_md')
list1 =['mango','apple','tomato','orange','papaya']
list2 =['mango','fig','cherry','apple','dates']
s_words =
for token1 in list1:
list_to_sort =
for token2 in list2:
list_to_sort.append((token1, token2, nlp(str(token1)).similarity(nlp(str(token2)))))
sorted_list = sorted(list_to_sort, key = itemgetter(2), reverse=True)[0][:2]
s_words.append(sorted_list)
similar_words= list(zip(*s_words))[1]
python-3.x nlp spacy
add a comment |
I am using Spacy's pretrained statistical models such as en_core_web_md. I am trying to find similar words between two lists. While the code is working fine. It takes a lot of time to load the statistical model, each time the code is run.
Here is the code I am using.
How to make the models load faster?
Is there a way to save the model to the disk ?
import spacy
nlp = spacy.load('en_core_web_md')
list1 =['mango','apple','tomato','orange','papaya']
list2 =['mango','fig','cherry','apple','dates']
s_words =
for token1 in list1:
list_to_sort =
for token2 in list2:
list_to_sort.append((token1, token2, nlp(str(token1)).similarity(nlp(str(token2)))))
sorted_list = sorted(list_to_sort, key = itemgetter(2), reverse=True)[0][:2]
s_words.append(sorted_list)
similar_words= list(zip(*s_words))[1]
python-3.x nlp spacy
1
Model loading in IO bound. If you want it to go faster load smaller models. You are usingweb_md
, which stands for medium- there is alsoen_core_web_sm
– mbatchkarov
Nov 19 '18 at 19:43
@mbatchkarov Thanks for your answer. But I think I do require medium models at the least. I also tried disable =['parser','ner','tagger']. It definitely speeds up the loading but what if In some case, the user does require parser, ner etc. The model loading by default has to be faster in such a case. I believe disabling is just a hack. Wonder is there a better solution. This was the gist of my question above.
– venkatttaknev
Nov 19 '18 at 20:15
A spacy model is a large and complex data structure. To make deserialisation faster you need to attack either the large part (by finding a smaller model) or by the complex part (by rewriting spacy or training your own models). Outside of these two options, we'd have to rethink the fundamentals of what you are doing. Here are a few questions. Do you have evidence to suggest you absolutely require at least medium model? Can you compromise on accuracy? Can you preload the models once and query them repeatedly (e.g. a web service/ a jupyter notebook cell)? Do you have a fast SSD?
– mbatchkarov
Nov 20 '18 at 9:05
add a comment |
I am using Spacy's pretrained statistical models such as en_core_web_md. I am trying to find similar words between two lists. While the code is working fine. It takes a lot of time to load the statistical model, each time the code is run.
Here is the code I am using.
How to make the models load faster?
Is there a way to save the model to the disk ?
import spacy
nlp = spacy.load('en_core_web_md')
list1 =['mango','apple','tomato','orange','papaya']
list2 =['mango','fig','cherry','apple','dates']
s_words =
for token1 in list1:
list_to_sort =
for token2 in list2:
list_to_sort.append((token1, token2, nlp(str(token1)).similarity(nlp(str(token2)))))
sorted_list = sorted(list_to_sort, key = itemgetter(2), reverse=True)[0][:2]
s_words.append(sorted_list)
similar_words= list(zip(*s_words))[1]
python-3.x nlp spacy
I am using Spacy's pretrained statistical models such as en_core_web_md. I am trying to find similar words between two lists. While the code is working fine. It takes a lot of time to load the statistical model, each time the code is run.
Here is the code I am using.
How to make the models load faster?
Is there a way to save the model to the disk ?
import spacy
nlp = spacy.load('en_core_web_md')
list1 =['mango','apple','tomato','orange','papaya']
list2 =['mango','fig','cherry','apple','dates']
s_words =
for token1 in list1:
list_to_sort =
for token2 in list2:
list_to_sort.append((token1, token2, nlp(str(token1)).similarity(nlp(str(token2)))))
sorted_list = sorted(list_to_sort, key = itemgetter(2), reverse=True)[0][:2]
s_words.append(sorted_list)
similar_words= list(zip(*s_words))[1]
python-3.x nlp spacy
python-3.x nlp spacy
asked Nov 19 '18 at 12:40


venkatttaknevvenkatttaknev
498
498
1
Model loading in IO bound. If you want it to go faster load smaller models. You are usingweb_md
, which stands for medium- there is alsoen_core_web_sm
– mbatchkarov
Nov 19 '18 at 19:43
@mbatchkarov Thanks for your answer. But I think I do require medium models at the least. I also tried disable =['parser','ner','tagger']. It definitely speeds up the loading but what if In some case, the user does require parser, ner etc. The model loading by default has to be faster in such a case. I believe disabling is just a hack. Wonder is there a better solution. This was the gist of my question above.
– venkatttaknev
Nov 19 '18 at 20:15
A spacy model is a large and complex data structure. To make deserialisation faster you need to attack either the large part (by finding a smaller model) or by the complex part (by rewriting spacy or training your own models). Outside of these two options, we'd have to rethink the fundamentals of what you are doing. Here are a few questions. Do you have evidence to suggest you absolutely require at least medium model? Can you compromise on accuracy? Can you preload the models once and query them repeatedly (e.g. a web service/ a jupyter notebook cell)? Do you have a fast SSD?
– mbatchkarov
Nov 20 '18 at 9:05
add a comment |
1
Model loading in IO bound. If you want it to go faster load smaller models. You are usingweb_md
, which stands for medium- there is alsoen_core_web_sm
– mbatchkarov
Nov 19 '18 at 19:43
@mbatchkarov Thanks for your answer. But I think I do require medium models at the least. I also tried disable =['parser','ner','tagger']. It definitely speeds up the loading but what if In some case, the user does require parser, ner etc. The model loading by default has to be faster in such a case. I believe disabling is just a hack. Wonder is there a better solution. This was the gist of my question above.
– venkatttaknev
Nov 19 '18 at 20:15
A spacy model is a large and complex data structure. To make deserialisation faster you need to attack either the large part (by finding a smaller model) or by the complex part (by rewriting spacy or training your own models). Outside of these two options, we'd have to rethink the fundamentals of what you are doing. Here are a few questions. Do you have evidence to suggest you absolutely require at least medium model? Can you compromise on accuracy? Can you preload the models once and query them repeatedly (e.g. a web service/ a jupyter notebook cell)? Do you have a fast SSD?
– mbatchkarov
Nov 20 '18 at 9:05
1
1
Model loading in IO bound. If you want it to go faster load smaller models. You are using
web_md
, which stands for medium- there is also en_core_web_sm
– mbatchkarov
Nov 19 '18 at 19:43
Model loading in IO bound. If you want it to go faster load smaller models. You are using
web_md
, which stands for medium- there is also en_core_web_sm
– mbatchkarov
Nov 19 '18 at 19:43
@mbatchkarov Thanks for your answer. But I think I do require medium models at the least. I also tried disable =['parser','ner','tagger']. It definitely speeds up the loading but what if In some case, the user does require parser, ner etc. The model loading by default has to be faster in such a case. I believe disabling is just a hack. Wonder is there a better solution. This was the gist of my question above.
– venkatttaknev
Nov 19 '18 at 20:15
@mbatchkarov Thanks for your answer. But I think I do require medium models at the least. I also tried disable =['parser','ner','tagger']. It definitely speeds up the loading but what if In some case, the user does require parser, ner etc. The model loading by default has to be faster in such a case. I believe disabling is just a hack. Wonder is there a better solution. This was the gist of my question above.
– venkatttaknev
Nov 19 '18 at 20:15
A spacy model is a large and complex data structure. To make deserialisation faster you need to attack either the large part (by finding a smaller model) or by the complex part (by rewriting spacy or training your own models). Outside of these two options, we'd have to rethink the fundamentals of what you are doing. Here are a few questions. Do you have evidence to suggest you absolutely require at least medium model? Can you compromise on accuracy? Can you preload the models once and query them repeatedly (e.g. a web service/ a jupyter notebook cell)? Do you have a fast SSD?
– mbatchkarov
Nov 20 '18 at 9:05
A spacy model is a large and complex data structure. To make deserialisation faster you need to attack either the large part (by finding a smaller model) or by the complex part (by rewriting spacy or training your own models). Outside of these two options, we'd have to rethink the fundamentals of what you are doing. Here are a few questions. Do you have evidence to suggest you absolutely require at least medium model? Can you compromise on accuracy? Can you preload the models once and query them repeatedly (e.g. a web service/ a jupyter notebook cell)? Do you have a fast SSD?
– mbatchkarov
Nov 20 '18 at 9:05
add a comment |
0
active
oldest
votes
Your Answer
StackExchange.ifUsing("editor", function () {
StackExchange.using("externalEditor", function () {
StackExchange.using("snippets", function () {
StackExchange.snippets.init();
});
});
}, "code-snippets");
StackExchange.ready(function() {
var channelOptions = {
tags: "".split(" "),
id: "1"
};
initTagRenderer("".split(" "), "".split(" "), channelOptions);
StackExchange.using("externalEditor", function() {
// Have to fire editor after snippets, if snippets enabled
if (StackExchange.settings.snippets.snippetsEnabled) {
StackExchange.using("snippets", function() {
createEditor();
});
}
else {
createEditor();
}
});
function createEditor() {
StackExchange.prepareEditor({
heartbeatType: 'answer',
autoActivateHeartbeat: false,
convertImagesToLinks: true,
noModals: true,
showLowRepImageUploadWarning: true,
reputationToPostImages: 10,
bindNavPrevention: true,
postfix: "",
imageUploader: {
brandingHtml: "Powered by u003ca class="icon-imgur-white" href="https://imgur.com/"u003eu003c/au003e",
contentPolicyHtml: "User contributions licensed under u003ca href="https://creativecommons.org/licenses/by-sa/3.0/"u003ecc by-sa 3.0 with attribution requiredu003c/au003e u003ca href="https://stackoverflow.com/legal/content-policy"u003e(content policy)u003c/au003e",
allowUrls: true
},
onDemand: true,
discardSelector: ".discard-answer"
,immediatelyShowMarkdownHelp:true
});
}
});
Sign up or log in
StackExchange.ready(function () {
StackExchange.helpers.onClickDraftSave('#login-link');
});
Sign up using Google
Sign up using Facebook
Sign up using Email and Password
Post as a guest
Required, but never shown
StackExchange.ready(
function () {
StackExchange.openid.initPostLogin('.new-post-login', 'https%3a%2f%2fstackoverflow.com%2fquestions%2f53374876%2fhow-to-make-spacys-statistical-models-faster%23new-answer', 'question_page');
}
);
Post as a guest
Required, but never shown
0
active
oldest
votes
0
active
oldest
votes
active
oldest
votes
active
oldest
votes
Thanks for contributing an answer to Stack Overflow!
- Please be sure to answer the question. Provide details and share your research!
But avoid …
- Asking for help, clarification, or responding to other answers.
- Making statements based on opinion; back them up with references or personal experience.
To learn more, see our tips on writing great answers.
Sign up or log in
StackExchange.ready(function () {
StackExchange.helpers.onClickDraftSave('#login-link');
});
Sign up using Google
Sign up using Facebook
Sign up using Email and Password
Post as a guest
Required, but never shown
StackExchange.ready(
function () {
StackExchange.openid.initPostLogin('.new-post-login', 'https%3a%2f%2fstackoverflow.com%2fquestions%2f53374876%2fhow-to-make-spacys-statistical-models-faster%23new-answer', 'question_page');
}
);
Post as a guest
Required, but never shown
Sign up or log in
StackExchange.ready(function () {
StackExchange.helpers.onClickDraftSave('#login-link');
});
Sign up using Google
Sign up using Facebook
Sign up using Email and Password
Post as a guest
Required, but never shown
Sign up or log in
StackExchange.ready(function () {
StackExchange.helpers.onClickDraftSave('#login-link');
});
Sign up using Google
Sign up using Facebook
Sign up using Email and Password
Post as a guest
Required, but never shown
Sign up or log in
StackExchange.ready(function () {
StackExchange.helpers.onClickDraftSave('#login-link');
});
Sign up using Google
Sign up using Facebook
Sign up using Email and Password
Sign up using Google
Sign up using Facebook
Sign up using Email and Password
Post as a guest
Required, but never shown
Required, but never shown
Required, but never shown
Required, but never shown
Required, but never shown
Required, but never shown
Required, but never shown
Required, but never shown
Required, but never shown
Lp,HMnqhsyaEXopl,jXETvrQ2GVfGuRyktYTrP8pZr
1
Model loading in IO bound. If you want it to go faster load smaller models. You are using
web_md
, which stands for medium- there is alsoen_core_web_sm
– mbatchkarov
Nov 19 '18 at 19:43
@mbatchkarov Thanks for your answer. But I think I do require medium models at the least. I also tried disable =['parser','ner','tagger']. It definitely speeds up the loading but what if In some case, the user does require parser, ner etc. The model loading by default has to be faster in such a case. I believe disabling is just a hack. Wonder is there a better solution. This was the gist of my question above.
– venkatttaknev
Nov 19 '18 at 20:15
A spacy model is a large and complex data structure. To make deserialisation faster you need to attack either the large part (by finding a smaller model) or by the complex part (by rewriting spacy or training your own models). Outside of these two options, we'd have to rethink the fundamentals of what you are doing. Here are a few questions. Do you have evidence to suggest you absolutely require at least medium model? Can you compromise on accuracy? Can you preload the models once and query them repeatedly (e.g. a web service/ a jupyter notebook cell)? Do you have a fast SSD?
– mbatchkarov
Nov 20 '18 at 9:05